mirror of
https://github.com/hwchase17/langchain.git
synced 2025-05-01 05:15:17 +00:00
Compare commits
241 Commits
langchain-
...
master
Author | SHA1 | Date | |
---|---|---|---|
|
da59eb7eb4 | ||
|
918c950737 | ||
|
ed7cd3c5c4 | ||
|
952a0b7b40 | ||
|
0b8e9868e6 | ||
|
275ba2ec37 | ||
|
bdb7c4a8b3 | ||
|
868f07f8f4 | ||
|
3072e4610a | ||
|
9ff5b5d282 | ||
|
7e926520d5 | ||
|
d614842d23 | ||
|
ff1602f0fd | ||
|
aee7988a94 | ||
|
a2863f8757 | ||
|
3fb0a55122 | ||
|
5fb8fd863a | ||
|
79a537d308 | ||
|
ba2518995d | ||
|
04a899ebe3 | ||
|
a82d987f09 | ||
|
a60fd06784 | ||
|
629b7a5a43 | ||
|
ab871a7b39 | ||
|
d30c56a8c1 | ||
|
09c1991e96 | ||
|
a7903280dd | ||
|
d0f0d1f966 | ||
|
403fae8eec | ||
|
d6b50ad3f6 | ||
|
10a9c24dae | ||
|
8fc7a723b9 | ||
|
f4863f82e2 | ||
|
ae4b6380d9 | ||
|
ffbc64c72a | ||
|
6b0b317cb5 | ||
|
21962e2201 | ||
|
1eb0bdadfa | ||
|
7ecdac5240 | ||
|
faef3e5d50 | ||
|
d4fc734250 | ||
|
4bc70766b5 | ||
|
e4877e5ef1 | ||
|
8c5ae108dd | ||
|
eedda164c6 | ||
|
4be55f7c89 | ||
|
577cb53a00 | ||
|
a7c1bccd6a | ||
|
25d77aa8b4 | ||
|
59fd4cb4c0 | ||
|
b8c454b42b | ||
|
a43df006de | ||
|
0f6fa34372 | ||
|
e8a84b05a4 | ||
|
8574442c57 | ||
|
920d504e47 | ||
|
1f3054502e | ||
|
589bc19890 | ||
|
27296bdb0c | ||
|
0e9d0dbc10 | ||
|
de56c31672 | ||
|
335f089d6a | ||
|
9418c0d8a5 | ||
|
23f701b08e | ||
|
b344f34635 | ||
|
017c8079e1 | ||
|
d0cd115356 | ||
|
34ddfba76b | ||
|
5ffcd01c41 | ||
|
096f0e5966 | ||
|
46de0866db | ||
|
d624a475e4 | ||
|
dbf9986d44 | ||
|
0c723af4b0 | ||
|
f14bcee525 | ||
|
98c357b3d7 | ||
|
d2cbfa379f | ||
|
75e50a3efd | ||
|
61d2dc011e | ||
|
f0f90c4d88 | ||
|
f01b89df56 | ||
|
add6a78f98 | ||
|
2c2db1ab69 | ||
|
86d51f6be6 | ||
|
83b66cb916 | ||
|
ff2930c119 | ||
|
b36c2bf833 | ||
|
9e82f1df4e | ||
|
fa362189a1 | ||
|
88fce67724 | ||
|
60d8ade078 | ||
|
ca39680d2a | ||
|
4af3f89a3a | ||
|
4ff576e37d | ||
|
085baef926 | ||
|
47ded80b64 | ||
|
cf2697ec53 | ||
|
8e9569cbc8 | ||
|
dd5f5902e3 | ||
|
3382ee8f57 | ||
|
ef5aff3b6c | ||
|
a4ca1fe0ed | ||
|
6baf5c05a6 | ||
|
c6a8663afb | ||
|
1f5e207379 | ||
|
7240458619 | ||
|
6aa5494a75 | ||
|
7262de4217 | ||
|
9cfe6bcacd | ||
|
09438857e8 | ||
|
e3b6cddd5e | ||
|
59f2c9e737 | ||
|
ed5c4805f6 | ||
|
2282762528 | ||
|
f7c4965fb6 | ||
|
edb6a23aea | ||
|
3a64c7195f | ||
|
4f69094b51 | ||
|
ada740b5b9 | ||
|
f005988e31 | ||
|
446361a0d3 | ||
|
afd457d8e1 | ||
|
42944f3499 | ||
|
bb2c2fd885 | ||
|
913c896598 | ||
|
2803a48661 | ||
|
fdc2b4bcac | ||
|
48affc498b | ||
|
d9b628e764 | ||
|
9cfb95e621 | ||
|
89f28a24d3 | ||
|
8c6734325b | ||
|
e72f3c26a0 | ||
|
f3c3ec9aec | ||
|
dc19d42d37 | ||
|
68d16d8a07 | ||
|
5103594a2c | ||
|
e42b3d285a | ||
|
48cf7c838d | ||
|
b6fe7e8c10 | ||
|
7a4ae6fbff | ||
|
8e053ac9d2 | ||
|
e981a9810d | ||
|
70532a65f8 | ||
|
c6172d167a | ||
|
f70df01e01 | ||
|
8f8fea2d7e | ||
|
cd6a83117c | ||
|
6c45c9efc3 | ||
|
44b83460b2 | ||
|
c87a270e5f | ||
|
63c16f5ca8 | ||
|
4cc7bc6c93 | ||
|
68361f9c2d | ||
|
98f0016fc2 | ||
|
66758599a9 | ||
|
d47d6ecbc3 | ||
|
78ec7d886d | ||
|
5fb261ce27 | ||
|
636d831d27 | ||
|
deec538335 | ||
|
164e606cae | ||
|
5686fed40b | ||
|
4556b81b1d | ||
|
163730aef4 | ||
|
9cbe91896e | ||
|
893942651b | ||
|
3ce0587199 | ||
|
a2bec5f2e5 | ||
|
e3f15f0a47 | ||
|
e106e9602f | ||
|
4f9f97bd12 | ||
|
e935da0b12 | ||
|
4d03ba4686 | ||
|
30af9b8166 | ||
|
2712ecffeb | ||
|
a3671ceb71 | ||
|
6650b94627 | ||
|
d8e3b7667f | ||
|
f0159c7125 | ||
|
2491237473 | ||
|
7c2468f36b | ||
|
bff56c5fa6 | ||
|
150ac0cb79 | ||
|
5e418c2666 | ||
|
43b5dc7191 | ||
|
a007c57285 | ||
|
33ed7c31da | ||
|
f9bb5ec5d0 | ||
|
f79473b752 | ||
|
87e82fe1e8 | ||
|
4e7a9a7014 | ||
|
aa37893c00 | ||
|
1cdea6ab07 | ||
|
901dffe06b | ||
|
0c2c8c36c1 | ||
|
59d508a2ee | ||
|
c235328b39 | ||
|
d0f154dbaa | ||
|
32cd70d7d2 | ||
|
18cf457eec | ||
|
9c03cd5775 | ||
|
af66ab098e | ||
|
b8929e3d5f | ||
|
374769e8fe | ||
|
17a9cd61e9 | ||
|
3814bd1ea7 | ||
|
87c02a1aff | ||
|
884125e129 | ||
|
01d0cfe450 | ||
|
f241fd5c11 | ||
|
9ae792f56c | ||
|
ccc3d32ec8 | ||
|
fe0fd9dd70 | ||
|
38807871ec | ||
|
816492e1d3 | ||
|
111dd90a46 | ||
|
32f7695809 | ||
|
9d3262c7aa | ||
|
8a69de5c24 | ||
|
558191198f | ||
|
4f8ea13cea | ||
|
8a33402016 | ||
|
6896c863e8 | ||
|
768e4f695a | ||
|
88b4233fa1 | ||
|
64df60e690 | ||
|
fdda1aaea1 | ||
|
26a3256fc6 | ||
|
8c8bca68b2 | ||
|
4bbc249b13 | ||
|
ecff055096 | ||
|
0c623045b5 | ||
|
e8be3cca5c | ||
|
4419340039 | ||
|
64f97e707e | ||
|
8395abbb42 | ||
|
b9e19c5f97 | ||
|
f4d1df1b2d | ||
|
026de908eb | ||
|
e4515f308f |
.github
.gitignore.pre-commit-config.yamlREADME.mdcookbook
docs
api_reference
cassettes
multimodal_inputs_0f68cce7-350b-4cde-bc40-d3a169551fc3.msgpack.zlibmultimodal_inputs_1fcf7b27-1cc3-420a-b920-0420b5892e20.msgpack.zlibmultimodal_inputs_325fb4ca.msgpack.zlibmultimodal_inputs_55e1d937-3b22-4deb-b9f0-9e688f0609dc.msgpack.zlibmultimodal_inputs_6c1455a9-699a-4702-a7e0-7f6eaec76a21.msgpack.zlibmultimodal_inputs_83593b9d-a8d3-4c99-9dac-64e0a9d397cb.msgpack.zlibmultimodal_inputs_99d27f8f-ae78-48bc-9bf2-3cef35213ec7.msgpack.zlibmultimodal_inputs_9bbf578e-794a-4dc0-a469-78c876ccd4a3.msgpack.zlibmultimodal_inputs_9ca1040c.msgpack.zlibmultimodal_inputs_a0b91b29-dbd6-4c94-8f24-05471adc7598.msgpack.zlibmultimodal_inputs_a8819cf3-5ddc-44f0-889a-19ca7b7fe77e.msgpack.zlibmultimodal_inputs_ae076c9b-ff8f-461d-9349-250f396c9a25.msgpack.zlibmultimodal_inputs_cd22ea82-2f93-46f9-9f7a-6aaf479fcaa9.msgpack.zlibmultimodal_inputs_ea7707a1-5660-40a1-a10f-0df48a028689.msgpack.zlibmultimodal_inputs_ec680b6b.msgpack.zlibsql_qa_11.msgpack.zlibsql_qa_15.msgpack.zlibsql_qa_25.msgpack.zlibsql_qa_27.msgpack.zlibsql_qa_31.msgpack.zlibsql_qa_33.msgpack.zlibsql_qa_39.msgpack.zlibsql_qa_45.msgpack.zlibsql_qa_47.msgpack.zlibsql_qa_55.msgpack.zlibsql_qa_57.msgpack.zlibsql_qa_60.msgpack.zlib
docs
changes/changelog
concepts
contributing/how_to
how_to
integrations
caches
chat
bedrock.ipynbcloudflare_workersai.ipynbgoogle_generative_ai.ipynblitellm.ipynblitellm_router.ipynbnaver.ipynbollama.ipynbopenai.ipynbperplexity.ipynbpredictionguard.ipynbqwq.ipynbrunpod.ipynbseekrflow.ipynb
document_loaders
document_transformers
llm_caching.ipynbllms
memory
providers
4
.github/ISSUE_TEMPLATE/bug-report.yml
vendored
4
.github/ISSUE_TEMPLATE/bug-report.yml
vendored
@ -29,14 +29,14 @@ body:
|
||||
options:
|
||||
- label: I added a very descriptive title to this issue.
|
||||
required: true
|
||||
- label: I searched the LangChain documentation with the integrated search.
|
||||
required: true
|
||||
- label: I used the GitHub search to find a similar question and didn't find it.
|
||||
required: true
|
||||
- label: I am sure that this is a bug in LangChain rather than my code.
|
||||
required: true
|
||||
- label: The bug is not resolved by updating to the latest stable version of LangChain (or the specific integration package).
|
||||
required: true
|
||||
- label: I posted a self-contained, minimal, reproducible example. A maintainer can copy it and run it AS IS.
|
||||
required: true
|
||||
- type: textarea
|
||||
id: reproduction
|
||||
validations:
|
||||
|
5
.github/PULL_REQUEST_TEMPLATE.md
vendored
5
.github/PULL_REQUEST_TEMPLATE.md
vendored
@ -1,8 +1,8 @@
|
||||
Thank you for contributing to LangChain!
|
||||
|
||||
- [ ] **PR title**: "package: description"
|
||||
- Where "package" is whichever of langchain, community, core, etc. is being modified. Use "docs: ..." for purely docs changes, "infra: ..." for CI changes.
|
||||
- Example: "community: add foobar LLM"
|
||||
- Where "package" is whichever of langchain, core, etc. is being modified. Use "docs: ..." for purely docs changes, "infra: ..." for CI changes.
|
||||
- Example: "core: add foobar LLM"
|
||||
|
||||
|
||||
- [ ] **PR message**: ***Delete this entire checklist*** and replace with
|
||||
@ -24,6 +24,5 @@ Additional guidelines:
|
||||
- Please do not add dependencies to pyproject.toml files (even optional ones) unless they are required for unit tests.
|
||||
- Most PRs should not touch more than one package.
|
||||
- Changes should be backwards compatible.
|
||||
- If you are adding something to community, do not re-import it in langchain.
|
||||
|
||||
If no one reviews your PR within a few days, please @-mention one of baskaryan, eyurtsev, ccurme, vbarda, hwchase17.
|
||||
|
15
.github/scripts/check_diff.py
vendored
15
.github/scripts/check_diff.py
vendored
@ -16,7 +16,6 @@ LANGCHAIN_DIRS = [
|
||||
"libs/core",
|
||||
"libs/text-splitters",
|
||||
"libs/langchain",
|
||||
"libs/community",
|
||||
]
|
||||
|
||||
# when set to True, we are ignoring core dependents
|
||||
@ -38,8 +37,8 @@ IGNORED_PARTNERS = [
|
||||
]
|
||||
|
||||
PY_312_MAX_PACKAGES = [
|
||||
"libs/partners/huggingface", # https://github.com/pytorch/pytorch/issues/130249
|
||||
"libs/partners/voyageai",
|
||||
"libs/partners/chroma", # https://github.com/chroma-core/chroma/issues/4382
|
||||
]
|
||||
|
||||
|
||||
@ -134,12 +133,6 @@ def _get_configs_for_single_dir(job: str, dir_: str) -> List[Dict[str, str]]:
|
||||
elif dir_ == "libs/langchain" and job == "extended-tests":
|
||||
py_versions = ["3.9", "3.13"]
|
||||
|
||||
elif dir_ == "libs/community" and job == "extended-tests":
|
||||
py_versions = ["3.9", "3.12"]
|
||||
|
||||
elif dir_ == "libs/community" and job == "compile-integration-tests":
|
||||
# community integration deps are slow in 3.12
|
||||
py_versions = ["3.9", "3.11"]
|
||||
elif dir_ == ".":
|
||||
# unable to install with 3.13 because tokenizers doesn't support 3.13 yet
|
||||
py_versions = ["3.9", "3.12"]
|
||||
@ -184,11 +177,6 @@ def _get_pydantic_test_configs(
|
||||
else "0"
|
||||
)
|
||||
|
||||
custom_mins = {
|
||||
# depends on pydantic-settings 2.4 which requires pydantic 2.7
|
||||
"libs/community": 7,
|
||||
}
|
||||
|
||||
max_pydantic_minor = min(
|
||||
int(dir_max_pydantic_minor),
|
||||
int(core_max_pydantic_minor),
|
||||
@ -196,7 +184,6 @@ def _get_pydantic_test_configs(
|
||||
min_pydantic_minor = max(
|
||||
int(dir_min_pydantic_minor),
|
||||
int(core_min_pydantic_minor),
|
||||
custom_mins.get(dir_, 0),
|
||||
)
|
||||
|
||||
configs = [
|
||||
|
2
.github/scripts/get_min_versions.py
vendored
2
.github/scripts/get_min_versions.py
vendored
@ -22,7 +22,6 @@ import re
|
||||
|
||||
MIN_VERSION_LIBS = [
|
||||
"langchain-core",
|
||||
"langchain-community",
|
||||
"langchain",
|
||||
"langchain-text-splitters",
|
||||
"numpy",
|
||||
@ -35,7 +34,6 @@ SKIP_IF_PULL_REQUEST = [
|
||||
"langchain-core",
|
||||
"langchain-text-splitters",
|
||||
"langchain",
|
||||
"langchain-community",
|
||||
]
|
||||
|
||||
|
||||
|
6
.github/scripts/prep_api_docs_build.py
vendored
6
.github/scripts/prep_api_docs_build.py
vendored
@ -20,6 +20,8 @@ def get_target_dir(package_name: str) -> Path:
|
||||
base_path = Path("langchain/libs")
|
||||
if package_name_short == "experimental":
|
||||
return base_path / "experimental"
|
||||
if package_name_short == "community":
|
||||
return base_path / "community"
|
||||
return base_path / "partners" / package_name_short
|
||||
|
||||
|
||||
@ -69,7 +71,7 @@ def main():
|
||||
clean_target_directories([
|
||||
p
|
||||
for p in package_yaml["packages"]
|
||||
if p["repo"].startswith("langchain-ai/")
|
||||
if (p["repo"].startswith("langchain-ai/") or p.get("include_in_api_ref"))
|
||||
and p["repo"] != "langchain-ai/langchain"
|
||||
])
|
||||
|
||||
@ -78,7 +80,7 @@ def main():
|
||||
p
|
||||
for p in package_yaml["packages"]
|
||||
if not p.get("disabled", False)
|
||||
and p["repo"].startswith("langchain-ai/")
|
||||
and (p["repo"].startswith("langchain-ai/") or p.get("include_in_api_ref"))
|
||||
and p["repo"] != "langchain-ai/langchain"
|
||||
])
|
||||
|
||||
|
1
.github/workflows/.codespell-exclude
vendored
1
.github/workflows/.codespell-exclude
vendored
@ -1,4 +1,3 @@
|
||||
libs/community/langchain_community/llms/yuan2.py
|
||||
"NotIn": "not in",
|
||||
- `/checkin`: Check-in
|
||||
docs/docs/integrations/providers/trulens.mdx
|
||||
|
6
.github/workflows/_integration_test.yml
vendored
6
.github/workflows/_integration_test.yml
vendored
@ -34,11 +34,6 @@ jobs:
|
||||
shell: bash
|
||||
run: uv sync --group test --group test_integration
|
||||
|
||||
- name: Install deps outside pyproject
|
||||
if: ${{ startsWith(inputs.working-directory, 'libs/community/') }}
|
||||
shell: bash
|
||||
run: VIRTUAL_ENV=.venv uv pip install "boto3<2" "google-cloud-aiplatform<2"
|
||||
|
||||
- name: Run integration tests
|
||||
shell: bash
|
||||
env:
|
||||
@ -76,6 +71,7 @@ jobs:
|
||||
COHERE_API_KEY: ${{ secrets.COHERE_API_KEY }}
|
||||
UPSTAGE_API_KEY: ${{ secrets.UPSTAGE_API_KEY }}
|
||||
XAI_API_KEY: ${{ secrets.XAI_API_KEY }}
|
||||
PPLX_API_KEY: ${{ secrets.PPLX_API_KEY }}
|
||||
run: |
|
||||
make integration_tests
|
||||
|
||||
|
8
.github/workflows/_release.yml
vendored
8
.github/workflows/_release.yml
vendored
@ -327,6 +327,7 @@ jobs:
|
||||
FIREWORKS_API_KEY: ${{ secrets.FIREWORKS_API_KEY }}
|
||||
XAI_API_KEY: ${{ secrets.XAI_API_KEY }}
|
||||
DEEPSEEK_API_KEY: ${{ secrets.DEEPSEEK_API_KEY }}
|
||||
PPLX_API_KEY: ${{ secrets.PPLX_API_KEY }}
|
||||
run: make integration_tests
|
||||
working-directory: ${{ inputs.working-directory }}
|
||||
|
||||
@ -394,8 +395,11 @@ jobs:
|
||||
|
||||
# Checkout the latest package files
|
||||
rm -rf $GITHUB_WORKSPACE/libs/partners/${{ matrix.partner }}/*
|
||||
cd $GITHUB_WORKSPACE/libs/partners/${{ matrix.partner }}
|
||||
git checkout "$LATEST_PACKAGE_TAG" -- .
|
||||
rm -rf $GITHUB_WORKSPACE/libs/standard-tests/*
|
||||
cd $GITHUB_WORKSPACE/libs/
|
||||
git checkout "$LATEST_PACKAGE_TAG" -- standard-tests/
|
||||
git checkout "$LATEST_PACKAGE_TAG" -- partners/${{ matrix.partner }}/
|
||||
cd partners/${{ matrix.partner }}
|
||||
|
||||
# Print as a sanity check
|
||||
echo "Version number from pyproject.toml: "
|
||||
|
2
.github/workflows/_test_doc_imports.yml
vendored
2
.github/workflows/_test_doc_imports.yml
vendored
@ -30,7 +30,7 @@ jobs:
|
||||
|
||||
- name: Install langchain editable
|
||||
run: |
|
||||
VIRTUAL_ENV=.venv uv pip install langchain-experimental -e libs/core libs/langchain libs/community
|
||||
VIRTUAL_ENV=.venv uv pip install langchain-experimental langchain-community -e libs/core libs/langchain
|
||||
|
||||
- name: Check doc imports
|
||||
shell: bash
|
||||
|
23
.github/workflows/api_doc_build.yml
vendored
23
.github/workflows/api_doc_build.yml
vendored
@ -26,7 +26,20 @@ jobs:
|
||||
id: get-unsorted-repos
|
||||
uses: mikefarah/yq@master
|
||||
with:
|
||||
cmd: yq '.packages[].repo' langchain/libs/packages.yml
|
||||
cmd: |
|
||||
yq '
|
||||
.packages[]
|
||||
| select(
|
||||
(
|
||||
(.repo | test("^langchain-ai/"))
|
||||
and
|
||||
(.repo != "langchain-ai/langchain")
|
||||
)
|
||||
or
|
||||
(.include_in_api_ref // false)
|
||||
)
|
||||
| .repo
|
||||
' langchain/libs/packages.yml
|
||||
|
||||
- name: Parse YAML and checkout repos
|
||||
env:
|
||||
@ -38,11 +51,9 @@ jobs:
|
||||
|
||||
# Checkout each unique repository that is in langchain-ai org
|
||||
for repo in $REPOS; do
|
||||
if [[ "$repo" != "langchain-ai/langchain" && "$repo" == langchain-ai/* ]]; then
|
||||
REPO_NAME=$(echo $repo | cut -d'/' -f2)
|
||||
echo "Checking out $repo to $REPO_NAME"
|
||||
git clone --depth 1 https://github.com/$repo.git $REPO_NAME
|
||||
fi
|
||||
REPO_NAME=$(echo $repo | cut -d'/' -f2)
|
||||
echo "Checking out $repo to $REPO_NAME"
|
||||
git clone --depth 1 https://github.com/$repo.git $REPO_NAME
|
||||
done
|
||||
|
||||
- name: Setup python ${{ env.PYTHON_VERSION }}
|
||||
|
29
.github/workflows/check_core_versions.yml
vendored
Normal file
29
.github/workflows/check_core_versions.yml
vendored
Normal file
@ -0,0 +1,29 @@
|
||||
name: Check `langchain-core` version equality
|
||||
|
||||
on:
|
||||
pull_request:
|
||||
paths:
|
||||
- 'libs/core/pyproject.toml'
|
||||
- 'libs/core/langchain_core/version.py'
|
||||
|
||||
jobs:
|
||||
check_version_equality:
|
||||
runs-on: ubuntu-latest
|
||||
|
||||
steps:
|
||||
- uses: actions/checkout@v4
|
||||
|
||||
- name: Check version equality
|
||||
run: |
|
||||
PYPROJECT_VERSION=$(grep -Po '(?<=^version = ")[^"]*' libs/core/pyproject.toml)
|
||||
VERSION_PY_VERSION=$(grep -Po '(?<=^VERSION = ")[^"]*' libs/core/langchain_core/version.py)
|
||||
|
||||
# Compare the two versions
|
||||
if [ "$PYPROJECT_VERSION" != "$VERSION_PY_VERSION" ]; then
|
||||
echo "langchain-core versions in pyproject.toml and version.py do not match!"
|
||||
echo "pyproject.toml version: $PYPROJECT_VERSION"
|
||||
echo "version.py version: $VERSION_PY_VERSION"
|
||||
exit 1
|
||||
else
|
||||
echo "Versions match: $PYPROJECT_VERSION"
|
||||
fi
|
44
.github/workflows/codspeed.yml
vendored
Normal file
44
.github/workflows/codspeed.yml
vendored
Normal file
@ -0,0 +1,44 @@
|
||||
name: CodSpeed
|
||||
|
||||
on:
|
||||
push:
|
||||
branches:
|
||||
- master
|
||||
pull_request:
|
||||
paths:
|
||||
- 'libs/core/**'
|
||||
# `workflow_dispatch` allows CodSpeed to trigger backtest
|
||||
# performance analysis in order to generate initial data.
|
||||
workflow_dispatch:
|
||||
|
||||
jobs:
|
||||
codspeed:
|
||||
name: Run benchmarks
|
||||
if: (github.event_name == 'pull_request' && contains(github.event.pull_request.labels.*.name, 'run-codspeed-benchmarks')) || github.event_name == 'workflow_dispatch' || github.event_name == 'push'
|
||||
runs-on: ubuntu-latest
|
||||
steps:
|
||||
- uses: actions/checkout@v4
|
||||
|
||||
# We have to use 3.12, 3.13 is not yet supported
|
||||
- name: Install uv
|
||||
uses: astral-sh/setup-uv@v5
|
||||
with:
|
||||
python-version: "3.12"
|
||||
|
||||
# Using this action is still necessary for CodSpeed to work
|
||||
- uses: actions/setup-python@v3
|
||||
with:
|
||||
python-version: "3.12"
|
||||
|
||||
- name: install deps
|
||||
run: uv sync --group test
|
||||
working-directory: ./libs/core
|
||||
|
||||
- name: Run benchmarks
|
||||
uses: CodSpeedHQ/action@v3
|
||||
with:
|
||||
token: ${{ secrets.CODSPEED_TOKEN }}
|
||||
run: |
|
||||
cd libs/core
|
||||
uv run --no-sync pytest ./tests/benchmarks --codspeed
|
||||
mode: walltime
|
11
.github/workflows/people.yml
vendored
11
.github/workflows/people.yml
vendored
@ -6,11 +6,6 @@ on:
|
||||
push:
|
||||
branches: [jacob/people]
|
||||
workflow_dispatch:
|
||||
inputs:
|
||||
debug_enabled:
|
||||
description: 'Run the build with tmate debugging enabled (https://github.com/marketplace/actions/debugging-with-tmate)'
|
||||
required: false
|
||||
default: 'false'
|
||||
|
||||
jobs:
|
||||
langchain-people:
|
||||
@ -26,12 +21,6 @@ jobs:
|
||||
# Ref: https://github.com/actions/runner/issues/2033
|
||||
- name: Fix git safe.directory in container
|
||||
run: mkdir -p /home/runner/work/_temp/_github_home && printf "[safe]\n\tdirectory = /github/workspace" > /home/runner/work/_temp/_github_home/.gitconfig
|
||||
# Allow debugging with tmate
|
||||
- name: Setup tmate session
|
||||
uses: mxschmitt/action-tmate@v3
|
||||
if: ${{ github.event_name == 'workflow_dispatch' && github.event.inputs.debug_enabled == 'true' }}
|
||||
with:
|
||||
limit-access-to-actor: true
|
||||
- uses: ./.github/actions/people
|
||||
with:
|
||||
token: ${{ secrets.LANGCHAIN_PEOPLE_GITHUB_TOKEN }}
|
1
.github/workflows/run_notebooks.yml
vendored
1
.github/workflows/run_notebooks.yml
vendored
@ -61,6 +61,7 @@ jobs:
|
||||
env:
|
||||
ANTHROPIC_API_KEY: ${{ secrets.ANTHROPIC_API_KEY }}
|
||||
FIREWORKS_API_KEY: ${{ secrets.FIREWORKS_API_KEY }}
|
||||
GOOGLE_API_KEY: ${{ secrets.GOOGLE_API_KEY }}
|
||||
GROQ_API_KEY: ${{ secrets.GROQ_API_KEY }}
|
||||
MISTRAL_API_KEY: ${{ secrets.MISTRAL_API_KEY }}
|
||||
OPENAI_API_KEY: ${{ secrets.OPENAI_API_KEY }}
|
||||
|
1
.github/workflows/scheduled_test.yml
vendored
1
.github/workflows/scheduled_test.yml
vendored
@ -145,6 +145,7 @@ jobs:
|
||||
GOOGLE_API_KEY: ${{ secrets.GOOGLE_API_KEY }}
|
||||
GOOGLE_SEARCH_API_KEY: ${{ secrets.GOOGLE_SEARCH_API_KEY }}
|
||||
GOOGLE_CSE_ID: ${{ secrets.GOOGLE_CSE_ID }}
|
||||
PPLX_API_KEY: ${{ secrets.PPLX_API_KEY }}
|
||||
run: |
|
||||
cd langchain/${{ matrix.working-directory }}
|
||||
make integration_tests
|
||||
|
1
.gitignore
vendored
1
.gitignore
vendored
@ -59,6 +59,7 @@ coverage.xml
|
||||
*.py,cover
|
||||
.hypothesis/
|
||||
.pytest_cache/
|
||||
.codspeed/
|
||||
|
||||
# Translations
|
||||
*.mo
|
||||
|
@ -7,12 +7,6 @@ repos:
|
||||
entry: make -C libs/core format
|
||||
files: ^libs/core/
|
||||
pass_filenames: false
|
||||
- id: community
|
||||
name: format community
|
||||
language: system
|
||||
entry: make -C libs/community format
|
||||
files: ^libs/community/
|
||||
pass_filenames: false
|
||||
- id: langchain
|
||||
name: format langchain
|
||||
language: system
|
||||
|
@ -15,8 +15,9 @@
|
||||
[](https://star-history.com/#langchain-ai/langchain)
|
||||
[](https://github.com/langchain-ai/langchain/issues)
|
||||
[](https://vscode.dev/redirect?url=vscode://ms-vscode-remote.remote-containers/cloneInVolume?url=https://github.com/langchain-ai/langchain)
|
||||
[](https://codespaces.new/langchain-ai/langchain)
|
||||
[<img src="https://github.com/codespaces/badge.svg" title="Open in Github Codespace" width="150" height="20">](https://codespaces.new/langchain-ai/langchain)
|
||||
[](https://twitter.com/langchainai)
|
||||
[](https://codspeed.io/langchain-ai/langchain)
|
||||
|
||||
> [!NOTE]
|
||||
> Looking for the JS/TS library? Check out [LangChain.js](https://github.com/langchain-ai/langchainjs).
|
||||
|
@ -30,7 +30,7 @@
|
||||
"outputs": [],
|
||||
"source": [
|
||||
"# lock to 0.10.19 due to a persistent bug in more recent versions\n",
|
||||
"! pip install \"unstructured[all-docs]==0.10.19\" pillow pydantic lxml pillow matplotlib tiktoken open_clip_torch torch"
|
||||
"! pip install \"unstructured[all-docs]==0.10.19\" pillow pydantic lxml matplotlib tiktoken open_clip_torch torch"
|
||||
]
|
||||
},
|
||||
{
|
||||
@ -409,7 +409,7 @@
|
||||
" table_summaries,\n",
|
||||
" tables,\n",
|
||||
" image_summaries,\n",
|
||||
" image_summaries,\n",
|
||||
" img_base64_list,\n",
|
||||
")"
|
||||
]
|
||||
},
|
||||
|
@ -11,6 +11,7 @@
|
||||
import json
|
||||
import os
|
||||
import sys
|
||||
from datetime import datetime
|
||||
from pathlib import Path
|
||||
|
||||
import toml
|
||||
@ -104,7 +105,7 @@ def skip_private_members(app, what, name, obj, skip, options):
|
||||
# -- Project information -----------------------------------------------------
|
||||
|
||||
project = "🦜🔗 LangChain"
|
||||
copyright = "2023, LangChain Inc"
|
||||
copyright = f"{datetime.now().year}, LangChain Inc"
|
||||
author = "LangChain, Inc"
|
||||
|
||||
html_favicon = "_static/img/brand/favicon.png"
|
||||
@ -275,3 +276,7 @@ if os.environ.get("READTHEDOCS", "") == "True":
|
||||
html_context["READTHEDOCS"] = True
|
||||
|
||||
master_doc = "index"
|
||||
|
||||
# If a signature’s length in characters exceeds 60,
|
||||
# each parameter within the signature will be displayed on an individual logical line
|
||||
maximum_signature_line_length = 60
|
||||
|
@ -7,7 +7,7 @@
|
||||
|
||||
.. NOTE:: {{objname}} implements the standard :py:class:`Runnable Interface <langchain_core.runnables.base.Runnable>`. 🏃
|
||||
|
||||
The :py:class:`Runnable Interface <langchain_core.runnables.base.Runnable>` has additional methods that are available on runnables, such as :py:meth:`with_types <langchain_core.runnables.base.Runnable.with_types>`, :py:meth:`with_retry <langchain_core.runnables.base.Runnable.with_retry>`, :py:meth:`assign <langchain_core.runnables.base.Runnable.assign>`, :py:meth:`bind <langchain_core.runnables.base.Runnable.bind>`, :py:meth:`get_graph <langchain_core.runnables.base.Runnable.get_graph>`, and more.
|
||||
The :py:class:`Runnable Interface <langchain_core.runnables.base.Runnable>` has additional methods that are available on runnables, such as :py:meth:`with_config <langchain_core.runnables.base.Runnable.with_config>`, :py:meth:`with_types <langchain_core.runnables.base.Runnable.with_types>`, :py:meth:`with_retry <langchain_core.runnables.base.Runnable.with_retry>`, :py:meth:`assign <langchain_core.runnables.base.Runnable.assign>`, :py:meth:`bind <langchain_core.runnables.base.Runnable.bind>`, :py:meth:`get_graph <langchain_core.runnables.base.Runnable.get_graph>`, and more.
|
||||
|
||||
{% block attributes %}
|
||||
{% if attributes %}
|
||||
|
@ -19,6 +19,6 @@
|
||||
|
||||
.. NOTE:: {{objname}} implements the standard :py:class:`Runnable Interface <langchain_core.runnables.base.Runnable>`. 🏃
|
||||
|
||||
The :py:class:`Runnable Interface <langchain_core.runnables.base.Runnable>` has additional methods that are available on runnables, such as :py:meth:`with_types <langchain_core.runnables.base.Runnable.with_types>`, :py:meth:`with_retry <langchain_core.runnables.base.Runnable.with_retry>`, :py:meth:`assign <langchain_core.runnables.base.Runnable.assign>`, :py:meth:`bind <langchain_core.runnables.base.Runnable.bind>`, :py:meth:`get_graph <langchain_core.runnables.base.Runnable.get_graph>`, and more.
|
||||
The :py:class:`Runnable Interface <langchain_core.runnables.base.Runnable>` has additional methods that are available on runnables, such as :py:meth:`with_config <langchain_core.runnables.base.Runnable.with_config>`, :py:meth:`with_types <langchain_core.runnables.base.Runnable.with_types>`, :py:meth:`with_retry <langchain_core.runnables.base.Runnable.with_retry>`, :py:meth:`assign <langchain_core.runnables.base.Runnable.assign>`, :py:meth:`bind <langchain_core.runnables.base.Runnable.bind>`, :py:meth:`get_graph <langchain_core.runnables.base.Runnable.get_graph>`, and more.
|
||||
|
||||
.. example_links:: {{ objname }}
|
||||
|
@ -0,0 +1 @@
|
||||
eNqVVnlwE9cZtzkSh1JKmmYgmTGoGwYS4pV2dUuOGx/CxgRbxhb4Koin3Sdppb28h2TZ9UyBkskE0mYHSBNKpg22ZXANBkw54zQUCMz0dtzO2FCTpslASupp66QZmkD6Vge2Y//THY32Pb3v+H3f+33fp+09MSjJjMDn9jG8AiVAKWgja9t7JNiiQln5QZKDSligu2q8db5OVWJGVocVRZTdJhMQGaMgQh4wRkrgTDHSRIWBYkJrkYUpM10BgU6MzilsxzgoyyAEZczd3I5RAnLFK6m1khAh5sYU2KpgBemXG/NAmZKYADQoYWiIQ4BekoHh0ZaRDQyHDLmxjoL7yqlf/KrEIguTa3c7lnphWbyqyAqANsaZKMNBmgFGQQqZ9J2o73TcHMJsUsIqFzDRJpo2VQRFPM7ICK/M8DgHaEYWeByhwXmgqBLEAwKQ6Dhgo8aIGDKZbXZCbMX/Py2so2NzASYJrB6JKkMJ0/ecQEMde0hUcKuRRIHJigQBh7mDgJUhypQgsPLUDAZVPnV3SPT+EqWAB5x+mkmiX1dDEnQqwWJaaNZ0IyERSEgZkULWDYkSumtJYWBql5VCS8irCFYzJqs8n0BqFCuotL6QAIN+QMFkEKIIGF6PFx0hcjESpHW9rKlJQSEQgZSCBDs2d/SEIaARhLGcxV1hQVa0o9Np1w8oCqIkQZ4SaGReOxJqY8QCAw2DLFBgL7oFHqayofVGIRRxwDIxmExraceAKLIMBfRzUwRdU1+Gm7gOZeZxr35BOGIYr2gnvQhESaWpJoHqgzeQRgdhJI614rKC4mYR33EWIDxJMXV+fuqBCKgoMoJnak9LppWPTpURZK27ClDeumkmgUSFtW4gcXbrwNTfJZVXEKu1nrKame4yh/fd9ViMJIk+x6dZlhM8pXWn6HV6mjZUpAROCciI9gaRpAQhykBtNPdBv58K+gNcUZ3fEvNbAhFPzMFyld6AnWcb1Von79vokNpCzFpbiTGyaf26al+8CicdVqvLaXcRZpw0EkaEArdXcrF4bcOGhMjzmzzlDRTXsq6lPFASLfFz3tKm0rWkTfFtiK6vMEf9PlaqdoQ50OJItFRG1pWysKKkwZMI0eIGX4uDV8iGoLVGoBWy1Ot0rPfVVjdWEy22RiHibamzSaFCA4Ksxhi6iKvdaC8JcYlgIF6/NlaxRoow6yo3EZsIm9PjquQCpRba72+CSn1TaApmh43AiSxswuok9OdoljIs5ENKWOu0OchDEpRFVP9wRxIlUlHl7V2InvA3V3syjfCg97lJZj/W5UFU1QbLJabAQDoNJaJkMBNmm4G0ui02N2EzVFT5+soybnyzMvO4TwK8HETsXJOthB4qrPJRSPeWzVoDg3oNoPvV4aP2g8NWUZAhnkGl9TXgtekRgFd6BtIFh6OOCXimLeVWG0wVQ7ytNU5TKk2HY3GOcLVZLaiTqFTwZEYF9Q3dDQKEc7LWZbbbzEczR1k+9qJgCZxEuSXfasUllAuW4RiU0NQ3w4sq+tb7OioJm57yczOlMuMKedAlzswUUIQoRIMtmb60EWyqhAQ5xHYd4nRnVhd63ppdctKhLvXm7EJZpw6Xy2y2fsWSDL8SXCfJyedmykx6Is2cfGamQMZLl83JyX2tWXmcobWRFWjjt9sdpJ12WkiHy0zazRQBbAGCouwOGyRsdpfjrN6ZKWRHp5AoSAouQwqNeiWhjRRwoFVveEUW0maxo8wVokFMsSoN69SAR9ADlQsNogT12dpPBXEKUGjSpVmv9Xgaq0uqKstONeBT6Yt7xfTfjB5ekHkmGEzWQQmxQetNDQ/UuSWYLCvHa0satZMui9kKzRDaKcJqpUknXop6YtbafbJ36W2/B7AIe4zSBsKWIsxttVqwQgMHipx2K0Gk/oxsS6an0OXcL5bvystJPXN311UJ14iFg5/X5z2zaueecy3vN7ePLmo+PWeQfmrhmiRnO7HiFrV58Qe//MYrj1yrtGz9ztLty5bf+WTtMqz0+0tefnirTe37Y+C/nwxuudS/8e6/N+7/jDv+zs3lQ6tuDw+c9YIh8/B/8l8rHBh/NXKy+Mq+j7cGX3azA78bWtGIL/m586HizgXfwo8M8fuv/zY50XqPeGnPRwUuMnr5saarV/tfWFy66K83Invn39u2+9Keiaodfx5a8O3bO/JyOz3tc4eb+Px9i1bOvbpXebJ5ZOhr5JwDtVjI98Ib4mf55TcvPLnas+WZic//8rcrv/59/b+4R5/t45g/jT93uOLTc8Pt+fP2bTkx9rOHx26E6i/n5Vb+5O27xX+PWBNPT7zozaupu/NAd/QXF4H8w7HiU7m3u68L7+Ut6aKU21/YzZe2foQ/+tPXD9aurmi+cKH39CPSx+cxX/dLo6NPr977Xan55gOHv/6j/PZlr++c99B7ptEFo4fKz18/nP/8wYGRwFj3TjBe/s/357k/PLT03aEPPyh/583iisSt7z3L7j7x+K5thQvzP51f/4Th8cF7AweazxY+eKbv3qq7OcTzo0CL7pgf+rF32a8Wnmq/039s7KZhacH+GwfAuHP/qSfmvzK8i6feHj9ycaXpbN8fwhcvjhYfnvjH3W++u2Js5VOeK7dM6Jq//HJujrk3ea14Xk7O/wDBk1ep
|
File diff suppressed because one or more lines are too long
@ -1 +1 @@
|
||||
eNrdVn1sG2cZT5sxJg1NXVWl0iTWkzexUvKe787n80eWjcRJvCRNnMZJ1sCQeX332nfxffXeO9txSBEZ7B9GywlEN5WKZkvsYkLarqXNOjIYowiham2lDS376KZOY6qoJm1ITEMp5T3HIYnaf/gX/3F3z/t8/Z73+b3P66lKHllYMfRNc4puIwuKNhGwO1Wx0D4HYfv7ZQ3ZsiHNDCSSQ887lrK0S7ZtE0f9fmgqtGEiHSq0aGj+POsXZWj7ybepolqYmbQhjb+1OT7h0xDGMIuwL0p9c8InGiSXbq8I9riJyJfPRkXb11x/ExlaiLJlhMmzYFCK5rl7CxSGGnrUN9lMrfnWtCnHUr0Aa0KUmKy8faugHVM1oEQXlJyiIUmBtGFl/Z5kepIHXiPA/bbsaGm/5JckfzxjgoKCCWas6ECDkoINHRAgQIe2YyGQNqAlFaCao8fMrJ8LCoxZBP+bl2/y/62cbxHklqHWynEwsny1Fc2QUA1/1rQBb3jl6URkyRvbFoIaETJQxajGA80khPSik1WGDk1WZAQlQtcrDVtmZAPb7vxGCh6HoohIYKSLhqToWffX2ZJiNlMSyqjQRlWCXkc1grvVHEImgKqSR+UVL/cENE1VEaGn94+R8ubqNAVeW25VV72yAOmNbrunEwREW7d/YJycFZ1i6SBHMyeKANtQ0VXCfaBCgqds1vQvrVeYUMyRIKB+Dt3yivP8ehsDu7N9UEwkN4SElii7s9DSBP7U+nXL0W3CBrcSG7g1XV25li5AswzNn9wQGI/rojtba8TZDc7ItsaBaJAY7jRTFg0jpyB36dNUSsyk0lqrkYh7GyyoMnQgsvhOmAoPjPfE5K6BXEc8m97XGeO0hJTePVAAbIiLBAOBIMcBlmZolmYBcvql3GBAlNsH421DhfDI7t6SmAwybK/V7bAdOb3EBfYGeh0uI/eMFYdjI85jQ5ZhyomOflW3u5VMHg2hIh4Z3tsed5jQiP1YtygXWiiCzskrUqusBVA6nuzXVCFt8bkIEvQunBrtpEfp4fZSfJ80UOht0zvbpGTfOng8HwRMHaHA8GHG+82vckNFetaW3eeD4cAxC2GTHBD0ZJlsme3gqRnCQ3Thz5X69Hsu0btG4aaZDsJJd7HP0JspjqUSok1xDMdTbCgajEQZgYr3Dc3F6mmGbkvBk0MW1HGG0LBzlfIVUXb0HJKqsduSfdEjO+mkB58cT4CKpoERqKNy5/aCwZW5D7o7Tq2cLEBGCtSVUi2tu1hjfaFULEiiI0lyvqAxkRIfUNLIETOn6y6mZXhpCCCgYXeGZyLMfF21SrwqKZYBLAMY9lwRkHOOVEVTyIbWnvXbh/gGyW4v3GpgGzlE7qnySjteXm9gIY0Q1su9FoWPRCK/vb3RaqRQJExafW6jEUbrsbCchhduNahHmGVZQcNzxVUHoEju0oNESDHhtBQgzOE5nuEy4SBMh3hOFNMRGBTFgMC+SIafIpJAXjtNw7IBRiK5a+1xd6lZg0VvyrQG2GBAILW2UIouqo6Ekk66w/CqwC2UaSHvIjge6wIxKJKxnKwx0K10jPa39XXHzuwF66kEEubKPV/RDawrmUw5iSzSGbcqqoYjkXFpoTKJNdg26p4OS0KAC0REQQoJvChxoJ0MotVo/yXejDdrK1Al2POie0oOtPqiPB/wtVAabA0LPMPU/g18r+zVqmfPb8I7fnhXQ+3XqO55pf8dZsviR1/reuShnX27vzoRo6Y3bx32z7bv5F5Tr14tPp09s+3Sv1t6hOv6F7bvuHL4s78tfpK78+gTv2o6qhp/vOfy9ocOHbz40XevXV9+4cae/Y+2vlRaeHHh4/2t1w5t21/9ztClGztOfzofPcF3HLy6U5UPX7rrwHzqQPWDV8898nrJd+fY5YMv0D3Tvzz2l57tH559bjl694TVNAreFxobikeusGn5k1984+vP/u6BRu7wPW+kW+44uvXze/c8tXTyGWrXe+7Ppr79zODNrtfaSneM+8wnr/34lQd/TgmN91//+1b6cfVPb8IPbzx9/Gzbww57ufXiclPq3PsLxtb7ygyNpo99JX9k0zt9P3j8pjPAXt4ye2/p98x9I8OzoafGCh98efJh3J5efrk6/4/Elw43nQ9fnAgl//rm/W8fOXPhn29NPvGvZ8+Xp+9+94uH1Pzg8oWp2Cl7+tVtP3l7+Q+cUN71o66534wsRScaGxpu3mxsCP705MyBzQ0N/wEq8t3d
|
||||
eNrdVn1sE2UYL0MBJ4ZgAEEEmgNBYG/v+rGuLRJXxpgT18HWgJtBfHv3tr2tvTvug22QQWQoCQh4fMSA8jHWrdJMWBk4BwwVI98BMUCsCyAQGQaMkQXFBJ3vdd3G3PjwX5vLte/7fP2e5/k9T7osvBCJEstzfWpZTkYipGV8kNRlYREtUJAkL68JItnPM6FZufnuKkVkYy/6ZVmQHCQJBdYAOdkv8gJLG2g+SC40kkEkSdCHpJCHZ8p+SEpbTARh6XyZL0acRDiMlMmSQnToEI43FxMiH0CEg1AkJBIpBM1jFJwcl8hlgiaRUamMJfEvB+EUkV72Iwm/S3g9G9T8aBd6CQbRK0R5SqddXIYNJV4RaXzRKVDEAL7W3g6iIxdFCPCQMZSwxWwQMSw08KKP1E6CdiJxckFcFlL2K0EPyZAMQ2Z5BVDCShiwxHIgCBlW4jmAkQAOyoqIgIeHIlMCA8WGIsFHmlKtlFAK/psVUf6/y2de+TxMAJ5BGlo6ABUGATNIBdiMQzIIQBmTjigP+xFkMDMv6QaH/Lwkq9EebNsNaRoJMkAczTMs51M/9S1ihRQ9g7yalwiteYzTWY0UIyQAGGAXovpSIMmQ5QKYg0DGxeEVWd3pynXPz8qek+mqaXeq1kFBCLA01MzJIgyuNsFMoBW9pzii8RfgBnGy2uDsgEnOKsOzw+kpg8VuoOruDx2AGHGNEJcfuF8gQLoY+wGJuVRr2o133a/DS2p1DqRz87u5hCLtV6uhGLRaumUpKpyWqBrOmNUzXELYGS5sNhiN+Il28yyVcbRa7YUBCUU7m9BpEzFRJjOgrIAyNnTzjWSxDNA8DqFWUrs6KhhAnE/2q1Wp1rRPRCQJmDuoogabyYq0LISbiU4dCyd2xI7cmV1UGB6ajhurNs0Q2RS90aZ3CqIeh07VGy0OM35S9Vk57tqMRBh3r42KukXISV7crMwO3oRpv8IVIyaS0StjYkRXxiKOH2CDrAwS6xE3UjuqIQtFUbHxD9UUURBXRosYMtvt9kf4xZVBsrpXyw9QFmC0uRNZmgp7j8NygoLpGd+0CVQ1GiqMa9Ij9buwddiMfwybByA0F8Ym9GaNR60HxGpbPNrkR+t3QUzYTHgcmwdATC2M6Xsz/1f52gONe4jm/YVr19Y/VPuBJYskOg9YRj2If8+njMYMV5bLLOVbTJkSnFZiQaKL87oau/zjpQ45dlGc3ZpdbJzZbjWnMmYPQB4vAyx2Wxqw201G4DGZbIzFZkyzMNaqhSxUI3jI9T6e9wXQbtoLaEjjnd0+g2p4eoHLmZOdUfsGyOM9PCajG2LScjyHavKRiMdejdABXmHwohVRTcYMkOcsUPfazSYLMpkpK4PsNq/NA6bhBdUxjZ3TFtK2dPzfwTt45kV89U2fPWNWDdDFP32Z2c1FI50D/xpa2aLUbZl58tDQJBAc87z/6eDEGVPGevufWWMdm3PhyN3LJ7kXXr2xPj2rhfh5+Vl6VcV7Fxe1Xjn69ZWWXz86unbLkqYv2m6nb7sZ/uNynXPKmpdzo5OePNJvVajRGZm9eg+sqCh1fb9jpnvq7l1zGyZv/Dg2p/nO7976S1zUOSFmG/nZUhe548iKTYc2DFsatb49dXJFTtKC7V8mu4ffTT/93YJ+TvbHyXcvtQ52REfv/fCpAeMHnxnhqLw64bXFwvmU67oLz+2s7F+1QT/k+sDKDyadu9Woc8713Hhmv3uEvHT5xMJB+ed+G2DIvrP/c+eOW+fPHku/dsw95PXt12Kbg/2cyQPde7eNzW+tpd8tuDKx6NazGadOJo9qLH8pr37FprCJeiJ7a+uJ7HWHR2+11amZx/Vnxq3eSs5+a974RaeHjaouaD5OrTyy6Xz65oaVdsuqoz/dnVv6d/P2ze8fOBxaF808vcS3jzmoO7F2Yz0dkHJ2frX/XtNUeIret+X41nrP4qbGpuJv1ZtlLclkQQmfdYEshbfb6LxflJaLF/+8N0ina2vrqyu2XU1an6TT/QMhrxTS
|
@ -0,0 +1 @@
|
||||
eNqNVQ9QFGUUB01TEZNySkvh3EFNYbm/4t2pJB5IJhwEV4AE9N3ud+zK3u66u4cCYolUBpYtCIikph53chFCMpGjNlqTWqOOjaVcWua/STNrpnIsNem74wBN/HNzf3a/937v/d7vvbdX7i6CgkhzbHALzUpQAISEbkS53C3AxQ4oShUuO5QojnSmpWZYtjoE2juJkiReNCqVgKdjACtRAsfTRAzB2ZVFaqUdiiIogKLTypHF3oulmB0szZe4QsiKmFGt0uiisV4XzJhTigkcAzEj5hChgEVjBIdIsJLfIhXzPosEl0rI4v8xYglQJATaChUSBRUkRzjsyN2IlUX3+fceIozIOQQCnfXZHAKDjn3fRqy3Cp60cdZFkJD8FaA7pQjsPANj0CVWVpZblosYcyT0YQgGOEiIa/HpuMixLJRwBkhIJKzMTUFAIiV/DApzUpwoye13qbMdEATkJRyyBEfSbIH8UUEJzUcrSGjzRfEQvoh++WVPIYQ8Dhi6CO5YiosSoFkGiYZLtB1yDkluNqda8pPmv5xodvUEldsAzzM0AXxw5SJEriUgJe4r/W6zxyc4jrrASnJnfC9NZVox6jWrUMXoDDGqtttTMwAxdvF++67bDTwgClEcPDBHsqsH3Hq7DyfKTSmASM24IyQQCEpuAoI9VndHlYKD9RUqu01pd6cLGPvSubUxajV6t98RWSxmCbnJBhgRtvc1oQ/j0ag0WlwVi6vUnXfEhpJQjBMcSiFvVrX2KshAtkCi5K0arWabAEUe7Qdc6UIwySGWO1Ez4aGD7sBQb0ld0D8KY50JqLHynnkCHa1Q6xXxvKBAqacr1DqjVmfUxSqSUiwtpkAay4CNarcIgBVtqFmJvXPjJigHWwhJj2nAifFi/RULKD9D22kJD6wzaqTvVnbqVCqVd/J9PQVoR8r4Mjq1BoPhAXGRMlCSO3z14SodrtZbAlWqFw6ch2Z5BxpP/6MhwMrlY4V4TXugfz+3Xszkh8Dcg6FmoXfKQGi0andRbNL7s0U92L+fYgAz5WEw96AYu9CrGAj+P/l6EkXex/N24Xq8Fff1vqdknkDncZqUd6PrfJVabTInmXXZMxLjs6hkQJloW+JC8/Sd/fE5oQCwdIl/un04b6TWEKudTmqtOLTaSFxn0M/ADQaNGrdqNHpSp1fP0JGxW4toIHvQkisKOK6AgdsJG04AgoJ4zw7K7oRsc3zKfFNLFp7OWTk0jBaAhpblWOjKgAJae9lDMJyDRA9aAbpM8/D0+Gy5w6DV6KAmVg/1ep3eNgPic9EDqncb+7bN6XtK+//NVqCdF9DRl4NgRNWwIP9rMPp0dzMZC7iTqpG3oiqOmN+zlQXFjUpc4t1qKvXWTXw1ev9xLMPz/OGQylvX4ouqi66MWhFcfT2PDH9nhyt2T8flL721y3/89c0/xc5dN67+e6x02d9PLzkdt+7ri3HpDVgY85Vl8pD8Ec+eTZC/s7geq9vdnHJOnbN+T2PuoUhVzZG6qgv/eK07dbX0oKj29dcPnAuzXEotjlu+SDxtCF4tq4ZTr4cGl78aMXvflauhXUfPb8wC/Pf7JlOPXpikyJkz9ASxQPz094vF6WNqvv4rKH5DSXntzpmgdSSz8hpQhDTOppJ+mlDf8GLyN4PPmkd8cuTYeFbzRdH1w5m2yOTIEWDmoPcPVgEpWvFX4+Mlq9a1pYydOXVHhGlL+CtrT62JmzbWeEJ6o2N4WUTX7xNDsleFP/NLeefa/ftOhO9SHTwY3fXEeOvQAx+YXrc/v2fvyLQR5qkbsZqMRYnmcQkvffyouvpkXcKEzp/GlJyqLxvTmt7wuXL/4nWj13QMKWZG57xAnumsWX2rLfP8m+8afsg7fShz91mrrYL/Y24FMYeaNTFzdEZX9aqQ1eGzfjPFnZpzyf1zFqgZtkRzQTuuamJzyE3M+XYluJh2Oq8Ry0l+sqI6qmH8dtw1euqKBKay++zHB7411hmWjeu8/GH1scK9oZWFe9MjsnRntk1yduc56spvlniHNK96bp5IbrKua3Rvqg0LXbxh2KS25tD23EIzMyp081vJp95rNpTmN8y6teZy0jm9LbewK6yqub4+M7OWbrtE/RD5dFNr94Ts8TfePvrZlTdalFEF9U+lpr4W7JulwUFReZXHyUeCgv4Dl57hHA==
|
File diff suppressed because one or more lines are too long
@ -0,0 +1 @@
|
||||
eNqVVQlwE1UYTkG5VMQqtMoIIRTQmpfsJmmbZCi2tBwFmnSgaCmF8rL70myz2V336AHWoYjjiBZdULAql6RJibUHVqkn4gUiakcULSA6g6Iz1dGRDohcvk3TAymHmUw27/3/9//ff+6qcBkSJYbn4hoYTkYipGR8kNRVYRE9pCBJXh0KINnH08E894L87YrIdEzyybIgOc1mKDAmyMk+kRcYykTxAXMZaQ4gSYIlSAp6eLrycJy6whCAFcUy70ecZHCShMVmNPToGJyLVxhEnkUGp0GRkGgwGiges+DkqESuFDSJjCpkLIk+nIZsJFEi40F62Yf05Qjih6hnOHxkJD0TwGadhipjLzh6g9ESr4gUvugVKCKLr7Vfp6EnIEVgeUibyhk/E0A0A028WGLWToJ2MuMIAzg3ZtmnBDxm2kzT5lleAZQzEmYtMRwIQJqReA5gToCDsiIi4OGhSJdD1m8qFUrMlpRUQqgA/w+FwzFQkPKhYi05OF39okCCDwVw1VhDVdWSqiU4tTyNtJAoFio0AlaQArBtDsmAhTIup6Eq7EOQxjU/prst6OMlWW25rI5NkKKQIAPEUTzNcCXqqyXLGcGop5FXsxKhNIvRRlEjfoQEAFmmDL1WASQZMhyLqwtknEFekdUdLnd+8aycB2a4Qt1G1WYoCCxDQQ1uLsXkGmI1B1pMl4sjWmcAXEVOVndl9tA051XiruT0hMnmMBHN/V2zEDMOCVH52/0FAqT82A6Idbwa6gY39tfhJbUuF1LuBZeYhCLlU+ugGEi1XRKlqHBaoGo4K+9ydzFhr7uw1USS+NtyiWWpkqPUOi9kJdTSW4ReTMRCWKyASAUEuesS20gWKwHFYxfqNqKxJ4Ms4kpkn7rdRpD1IpIE3GDo0RCGyYq0KoiLiQ7sC8em72X33L5WSAxm48Kq784UGaOetOszBVGPXafoSZvTanOmWPWzcvMbsmJu8gcsVEu+CDnJi4s1o6dvwpRP4fyIjmQN2DEdhr6IReyfZQKMDGKLBxdSO6pBG0EQHZOvqimiAM6M5jFodTgc17CLM4NktVWLDxA2QNrzu6O0OQoH9sNwgoLbM7rDYqxCGivMK/ma+n3cejCTrwMzMMMUorBjykBoPGqXUayzR73dd239PooxzJTrwVyBorWwQz8Q/D/p63aUdBXN/onr1tZfVfuKKYvEKg8YWn0H/y8mSDLLNcuVkuaF4hx/djZX5vJYCly+N/vs480POWZ5tLs1XEeS1ZFqTaGtHoA8XhrYHPY04HBYSOCxWOy0zU6m2ejU7WUMVCN4yPUlPF/CoibKC6KrG3TPoBrOXuTKzM3JaigA83kPj5sxH+Km5XgOhRYgEY+9GqFYXqHxohVRKGsmmJ+5SG11WC02ZHE4aA9B270OCkzHC6pnGnunLaht6eh7txrPvIivPh40afyTw3TRz+Ci+Z9xR4mbz42evnHpO5k7c9sPjW+/o6pozdE1nsy5RS8W1IrZpXdP3Lc7YWfasK/W69d9WDvhzG/2tkM1h8c38uPeOvf32b0bz0y5MOKH3WUHz39//sKRjpQvNjxROnqHx934kOPjuzIWPpBFfttG3rimUZnmGd56tu3tI0z8hCO/N53elfbt2Xf9L7k9SScO3/9lU27N5uSHqwpPE6MOrE7NGzKmuXp0xUebdeEniPsKVq3VbZto/GRv/NjnicVF7+/N/2BQXMLzHUO2TnGT6xJfWvTm8X1vFGWi9oODGlYKJ0zbE+WW9iEvLArfOPWXIrmzfuuGT89lxLX/EhzBLt4yd5x1z9Tm27aufjLPvmCpXrz/u7vSudCGIa1d6Ttaun4k195SDxN+YM8mVdes/3XmzlP13yQsq7u4ddScxsyxw2aOnBAfPP7PTW22FzeamroKs4eOGbS25Smjr+n9abcP77pz4YOqNKf6hrdWOw4kul9ZOW/8HUlxc5c9vbAA7d83vy48NfUZyz2b7t1d+13c2I+edY1Q3Urx7T9PrPQv+ynx2IbWXJ93+Ij0LTnLZp+ccTS58/vDnS2PRR6ZV/jg0tDnnmMFgYzqtaV7MipOtGUkHDSuXJJc9vPSC6kukPv4vPqcos7ZH+yZM6Y85570MfHuxufiqeP7l//5+2tU7Zq7jZtmf/36rpqTvw51/j2vc/bIP06mlW853TSplji38T2uKPnUyOaLXX/9datOd/HiYJ11p+NMzWCd7l+O/AW4
|
@ -0,0 +1 @@
|
||||
eNqVVQtMFFcUhWBNSesPatQaZTtQCHVn/+AutFVYkaDlIyBVROHtzNvdkdmZceYtHxFT0aZRInGMn0YiFllYu0GEQksVq1YtNRVNtaaKMbSptpjaRq1S06TVvlkW0IqfbiY7+969597z7rn3bZW3BIoSw3PBzQyHoAgohBeSXOUV4So3lNCGJhdETp72ZGXm5Da4Rab3dSdCgpSg1QKB0QAOOUVeYCgNxbu0JXqtC0oScEDJY+Pp8svBbAXhAmWFiC+GnEQk6HUGk5oY8iESllUQIs9CIoFwS1Ak1ATFYxYc8ltQuaBYECxD2OJ/JRDzoESJjA2qkBOqSiHAL1HFcHjJSCrGhcMmEJXqYbB/B6Ml3i1SeGPY4BZZvK18JxBDB3ILLA9oTSlTzLggzQANLzq0ykpQVlp8QheujRY53S6bltbStDbVLpCljIRZSwxHugDNSDxHYk4kB5BbhKSNByJdCthizUrBoTXExeuEMvL/oYjKyuWVy3HVeBoqbCkWuGlIGsk4EsM4iEgWIKwUUel1QkBjOfuCJnmcvITktsckOgAoCgqIhBzF0wznkPc7VjOCWkVDuxLFRykR/T0g+4ohFEjAMiWwvYyUEGA4FgtHIlwc3o3kjzMycwtT0/JSMpoGg8qtQBBYhgIKXLsSk2sOyEkqRX/c7FNEJ7FAHJI7k4ZoarPKccNxKp3GZNHoWh9OzQLMuEnw27seNgiAKsZxyEAzy02D4JaHfXhJbkwHVGbOIyGBSDnlRiC64k2PnFJ0c8pBZa816/F0AeNwOq9Ro9fjp+2RyFI5R8mNdsBKsG1YhGGMz6AzGEldPKnTdz4SGyKxnKR4nEKu17UMVZCFnAM55QZjvGmfCCUB9w5c34RhyC1VebCYsOeUNzBYezMXjrTCVM88LKz8xXyRUav0ZlWSIKpw6jiV3pRgxI9BlZqe22wNpMkdVai2XBFwkh2LlTLUN17K6eaKIe2zjtoxvcTIiUWcn2VcDCIDdwoWUlnKHpNOp+uNfqqnCF24MkpGj9FisTwjLq4MRHKHcj5SZyL15tzBUxos+aPnYTjBjdvTfz0FWDUprDCvN57pP8JtCBP9HJjRGRp1+b0xo6HxqD1GsdHszzbr2f4jFAOYmOfBPIGiIb9XNRr8P+UbTBT1FM+HCzforXqq9xNL5gsoTzK0fBj/LtTp9daM1AwjvXgRYpKd4hJudn4GPd92cCQ+vtQBx6z2d7eC640yWuKNcbTRRkKbnSZNFvNs0mIx6EmbwWCmTWb9bBMd31DCANmHh1zl4HkHCw9QdpICFL6zB2dQ9s5bmpGUnmZtXkJm8zYeN2MuwE3L8RxsyoEiHnvZR7G8m8YXrQibrPPJ7KSlcofFaDBBg95mMtvN+LGRyfiCGprG4WnzKLe0/y91HZ55EW99FXwjovrFIP8nhM7+sua7uS//M7lYU3B+8bKSSd9M/lyduiM8eXvi1ei8mLrm33vDfPcTFxxKaTZvvnbv7772t/qPxXaAVq6zb80Dw5XSZRFH1lZMe/vG1J9v3773S46zKjPyjNpwoiY5vOPawffsbM1P9aHWtG3W6bbuXc6BtQOtnxDJRy63dN6rHSiZvmtitXnjB39+/Vf0r3eLucK6+DcHxsQk30xb98JhR8jM1shjq6JyQ7StMXMvhef1W8+MO/nOvi1CVXjFhEhff6hNE5delBrdtd5bty1o+48n4zMmLv308na6qGVD6o7xupX62oHtXX1gz6x1M7wpwX8U1DJ5XTU94y79MM3jk85V7MoxT7hwbElP2Z6YU9fHlAnZsSkfXZ2y9f3VyTNevb4gI+pWgb49M2v8S59NTToYlpjSVSOc7uo5d0etDlXvTYxtHLskLLWAmLll4KaGkk+eTfntUGRs0ZWNZ9ZuUme90lM9fnZEz4mj6e3151/LQQsunp74oXT2IlpYI+vO19vnRmzafLq7sL93ygV0fM2Y6ig0RTOntmhF9+6wfPnbsVs77vjCVgQ39C8Ii+3Ilrjk5fdXVIxXUwO7d0ZufL/kzq3b1nCiu65kUWj/4a7ObQPj3j1+d06e7+ikAl67cw5W9cGDkKD9dd/vnhoSFPQvid/bWw==
|
@ -0,0 +1 @@
|
||||
eNqVVntsHEcZd5UqIEJBEVFLkVqm25AIenPvc+8OFOE6TpqaOIltWhqIktndudvJ7e4sM7M+X4wFsSukCvHYBglERKsmZ5teQxuLKlVUqKo2EqUU2qrQyJUKfaj9J0X8gQQSUjDf7J19NnEeWOe9m5nv8ft9r9mpuTEqJOP+daeYr6ggloKFjKbmBP1mSKV6YNajyuF2c++ekdGToWALn3OUCmQ5lSIBSxJfOYIHzEpa3EuNZVIelZJUqWya3G68ef2hCcMj4wcVr1FfGuVMOptPGEsyRvnrE4bgLjXKRiipMBKGxQGFr+IT1Qj0iaLjCk7ir7KxnUpLMJMi5VBUpwS+BGI+LJlEzAOzZWMysawc74C25KGwYGP5IBQubOtn2VgiFAYuJ3ayzmrMozYjSS6qKb0K9CoFDD2ITUo5oWem7JRtp3ZWAlxnElBL5mOP2ExyHwMm7BMVCopNToRdJ24teTioprKF3nQwjv8/LaBjWMRy6EEdHAjXChY0cKgHWXONyckDmnYnmkRKJhUkZ2VIjdHLhQyRIKBESKQ4gtASeJBQsUroJlBAhHIbyHJ5aDeQTRqozpSD6LhFXRfsojEmmclcphpJpD3IWgOBYQIHpgAIyHRD2lYC0gGYYkKEsm1RIqkEVcDORsQSXAIi1bbTOScClB2mAJZvd4AiKNoGclnVUfFupU2qkUAyrEJhKeZXkUVcDzYUMd0ubQiGzeISbzuJbWjpjqJ2v1UiGwodNnWNecy2wQCvxCvgn2hzMUXsXoa+dJhPEXPd0GOQwCXFqqDUhyfkIgZZ59yGjW5uNQBBwZvPIR02s4gGpj0JwnwNnQtgwAWSFAh3SWAAiVzOaxLw13S+Aioq1FIJQOva3SS1I1QH4xJV2Rh4d3i9Ay6GtSrtumBsjd7jUqc8DNoURdurcgigp1rAoWSMgYTp8rqPiMlDlTRWlN+1NvNI6HlEsCO6m4nSNVmAMAlbgrUDkwdgUkDIdIdaLgltinO4gKFVfKqwSxSky5icAyw2jLC/9GxsOoA7mr9kLD1JLIsGClPf4ppf9MvqERYkkE0r2krL0hbjuRe1apQGmLgQq1+NYwgI810YVljBQACS0WNDe0YP7tx178DQbNtodBpi6HZSlzoM4E51WGPN+NLjlo4Nhp7zVfR03xLM1N4GDFkfpZP5UjJ9eqVrlwDi2SA+f2blQUCsGtjBnQEezbaVn1gpw2U0s5tYe0ZWmSTCcqIZIrze/CqWIvQ10Wiuf++l7jqHy+7mcslMBj7zqyzLhm9FMxXiSjq/nIRlnVY2nc3hdC9OZ55eZZsq0cAWBxfRo+knliII46WqnKiZyWTTvxBUBtC2dHoW9FQop5qQTfryi3Od2+TEnsFuLXy6uR0yG/1mh2AJlCmivkAg8F1AmXw5ly8XCmjn7tFT/R0/o2tman4UppesQLYGlgpnznJCv0btVv+aJbNgdClD01CXeUzhzkUKmdTLqJlPp9MLW64oKagHodEem7lSqXQVuxAZqqKnND+czuNMcbTDMrd/bT/MD0Koz/hO7qCa1agA1xeuKt/FtqSz5Rp0LoMwv39h61ra0GuXQJwpxt7uuLp8F2JHZ+u16FwGYmH/AlpL/X/C13a0+QqSKwPXlkZXlL5syFqdzGNmR7+G3wfTmUz/0M6hwogpB746zO7KkcFhNnTk7rNd+/AmQ3x2JK5urbewOVfqzRXsnImpWbFxvlS8E5dK2Qw2s9minS9m7szbvSfHGIla0OWoynnVpU9aFRy/iuB2D0Zz2+8f6tu9q//U1/AwNzkU4yiBovW5T2dHqIC+j1rxPQ6TVtDZ/h14uO/+6KlSLpunWbNgmul0sVKy8F0woZa6cbnbmnpMx++RR6Hn9YV8bvGz3/toT/y3Dv4XF7/xg77BF7686YHFe376jx0P5QcWTn7klRMDG6PTb33p8MsfeD/714033LZ4YP+Hjz68/oPjN1f6zj9v/qh/5MfP3HJh8J4/v/7eCw//8OLfLpy/WBmfPvrH238+vPndT1w//fnvHntne3nfbz82fduZTYfemR7E7x//1vFBe8sfHj/R+v2J1qG3b79j/vlnUz/ZN/PIyMXEja882Ohd2Lb5pebf7/7KP9/6XbG67dyfXrrhjU+ue63Y+6nSgx/f8O6Z1/KZ/3zn1czGian3N031lb99ZkP51mO9n5kwj+ILbz73xcVd33/kRXbT2bNDm27aFtUW58XEfTefa54/duHfGzTLdT1/Xb9+377renr+C7bLfqw=
|
File diff suppressed because one or more lines are too long
File diff suppressed because one or more lines are too long
@ -1 +0,0 @@
|
||||
eNqVVX1QFOcZPyVNIBPUpGDbyUe3ZzpUw97t3u19Qc4OHHhBQJCvYhql7+2+x663X+zH3QFSjXEytVh1zTRJaeq0ctxZQsAERdHaZExUEtO0TTK1YEpnNE2b6EzaVBtRE/vucRQY/ad7M/fus+/z/p7f8z6/93m3pqJQUTlJXDDAiRpUAK0hQzW2phTYpkNV25YUoMZKTKK2pr6hV1e48RWspslqkd0OZM4myVAEnI2WBHuUtNMs0OzoXeZhGiYRkpj2iQWbOq0CVFXQClVrEfb9TistoViiNm1o7TJEb1YNxjVrYWZENgNVWuFCENNYiMUgQIOCcSIyORXjBIRm7SrEZtenP7XoCm+CzBpFyGV6tM4Q12VeAowtxkU4ATIcsElKq920ZNMyExAQebvG6kLIztgZxh4My3iMUxFvlRNxATCcKok4YoSLQNMViIckoDAxwEdsG+VWu8PlJuQ4/v+tsnZ1rUfMFYlPp6OrULGmvwgSA9P8W2UNpyQzPRGZJBpVTYFAQEYY8CpMb54goyqa6OgrYfN0pVgIGFTjScuSBCupmjE4v25DgKYhAoYiLTGc2Gq81NrByYUYA8M80GA/Yi/CtCqM/giEMg54LgqT06uMA0CWeY4G5rx9I0pvIFNb3CzLrdP9Zlo4qo2oGQdrEImSCnttOxKYiJE2l8NGHIjjqgY4kUeCwXmA+CTl9PyxuRMyoCMIBM+I10hOLx6c6yOpRl81oGvq50EChWaNPqAIbmp47ndFFzWkBiMVqL01XGZyNpzTRhI26uV5wGq7SBt96UIcnrcYako7TksIw/gVkaQlKcJBY/yzlhY63BIS/FJN0NxgN88CHUCFKgct3tr21QF2VW2kLNgaaisPOIQaJlRVG8NJj8PncjpdDgdO2ggbaSNxqK9hInVOmi2tC5Y0xLxNVZUddL2LICuVCp0si4gdDmezs1J3hNnVG+ONgSb9sQZFktmasjW8qFVw4ShsgHG1qbG5NKgTnibtsQqajRVjiJ0e5Rg/KzhhKFi/RuDdIYWK+KBbXKW2rCu3rbM1lnYE25jaWGWJWF7C1FfPoUdRLpzIMHQTlJcwn8EZbfBQbNVYo9fpJfYrUJXRAYFPJdGWabq6NYF0CN8eS2Vaxr6aylkJL02UIU0ax6slsRBzkFgNrWEOwkFhpKfI5SsiSCxY3TAQyIRpuK0EX25QgKiGkQzLZySfolldjECmP3BbsR83xY4qadJHxxOHcVlSIZ5hZQw043XTzRKvKBuePlk4ailA5DrSYY3jadXHOuIxhtYZho3GBMLXQTlRd9Pp8MHMElmRzDCIEC4g7ZI+kiIGM3MzyutH2RI4SeAEeTSOo4MOeU7g0I6m/zM9WzUSLrTdR2510KQIRN09OV2P3851UKCAFGsGn0WhfD7fb27vNIPk8fkchO/ofCcVzuVCOgT1yK0OGYSEyyeoA/EZf5xjjPGHkdHi8RJunxOQTreTIhkv6SF9XsIXIimHz+FyAdcoan4cjXDMcsqSouEqpNEFpbUb44UCiJtdxu8kXU43SrUY3Rw0rzOwXg+VSWYSajEmK9C8CIYCq/AAoFFbrk8r0EiVrVtTUl0RGGnG50oJr5GnL8eUKKkiFw4n66GCCmP007ykM6hdKjCJsOpK1hkHvYzb6UC/cJhyUDTjwEtRI5pB+5/wEmavTQEecY/SxjDr9FuLKMppLcYE4Pe6KYJIX6FPJs1cxdaTC+/8Zne2Jf1k8XXV0jninuPXv7fk6KXzeVP8xAdP/+Jp8NqW3O8cANns9pFDY/seebd6eN/Nrt3PuL8x0b3o03/593RsWmHZEQtnnRrZP3Lx9Q8vPHf99OlznT2TXwxc+vzKtXPrb1yroDbtXfzl0PLhrS9+l7lSrsPRi1m//ns8IVgb3hocvnIjeqh54KMXjX/8ee3YK8mzb+4p+PfHm3w/m6xKni0AZyb3PJ97Y4XFsveUZ9fXH/T35Fad+Vpi2b38GL+h21J1fve9T1k35PX2fFb1+M7VbS9cC1YeKHj/2fuuZk/cd3XR/QsvdZ54+Jf35D+Zuy2HKNr9rVOBnM4hHGt40/NSaf6dC8cfPHX3tp7/YEsblxtZ98dl5SujjWN/W+nGAu/t3r/l7OKF2V1Y3o67Fy3+9h2v9spb/MnBOz7Nznr/hS136W/n/KF/8z8/+fhkfu9Xu/0lP5jMefTqCjj0aPUzPwrlrCyS2vj1F7IKBk60t53549QDPTuEnjc6hSHb5sK1/uyPXju/5ETO5h/+BJPfKxj88I1Xzx/atWFCsObtnDpWPupZdv0Jy42Heh/6svL3l7cf23D4reIfj8vun//lgdzfTR195/LGNutP81/v4/2P/LXtg6K6yyW2d042H8RHh6OPPzHw+cjFzqWn87v4ySN5BQXL7lr7ycihXbl9K6cuLPjTF9HRV87ufPZwd9/yvd2bUcFv3syyNDGD727Pslj+C1CB3ug=
|
File diff suppressed because one or more lines are too long
@ -1 +0,0 @@
|
||||
eNqVVn1sU9cVd4DR0Q2BNm10Slk9M5UK5dnvy3acNGsSO5gQEpskREmqLFzfd5334vfF+4jtMG8t7R8VbJ1epMLUqa1IHbvLXKcosDIYdO3E1mls1dBWmjGBVHUaYk3VMlVlzSp237PdJIJ/5j9877n3nHN/55zfPfcdKk4gTRcUua4kyAbSADSwoFuHiho6YCLdeLIgIYNXuHw81tf/oqkJCzt4w1D1Jp8PqIJXUZEMBC9UJN8E5YM8MHx4rorIcZNPKFz2b2smD3okpOtgDOmeJvejBz1QwWfJRkUwsirCM4+BMoanoTpimUM61IQEchs8cqcRwIPmFmQsCrpbkLA3T67BvWzvLI2ammg7WRaasEpl9NSAm6qoAM6bFlKChDgBeBVtzGdLqi3ZAUgYvM/gTSnh43wc54smVSIt6Bi3LsiEBDhBV2QCIyJkYJgaIhIK0Lg0EFPecXXMR/sDpJoh/j8rTy43gpFriuiEY+pI8zgrksIhB/+YahCsYocnY5HCo25oCEhYSAJRR07yJBVX0faOV0lv0F5TFFFfneukKTuFtn19PrczJQPJUajme9S2tZUqxVCrep7IXWpjq6lAww4wj3THm6phfmiGgCpiTdGeI9m0cT/q0U1Zztq2UFRMzplpQMBLdug1vDhOQbYzZO9iYgoa4hzrmsuVykpiHEEDK+dGckUeAQ7DueranOcV3bDKq1k7ByBEOK1IhgqHj7BeHpsU1AY3h5IiMNAsrp2MnPRYsymEVAKIwgQqVKysV4CqigIE9r5vHBe3VGU2YWO5c3vWLiqBmSkb1skYBtHW6Ytn8fWS3ZTXT3vJVzKEbuDoRXxdCBFgPAXV2T+7ckMFMIWdENWraxUqxuWVOopuzXQDGOtb5RJokLdmgCYF2PmV65opG/guWMVw/M7jqpvLxzFeivSyJ1Y51rMytGYcGr66yhgZWpaACvZhHScLUFFSArIWbo6OwuRoQmpRYlE7wQGRByZAGtsBRhvj2d1hfmc8FYmOJQ50hGkpxiX2xNMEFaRDfobx0zRBeUkv5aUIZPZwqV4G8u290bb+dOPAnq5J2OcnqS6t06QiKXmSZgaZLpNO8rvHM/vCA+aufkxKPhbpEWWjU0hOoH6U0Qf2DbZHTTI4YOzqhHy62Y3RmRMC18JLDEpE+3okMZDQ2FQIBeSd+uhQh3fIu699MnqAi6e72uSONq6vewU8lvUTZBVhgGQbSftXrnFDRPKYwVsvBhj2JQ3pKm4P6IkCTplh6ofymIfo4pvFasOcjnUtU/jr+QjmpHWuW5Eb3DTljkHDTZM066aCTf5QE0W6o939pXD1mP67UvBEvwZkPYlp2FGjfBHyppxC3Gz4rmQ/Z5MdV9KGj5sTgTKqoiOiisoqDRK9laeC6IzMV24WgRsqkIVJ51jrnMP69GQmzUGT4/iJtESGJlkG9w8TJk9WTXCvsI/BgAhJt/IsSTPl6laNeLM4WJKgSIKkfml3BIjvmR2NqmgGoSOIXycjay00SCBjX7IWhvIzAZz5ZvxsQNHkUJ+ZiCgSpqbe7FY1ZL8CZzIEbpdIFCQBV8b5r758GIQfG5++U8FQUgi/kYVKXc+vVNCQ7d4OYtkLGwqFfnV3pZqnYChEk6Ezq5V0tBILRUv66TsVqh7y/pCklzI1fULgrIVvY2G0EaJgMgQDITZBBgAHko0sSNI0pGCSoZgQnAvvJMIA4jepzyGgVYwM9bR1d4Z/MUisZBIRUytfBkVZ0WUhmSz0IQ0Xxpp1+jbulhoqYF+9bUPWyUYuwNBMIpgASchCjibacR+qefucd3m71RaBiGs3Aa15nmnxNLEs42l2S6ClMcCSpPP98Hih0v0v1P30gSNfdDm/teLekdSV1s3fuzw3+MHQvVMNzSeufTrVOvzQk633zjz1sUCMaGfOXi2rez78gW/qka6b2iYmOM+cfWDDY/SPhh+/tPXwB0/88Xzu9tmlj25dOn/s9ZaB2PzsO5fmlq68ENv01ie+HVu/9v3Pdg/3zNYvLu3v/GH6nuFo+/E/nzpNHPv3gvhdYvv0JxsX9r69/vc7XngmO4T+M3LU9/Qbf6rvLg182FznuvWTq9R06vrIy+5YbFfs4jtTw889UvfS9cWnHuICDdcjM1t6D25/r/CXt7a+fjTedHj//bui+yfFDeS6N950f/PtdQ83vtoFUvm166QN20qLrb9Wmlsvu5aef8x/OJ5ff/Lj8G+Cl8PbpPW/PTFfX2p5d0n/0r+ubP7s2R//9xvX7hs7/fNt3zoYfDD5UVv3+9+pu8VIqVtK3Y7ItXt+duE1q37jkcTe+7/813+0bjnzsPXuFxZPvV/e85paqv8Ke3QuUGZvPP3skUCsfCP3/KfPXDg+fWBx+7H3ruczv2vPnaPKM/dNh/6ufvXGeHnLxn1/GJqZyt3c5HLdvr3WtebiqX9eXONy/Q8Vr/3y
|
File diff suppressed because one or more lines are too long
File diff suppressed because one or more lines are too long
@ -1 +1 @@
|
||||
eNrVVk9v3EQUByQuHPkEI4sTWm+8/+Lsoh5Cqcq/0KKkVVFbWWP72R5iz7gz42y20R4oPSOZT9CSKKmiliJAXKASRw58gXDgs/BmvJu027RFVEIQJbLz3rz3fu/fb3zrYAukYoK/ep9xDZJGGv9RX986kHCjAqVv7xegMxHvnj+3sVtJdvRWpnWpRktLtGRtVTCdtXPK0yijjLcjUSwxnoi9UMSTXw8yoDG6v314SYF0V1Pguv7RnLZ2bjlZ8tqddqfvP1yNIii1e45HImY8rR+kN1nZIjEkOdWw36jr72hZ5iyiBuPS50rww7OCc7CY68NNgNKlOduCexJUiWnAl/tKU12pW3voF37/7aAApWgK31z4aA7uz1fe3L8oaVrQ+h4XbkSjDH4y8ZRy0buWInfX6LZBX+8ue97PC7rVPBdj94JkKeP1nV9O1b7fhKrvvn26fs2W2Oi/v+K+RzWNRepuYDPA/SCuj8gwXg7BH/hd6PdpJxx2BivDOFnxw0HY8f2QHpFT3Z6VEGPJGc1VvadlBT9ssAKru4D54RXXmOfuue2SYeXqu97B/PXRRla1iNchH1JOOkPfI543sr/k/NrGfRMPI7gbkxKe7s4epgL1o3WqW6TbJWtUkq7XHZDO8qg3GPV94+LbuYuPgac6wxL73b3LVE7q/Qbfo4XUEJlQ8FhJD1Zz7a5vRfVRO+udcUb9fs95hxT0THcw7Hqe18p6bnd4imL3MqP1Ic4fSYVIc3hw1vR+Hqj+vqxCTKeFFtsuzsyZZc+O9Rc4UxKr+Mdrd3ac2fY4I8drD9u+77QcxnHmeAQBjm6qnNGOE+YiDJQWOGUQAKdhDrEzMg1pLeowYUBn6z10FOMgKNABbNOizEEFRZVrVlKpF528+ASuHi63hoCyAPdaThYPCJkGkQTbvCBmaqZMcHZQW9JJgU1aNCoxe8FpHqC1etpK9Z6VtQIqo+wpaSbGgdZ5ULG5SJslCDQDGcSVnKGjE1vWXPDUbDva97HNxlzqmaDTn7acsZCbqjQOVCRKMCgDxreYBnWM8abScYC0VeK0m04+iQmdhFQjUuw3kiEe5AlLTfBKwRPVjkuBBHqcSURzCKoyuKHYTcSPk5yCRFieBTrXcp1hyWMV5LiXaNxZnitjMeYBh6LUkxPrPmqNu/lp6+tYEIQTm1jXG/qdQdebTt94Nouvv4jF8Q+laulY6lK2pG7krp0eV02UhsItJVZOLxmOVvp/RPpf3Y9mtKNPZa4H9hpwoxkV/Acuhr9L8ftYdCSl+qDaYpGQ/HTKX2TdvU6v5+3FL6brf4+Nn+Ta17s7TjOSQUZVhhQ5iJejrgdDn3q0F0fLyXAw8DtD2lkZQLgyXPHjYRLBCvX6EHXixPd6/X6SDLv9oQ9hlIRIsAXlLMHBtWQS4UIh3+A4oHNsPAauImQvw+jIBled401ASTP3Ct9QovFxFh8XrXADl9aMr3O95WyOqWyugNkI4vvVl461bndvrfH4vKCN5ctkN/PQcp4XhvGy0sEWlcwwp0nRiXEgcUPRUIsy2DRPowvMt6G9ItHCONEzh0EiZIHJjZzEbTrunChReh73mxP8BLGWxHIa7mqL2CsLCCVqwrX5eEX2zCcEJ19ifLIzAzIllraIFkRW3DwyyEuSMB4TnaE9V2OQbXKJYwLKipDgJVElRCxhoDAwyZg6jmwiNrqI8KoI8axIyPweNg4mZMxUZkKJUGNdW4TmY7y5iCVrMhGVPAFFNSmEQry2XFOCVIZ3i2qTz0RFIsxbSNwvi2umIuEEMUjIYYtyjQnnVWETk6Arye1R69J+1hvQPD3Bx5oD5gsjxG+M9jV+jX8CyBszSNgOhJvbQ41rRRIcgcfTth1tEcGx3lRtNjbWIoHxIjJFUttCo57X0Ea9SNFaGy4yVUX8WPfG50lswmlhi0pt3WxBFMA8C4XEXFC8NlQkWWk9k3fRlkpIqtz45EI/ltgckfUXN1rYZkq3yWquRIuUi5jGGYuyORhmy9eIbA1sHhcMZIPdFkAYtjUVtwfU6BrfOVmAqTn/6awII7JjZ3rqTPHneuufb9N0evIhiFbXp38B114n3w==
|
||||
eNrVV91u3MYVTnLRi9zlovcDIoDRgFxxydXfGgaqOIbTH8cupAQuYoOYHR6SE5Ez9MxQ67Wgizp5AeYJklqQAsNuirRIUbQBcpmLvoB60WfpmeFSstcbK4ETIBEkcDVnzjnf+fsO9/7xHijNpXj5ERcGFGUG/9Ef3z9WcKcBbT46qsAUMn1w9crOg0bxk9cLY2o9XlmhNR/oiptiUFKRs4JyMWCyWuEik4cTmc6+Pi6Apmj+o4fvalDBVg7CtH+3t51eUM9WwsFwMBytf77FGNQmuCKYTLnI28f5PV77JIWspAaOOnH7V1rXJWfUYlz5QEvx8LIUAhzm9uEuQB3Qku/BZwp0jWHAh0faUNPo+4doF/7zzXEFWtMc/nz9dz24/730y+Mrd2uOKu1XO0Xjk3BIfksFGW6uhyQMx+6XXL228/nNwOIog/76p+GD9zhtH2IIJJcyL+GLm8Fb1NBU5sEO5hKC36TtCYmGaQjra/Eai9jqBMMNV0dsLU43hmtDlqXrX1mzWgcYjFHS2Zcagrc7gO2nb3x508kwfcHOrIbgeu2q1B4LqQXPsr/00t+DyE3RPlhbj75cMHqN3rUVQFkY/nPbKM6MxSgwUcoE28CwuGbWnvgVXsQUXYqHqzHeDS8SLljZpLDdTN6SFZZZXyS1glLS9PA9qmbt0XXFcy5OyILLrbKU0+CyghSxcVrq9tCoBv619Fpno/3k30ul11wT2lz8o4+1hxzckNgTiPxKpmgFARWob6TS5IKGMrtA+oZd0qwXiZx8gP0TaMXIBSEFXDi6oWhe0fYzIQNGWQHHWyU622PtyaCIL3nj0Sj2LpKKXopWNyPMj1/EQbS5RLA8krOqHmKrAPZcA9hzG2SrViQKo1UyjMfRxjhyPffoybo/2/+PL1uEvYf2i7qZoNwnfQ3Xwr/t8AoHaiHJbj7/hMOhUPbfVz7Z9+Y04I09HMlwEHm+h4U2NpkJzmCuvfG+NynlJLG5RdsJCDopIfXGtqb+ogzdABrbjtFQiiOhwSRwl1Z1CTqpmtLwmiqzaOT8G8ghyFIGEsoTJCg1W7wgVZ4wBS5HScr1XJhh+6G0prMK07moVGP0UtAyQW39rJaOvy1qDVSx4pnTQk4TY8qk4f2RsXSQGA4qSRs1R0dnLq2lFLmlLdQfYT9ZdWXmB8PRge9NpdrVtTWgmazBoky42OMG9CnGe9qkCbY0Tqa2lXwaExqZUINIsd44HXhRZDy3zhsNT2U7rSVugtNIGC0haerkjub3ED/2Tw4KYYUOaC8VpsCUpzopsdtQebjWC1M5FYmAqjazM+0RSq25/razdXqQTGYusCjcXB+uRuHBwavfvo62z1tH+IeneuX0NKB8Rd8pA9c9gZ5pA1VQK8ycWbHLRpuf1fZ67bzl8F1peXGXPWJzq2Yp9fxYLP6r5Sy+hKkXt97hENfV89beEdYRea49bvY4k0ocps8j4OGmJeDl/PnYbYaAzXn3xXfFua8A5y2TH26lP7UafvHrfa+boKSgukBGRwM0hM00zMJsNcWPsLkxDGmEn2jMNlMWpxAPJzHFO6thtB6NopDFMYw2YA3iyO6DimJfYnUd9zGcf6RH7DE0jglFxw3DwtsFhOT1vnc6uHjSjanGT3hi8HEZHzfc4Q5yjJ0277bv7U6p6jbWfGLw8/sv7GvbUcW1zuLznHaaLxLd3ILvPc8NF3Vjkj2quCV6G6KX4jjjkKCikXWya59Wlth3cqts5oaSTKoKgxp7WdBV2jsT4ulVpCFB8CXY+SCOenHwfeI2KxBK9EwY+2UBSb6cEZwmhX7J/hzAAXHsSowkqhH2UUBZk4yLlJgC9YWeghqQdwUC1+4I95AiugbGMw4aHZOC61PP1mMnY0Q01QTvyoz0rwvWwIxMuS6sKzkxmE+f0HKKC5a4nUJmslFnoKghldSI16XpgCDj4grUA/JH2RCGcUuFc+VwzUVkMkMMOCKwR4XBgMumcoEpMI0S7qoz6b5GWdAiP8PHuwv2RWiCr0KDW+KWeAeQi+aQsBwIt3SXOtOaZFj6J8N2lfSJFJhvqnc7HaeRwXQRmSa5K6EV9zl0Xm9Q1DaWNm1WET/mvbN55psIZGCbVOry5hKiAfooNBJdRXG7aaa4WzYD8ibqUgVZU1qbQponAusROXtpJ4W7XJsB2Sq19Em9iGlacFb0YLhLX3fkcuDiuG4hW+wuAdKyoc24u6DHt8T+WeMfeAf447/w/L/dIHH9pMffHX2fOf/DvDXGZN/pdqm67X9vbvF735jo07d41Lp98H8ZDhN8
|
File diff suppressed because one or more lines are too long
File diff suppressed because one or more lines are too long
File diff suppressed because one or more lines are too long
File diff suppressed because one or more lines are too long
File diff suppressed because one or more lines are too long
@ -1 +1 @@
|
||||
eNrVV09v3MYVb3voIcd+ggGRQ1MsVyT3n3YLHwRLcJxIlptdpwligxiSj+RkyRl6Zqj12thD3ZwLbD9BUgtSYNhNkQa5tAZ67KFfwD30s/TNcFd/1kpt5FAkggSS8+a9+b03v/eb0ePTI5CKCf7TZ4xrkDTW+KH++PhUwv0alP7spASdi+TJjb3Jk1qyl2/nWldqtLVFK9ZWJdN5u6A8i3PKeDsW5RbjqTiORDL/x2kONMHwnz29o0C6OxlwvfzGzLZ+bjXf8tp+2+8OvtqJY6i0u8djkTCeLZ9nD1nVIgmkBdVw0piXf6FVVbCYGoxbnyrBn14XnIPFvHw6BahcWrAj+FKCqjAN+P2J0lTX6vExxoV//fO0BKVoBn86fH8N7j8/+cXJbUmzki6/5MKNaZzDt2Y9pVyMrqUo3AP6wKBfPul73t82bDtFIWbuoWQZ48vP/36l9d1mqeUXv7rafmBLbOxff+TuUk0TkbkT3AxwbybLlySK++D1aeTFve0g8ob+9nYUdagXRd1h2uvEL8mVYa9LSLDkjBZqeaxlDX+dsBKru4H5q49c4164ew8qhpVbfuGdrl9fTPK6RTyfvEc58YcDj3jeyP6SGweTZ2Y9XMGdzCt4dXeOMRVYvhhT3SJBQA6oJIEX9IjfH3V6o15gQvx5HWIfeKZzLPEgOP6QyvnypMH3YiM1RCYUXCjp6U6h3fFRvHzZzjvXnFG323F+TUp6LegNA8/zWnnHDYZXGJ58yOjyKfKPZEJkBTy/bvZ+vdDy66qOMJ0WejxwkTPX+p6l9e+QUxKr+O+fff7IWXWPM3K89rA9GDgth3HkHI8hROpmyhk9cqJCRKHSAlkGIXAaFZA4I7MhrU0bJgwYbNzBQAkSQYEO4QEtqwJUWNaFZhWVejPI62dg62FzawgpC7Gv5XxzgpBZGEuwmxcmTK2MKXIHrRWdl7hJm04VZi84LUL0Vq96qc53Za2Ayjh/ZTQXs1DrIqzZekibJgg1AxkmtVyho3Nb1kLwzHQ7+ndxm4271KsBv7toOTMhp6oyAVQsKjAoQ8aPmAZ1hvGh0kmIslUh281OXsaEQSKqESnuN4ohTuQpy8zitYJL1U4qgQJ6lklMCwjrKryv2EPEj0zOQCIszwJdW7nOseSJCgvsS3T2+2tjImY85FBWen7u3UWrCbeebWOdDYTR3CYWeMOB3wu8xeKt71bx8etUHP9wVG2djbqUban7hekE7FY1VxpKt5JYOb1lNFrpH5Ho/+FZvJIdfaVyPbfHgBuvpOAHcDC8qcSfYNFRlJan9RGLheRXS/6m6h77/X7/OPmfcu0buf7/qfFlrf3540dOQ8kwpypHiez4fq+f9FLoDLs9PBAB/EHg9/zI2+4P/CAJgmg7HnjpdurHXs/rpb1ub9AbdrtBF5JOL0CBLSlnKRLX9DPDjv/EOWM7WhtuK3zDEY2P6/i4bQcn2JiGos69llPE2IkoVMgjRIWMQcR1jLKHHtMZlc0RsKIgvn/yRmuNbX8dNF7fd9Em6uuyW81qOd93Gb32GDkfi5pQCQQvC1YnsJcVyzgkRAuyvmCSGeoAoWT8m31izrgIT7n2XX4De5gbT8arWhOrW9iPLWKPJYxJ1Jxrc0FFhSzmBNktUQXIowTZjy8LYg82s5Ks0U3nGK4QYkqoNh8E9QEFWxGR2s9mNuUJGnQtuR2kXM1AIpo7HM9TZcdQ6iVRFcQsZaAMjuY9JrwuI7RhwPUJbBzmmKDKDQ4Raax2i9BihmcWsTJN5qKW51ARWykUZqFFFU4Xa5CIwNQyxnIIia11KYEIYeNHAUcUSxyLoi65Tfs8DxvTFtxUkWfnAFkz4ULhbwEKxgpRKiSiLeyUJrAiKfLiYtbaHJAtIjhuAlVT69PAu4RIkczu6KrWdjNXaeX0CEttlcTg1kIUyoY5+x8EEVuWbEA9NGviftjxCFDSGuc2uWQx/4LI0sr5qijIQKzahpdZ+4zUzb6kDK8z5ywwYA/ujCckEXgZxJLkEE8v7mAEuBJgdSGuLWqm2+RmaqaQDLShM0iJmc1yVlycR5sALcQ3k3gj2aCkNs/MHMZ3+V2+e0huHU5QLKeGoXOye7BPzBkH5lKmyC9v3hrvfTBpkTu3d3cmey2yu7e/Z58fHN4moOP2O7bMl2t5l0+EiSJt6gRvT3WRkJ393+58PL7UN3a/XyGOiagAMDHaBDBctQlg7F1hAaspq9CJKVwHKhyfmJ68sNqqDTCswtKWdN2blr5nfGoAtO3dGqUhPKKS2SEjaqvuR6PtICNba0EKGxagLqVuc444C/y592ZxFovzizVOuLf4Lw/1m1w=
|
||||
eNrVV01v3MYZbnvoobceeh8sCrgplityv/Rh+CBYgutWstRonTqIDWJIDsmJyBl6Zqj12tChbv7A9hcktSAFht0UaZGiaAP02EP/gHrob+kzw119rJXISNpDDRnkzjvvO8/79bzD5ycHTGkuxXdfcWGYorHBD/3b5yeKPa6ZNh8dl8zkMnlxZ3P0olb89Me5MZVeW1qiFe/okpu8U1CRxTnlohPLcomLVB5FMpn8/SRnNIH5j17e10x56xkTZvonu9vpedVkye8EnaC//Nl6HLPKeJsilgkX2fR19pRXbZKwtKCGHTfi6R9oVRU8phbj0odaipe3pRDMYZ6+3Ges8mjBD9iniukKbrDfHGtDTa2fH8Eu++c/TkqmNc3Y73Z+MQf37+/86GTzScWhMv1ylNdt4gfk51SQYHXZJ76/5v7Ine3RZw88i6Pw5ts/8V+8x+n0JVwgmZRZwT5/4G1QQxOZeSPEknl3k+kpiZJuEKU9libdwbAXp92BHyVBN0qX/X7SH0ZfWrNae3DGKOnsS828nzUAp5/89IsHTobweaNJxbydymVpeiKkFjxNfz+XbjGRmXz6Yrjc/WLB6DZ9YjMAme//Zc8oHhuLUSBQynh7LEZyzWR62i6xESG61QsGPez1bxIu4qJO2F4dbcgSadY3SaVYIWly9B5Vk+nxjuIZF6dk4cj1opBj77ZiCbBxWujpkVE1++uV2xob04//dqV02xWhjcWf577OIXu7EjUB5JupoiXzqIC+kUqTG5oV6Q0yL9grivUmkdGHqB9Pq5jcEFKwG8e7imYlnX4qpBfTOGcn6wUOO4inp528d6u11u/3WjdJSW91B6tdxKed97zu6hWCqz05z+oRSoWh5mqGmlsh65UiXb87IEFvrbuyhhfU3KuLeX+z/l/ftgjnJ0w/r+oI8jaZ53Do/3HESzTUQpBdf/4azaEg+9f3Pn7WmtFAa62FlvQ73Va7hUQbG8wQPZjp1tqzVlTIKLSxhe2QCRoVLGmt2Zy2F2U4hsHYXg+GErSEZiZkT2hZFUyHZV0YXlFlFo1cvwMcApYyLKQ8BEGpyeIGqbIwVszFKEy4nglTlB+kFZ2UCOeiUgXvpaBFCG39ppbufZXXmlEV52+s5nIcGlOENZ8vGUsHoeFMhUmtZujoxIW1kCKztAX9PurJqiszWwj6h+3WWKp9XVkDOpYVsyhDLg64YfoM41NtkhAljc7UNpOXMcFIRA2QIt/oDmwUKc/s4bVml6KdVBKT4MyTmBYsrKvwseZPgR/1kzEFWL4DOpcKkyPkiQ4LVBuUg+FcmMixCAUrKzM51+5Das3NdztbZwthNHGOdf3V5WDQ9Q8Pf/DV42jvunGE/1jVS2erHuVL+nFh+wN9pSfasNKrFCJnluyw0eb/anr98Lrh8La0vDjLXsUzq+ZK6vlfsfg7V7P4FUy9OPWOguFw+HVj7xh5BM9NT+oDHksljpKvJeC+JeCr+fO1mwxePOPdbz8rrr0CXDdM/nsj/dJo+P7zZ62mg8Kc6hyM3guCwTAZpKy32h8EKyuMBcvdYBBE/spwOegm3W60Ei/76UoaxP7AH6SD/mB5sNrvd/ss6Q3sYCkp6hLZtfTDQVAftM6aE9KmFTXesGLwuI3HrlscgUdsR7UetVtFDOIAr6I4gQqZAOI6RsVAY39MVTOxZh2D9w/e6qw9RwfbjdY3PbSxep13s13t1jc9xsw11lrvy5pQxQiurY7WQD2aZ4IlxEgyv9iTMWiLULL3yy1iR3KEodx5KO6AcoTV5KKqDXE0iyZvEzdFYZPoiTD2wwCEXkwIOkehEcmzBNyBl0Pi5rA9SdVQMznMFVLuE2rsDwI6w3zRRKbuZ7ObigQCUyvhFlG0Y6aA5r7A+NduDZNJEV2xmKecaYujeY+JqMsIMhicXxiswgQO6tzikJFBtNuEFmOMWOKmCpnIWp1DBbZSanhhZBXuH85BAoGNZYxwSIXWuuRABNj4UbADihDHsqhL4dw+98PZdAG3URTZOUDebLgQ+HsMZDRDlEoFtIXb0hjWJEVdXPTa2HneJlIgCVTvO50G3iVEmmQuo7NYu2TO3MrpAULtiMTiNlIW2pk5+/YDYlclC1B37JnIh1uPwBPjRrlDLknsp58q3YyYBQUViKgtaNmzz4q6yUvKcfs6rwILdvv+3ogkEjdahCRn8f7FDEYMJzFEFyTnUHPTIXdTu4VkzNhyZkrBs3HOi4v7aGOgDXxjsCNbKEljn5m9OzwUD8XGDrm3MwJN79sKnZCN7S1iRzKzd0hNfnL33t7mu6M2ub+7sT7abJONza1N93x3Z5cwE3fecWG+HMuHYiStFeVcJ7js1UVC1rd+tf7+3qW+cfl+o3CsRc0YHKONAVurzgHY3pAOsN7nFZS4xjmswvrI9uSF02ZtALMaoS3pvDdd+Z7VUwOg4z4FQA3hAVXcLVlSm3U/hK6DLG3NCSlsqgC8lHrNHGkd4t+jt7NzeHj+HYANjw7/AxNYKrM=
|
File diff suppressed because one or more lines are too long
File diff suppressed because one or more lines are too long
File diff suppressed because one or more lines are too long
File diff suppressed because one or more lines are too long
File diff suppressed because one or more lines are too long
@ -6,5 +6,5 @@
|
||||
|
||||
- `BaseChatModel` methods `__call__`, `call_as_llm`, `predict`, `predict_messages`. Will be removed in 0.2.0. Use `BaseChatModel.invoke` instead.
|
||||
- `BaseChatModel` methods `apredict`, `apredict_messages`. Will be removed in 0.2.0. Use `BaseChatModel.ainvoke` instead.
|
||||
- `BaseLLM` methods `__call__, `predict`, `predict_messages`. Will be removed in 0.2.0. Use `BaseLLM.invoke` instead.
|
||||
- `BaseLLM` methods `__call__`, `predict`, `predict_messages`. Will be removed in 0.2.0. Use `BaseLLM.invoke` instead.
|
||||
- `BaseLLM` methods `apredict`, `apredict_messages`. Will be removed in 0.2.0. Use `BaseLLM.ainvoke` instead.
|
@ -15,7 +15,10 @@
|
||||
* [Messages](/docs/concepts/messages)
|
||||
:::
|
||||
|
||||
Multimodal support is still relatively new and less common, model providers have not yet standardized on the "best" way to define the API. As such, LangChain's multimodal abstractions are lightweight and flexible, designed to accommodate different model providers' APIs and interaction patterns, but are **not** standardized across models.
|
||||
LangChain supports multimodal data as input to chat models:
|
||||
|
||||
1. Following provider-specific formats
|
||||
2. Adhering to a cross-provider standard (see [how-to guides](/docs/how_to/#multimodal) for detail)
|
||||
|
||||
### How to use multimodal models
|
||||
|
||||
@ -26,38 +29,85 @@ Multimodal support is still relatively new and less common, model providers have
|
||||
|
||||
#### Inputs
|
||||
|
||||
Some models can accept multimodal inputs, such as images, audio, video, or files. The types of multimodal inputs supported depend on the model provider. For instance, [Google's Gemini](/docs/integrations/chat/google_generative_ai/) supports documents like PDFs as inputs.
|
||||
Some models can accept multimodal inputs, such as images, audio, video, or files.
|
||||
The types of multimodal inputs supported depend on the model provider. For instance,
|
||||
[OpenAI](/docs/integrations/chat/openai/),
|
||||
[Anthropic](/docs/integrations/chat/anthropic/), and
|
||||
[Google Gemini](/docs/integrations/chat/google_generative_ai/)
|
||||
support documents like PDFs as inputs.
|
||||
|
||||
Most chat models that support **multimodal inputs** also accept those values in OpenAI's content blocks format. So far this is restricted to image inputs. For models like Gemini which support video and other bytes input, the APIs also support the native, model-specific representations.
|
||||
|
||||
The gist of passing multimodal inputs to a chat model is to use content blocks that specify a type and corresponding data. For example, to pass an image to a chat model:
|
||||
The gist of passing multimodal inputs to a chat model is to use content blocks that
|
||||
specify a type and corresponding data. For example, to pass an image to a chat model
|
||||
as URL:
|
||||
|
||||
```python
|
||||
from langchain_core.messages import HumanMessage
|
||||
|
||||
message = HumanMessage(
|
||||
content=[
|
||||
{"type": "text", "text": "describe the weather in this image"},
|
||||
{"type": "text", "text": "Describe the weather in this image:"},
|
||||
{
|
||||
"type": "image",
|
||||
"source_type": "url",
|
||||
"url": "https://...",
|
||||
},
|
||||
],
|
||||
)
|
||||
response = model.invoke([message])
|
||||
```
|
||||
|
||||
We can also pass the image as in-line data:
|
||||
|
||||
```python
|
||||
from langchain_core.messages import HumanMessage
|
||||
|
||||
message = HumanMessage(
|
||||
content=[
|
||||
{"type": "text", "text": "Describe the weather in this image:"},
|
||||
{
|
||||
"type": "image",
|
||||
"source_type": "base64",
|
||||
"data": "<base64 string>",
|
||||
"mime_type": "image/jpeg",
|
||||
},
|
||||
],
|
||||
)
|
||||
response = model.invoke([message])
|
||||
```
|
||||
|
||||
To pass a PDF file as in-line data (or URL, as supported by providers such as
|
||||
Anthropic), just change `"type"` to `"file"` and `"mime_type"` to `"application/pdf"`.
|
||||
|
||||
See the [how-to guides](/docs/how_to/#multimodal) for more detail.
|
||||
|
||||
Most chat models that support multimodal **image** inputs also accept those values in
|
||||
OpenAI's [Chat Completions format](https://platform.openai.com/docs/guides/images?api-mode=chat):
|
||||
|
||||
```python
|
||||
from langchain_core.messages import HumanMessage
|
||||
|
||||
message = HumanMessage(
|
||||
content=[
|
||||
{"type": "text", "text": "Describe the weather in this image:"},
|
||||
{"type": "image_url", "image_url": {"url": image_url}},
|
||||
],
|
||||
)
|
||||
response = model.invoke([message])
|
||||
```
|
||||
|
||||
:::caution
|
||||
The exact format of the content blocks may vary depending on the model provider. Please refer to the chat model's
|
||||
integration documentation for the correct format. Find the integration in the [chat model integration table](/docs/integrations/chat/).
|
||||
:::
|
||||
Otherwise, chat models will typically accept the native, provider-specific content
|
||||
block format. See [chat model integrations](/docs/integrations/chat/) for detail
|
||||
on specific providers.
|
||||
|
||||
|
||||
#### Outputs
|
||||
|
||||
Virtually no popular chat models support multimodal outputs at the time of writing (October 2024).
|
||||
Some chat models support multimodal outputs, such as images and audio. Multimodal
|
||||
outputs will appear as part of the [AIMessage](/docs/concepts/messages/#aimessage)
|
||||
response object. See for example:
|
||||
|
||||
The only exception is OpenAI's chat model ([gpt-4o-audio-preview](/docs/integrations/chat/openai/)), which can generate audio outputs.
|
||||
|
||||
Multimodal outputs will appear as part of the [AIMessage](/docs/concepts/messages/#aimessage) response object.
|
||||
|
||||
Please see the [ChatOpenAI](/docs/integrations/chat/openai/) for more information on how to use multimodal outputs.
|
||||
- Generating [audio outputs](/docs/integrations/chat/openai/#audio-generation-preview) with OpenAI;
|
||||
- Generating [image outputs](/docs/integrations/chat/google_generative_ai/#multimodal-usage) with Google Gemini.
|
||||
|
||||
#### Tools
|
||||
|
||||
|
@ -92,7 +92,7 @@ structured_model = model.with_structured_output(Questions)
|
||||
|
||||
# Define the system prompt
|
||||
system = """You are a helpful assistant that generates multiple sub-questions related to an input question. \n
|
||||
The goal is to break down the input into a set of sub-problems / sub-questions that can be answers in isolation. \n"""
|
||||
The goal is to break down the input into a set of sub-problems / sub-questions that can be answered independently. \n"""
|
||||
|
||||
# Pass the question to the model
|
||||
question = """What are the main components of an LLM-powered autonomous agent system?"""
|
||||
|
@ -126,7 +126,7 @@ Please see the [Configurable Runnables](#configurable-runnables) section for mor
|
||||
LangChain will automatically try to infer the input and output types of a Runnable based on available information.
|
||||
|
||||
Currently, this inference does not work well for more complex Runnables that are built using [LCEL](/docs/concepts/lcel) composition, and the inferred input and / or output types may be incorrect. In these cases, we recommend that users override the inferred input and output types using the `with_types` method ([API Reference](https://python.langchain.com/api_reference/core/runnables/langchain_core.runnables.base.Runnable.html#langchain_core.runnables.base.Runnable.with_types
|
||||
).
|
||||
)).
|
||||
|
||||
## RunnableConfig
|
||||
|
||||
@ -194,7 +194,7 @@ In Python 3.11 and above, this works out of the box, and you do not need to do a
|
||||
In Python 3.9 and 3.10, if you are using **async code**, you need to manually pass the `RunnableConfig` through to the `Runnable` when invoking it.
|
||||
|
||||
This is due to a limitation in [asyncio's tasks](https://docs.python.org/3/library/asyncio-task.html#asyncio.create_task) in Python 3.9 and 3.10 which did
|
||||
not accept a `context` argument).
|
||||
not accept a `context` argument.
|
||||
|
||||
Propagating the `RunnableConfig` manually is done like so:
|
||||
|
||||
|
@ -66,7 +66,7 @@ This API works with a list of [Document](https://python.langchain.com/api_refere
|
||||
from langchain_core.documents import Document
|
||||
|
||||
document_1 = Document(
|
||||
page_content="I had chocalate chip pancakes and scrambled eggs for breakfast this morning.",
|
||||
page_content="I had chocolate chip pancakes and scrambled eggs for breakfast this morning.",
|
||||
metadata={"source": "tweet"},
|
||||
)
|
||||
|
||||
|
@ -13,23 +13,33 @@ Install `uv`: **[documentation on how to install it](https://docs.astral.sh/uv/g
|
||||
|
||||
This repository contains multiple packages:
|
||||
- `langchain-core`: Base interfaces for key abstractions as well as logic for combining them in chains (LangChain Expression Language).
|
||||
- `langchain-community`: Third-party integrations of various components.
|
||||
- `langchain`: Chains, agents, and retrieval logic that makes up the cognitive architecture of your applications.
|
||||
- `langchain-experimental`: Components and chains that are experimental, either in the sense that the techniques are novel and still being tested, or they require giving the LLM more access than would be possible in most production systems.
|
||||
- Partner integrations: Partner packages in `libs/partners` that are independently version controlled.
|
||||
|
||||
:::note
|
||||
|
||||
Some LangChain packages live outside the monorepo, see for example
|
||||
[langchain-community](https://github.com/langchain-ai/langchain-community) for various
|
||||
third-party integrations and
|
||||
[langchain-experimental](https://github.com/langchain-ai/langchain-experimental) for
|
||||
abstractions that are experimental (either in the sense that the techniques are novel
|
||||
and still being tested, or they require giving the LLM more access than would be
|
||||
possible in most production systems).
|
||||
|
||||
:::
|
||||
|
||||
Each of these has its own development environment. Docs are run from the top-level makefile, but development
|
||||
is split across separate test & release flows.
|
||||
|
||||
For this quickstart, start with langchain-community:
|
||||
For this quickstart, start with `langchain`:
|
||||
|
||||
```bash
|
||||
cd libs/community
|
||||
cd libs/langchain
|
||||
```
|
||||
|
||||
## Local Development Dependencies
|
||||
|
||||
Install langchain-community development requirements (for running langchain, running examples, linting, formatting, tests, and coverage):
|
||||
Install development requirements (for running langchain, running examples, linting, formatting, tests, and coverage):
|
||||
|
||||
```bash
|
||||
uv sync
|
||||
@ -62,22 +72,15 @@ make docker_tests
|
||||
|
||||
There are also [integration tests and code-coverage](../testing.mdx) available.
|
||||
|
||||
### Only develop langchain_core or langchain_community
|
||||
### Developing langchain_core
|
||||
|
||||
If you are only developing `langchain_core` or `langchain_community`, you can simply install the dependencies for the respective projects and run tests:
|
||||
If you are only developing `langchain_core`, you can simply install the dependencies for the project and run tests:
|
||||
|
||||
```bash
|
||||
cd libs/core
|
||||
make test
|
||||
```
|
||||
|
||||
Or:
|
||||
|
||||
```bash
|
||||
cd libs/community
|
||||
make test
|
||||
```
|
||||
|
||||
## Formatting and Linting
|
||||
|
||||
Run these locally before submitting a PR; the CI system will check also.
|
||||
|
@ -83,7 +83,6 @@ LinkedIn, where we highlight the best examples.
|
||||
|
||||
Here are some heuristics for types of content we are excited to promote:
|
||||
|
||||
- **Integration announcement:** Announcements of new integrations with a link to the LangChain documentation page.
|
||||
- **Educational content:** Blogs, YouTube videos and other media showcasing educational content. Note that we prefer content that is NOT framed as "here's how to use integration XYZ", but rather "here's how to do ABC", as we find that is more educational and helpful for developers.
|
||||
- **End-to-end applications:** End-to-end applications are great resources for developers looking to build. We prefer to highlight applications that are more complex/agentic in nature, and that use [LangGraph](https://github.com/langchain-ai/langgraph) as the orchestration framework. We get particularly excited about anything involving long-term memory, human-in-the-loop interaction patterns, or multi-agent architectures.
|
||||
- **Research:** We love highlighting novel research! Whether it is research built on top of LangChain or that integrates with it.
|
||||
|
@ -40,7 +40,7 @@
|
||||
"\n",
|
||||
"To view the list of separators for a given language, pass a value from this enum into\n",
|
||||
"```python\n",
|
||||
"RecursiveCharacterTextSplitter.get_separators_for_language`\n",
|
||||
"RecursiveCharacterTextSplitter.get_separators_for_language\n",
|
||||
"```\n",
|
||||
"\n",
|
||||
"To instantiate a splitter that is tailored for a specific language, pass a value from the enum into\n",
|
||||
|
@ -50,6 +50,7 @@ See [supported integrations](/docs/integrations/chat/) for details on getting st
|
||||
- [How to: force a specific tool call](/docs/how_to/tool_choice)
|
||||
- [How to: work with local models](/docs/how_to/local_llms)
|
||||
- [How to: init any model in one line](/docs/how_to/chat_models_universal_init/)
|
||||
- [How to: pass multimodal data directly to models](/docs/how_to/multimodal_inputs/)
|
||||
|
||||
### Messages
|
||||
|
||||
@ -67,6 +68,7 @@ See [supported integrations](/docs/integrations/chat/) for details on getting st
|
||||
- [How to: use few shot examples in chat models](/docs/how_to/few_shot_examples_chat/)
|
||||
- [How to: partially format prompt templates](/docs/how_to/prompts_partial)
|
||||
- [How to: compose prompts together](/docs/how_to/prompts_composition)
|
||||
- [How to: use multimodal prompts](/docs/how_to/multimodal_prompts/)
|
||||
|
||||
### Example selectors
|
||||
|
||||
@ -170,7 +172,7 @@ Indexing is the process of keeping your vectorstore in-sync with the underlying
|
||||
|
||||
### Tools
|
||||
|
||||
LangChain [Tools](/docs/concepts/tools) contain a description of the tool (to pass to the language model) as well as the implementation of the function to call. Refer [here](/docs/integrations/tools/) for a list of pre-buit tools.
|
||||
LangChain [Tools](/docs/concepts/tools) contain a description of the tool (to pass to the language model) as well as the implementation of the function to call. Refer [here](/docs/integrations/tools/) for a list of pre-built tools.
|
||||
|
||||
- [How to: create tools](/docs/how_to/custom_tools)
|
||||
- [How to: use built-in tools and toolkits](/docs/how_to/tools_builtin)
|
||||
@ -351,7 +353,7 @@ LangSmith allows you to closely trace, monitor and evaluate your LLM application
|
||||
It seamlessly integrates with LangChain and LangGraph, and you can use it to inspect and debug individual steps of your chains and agents as you build.
|
||||
|
||||
LangSmith documentation is hosted on a separate site.
|
||||
You can peruse [LangSmith how-to guides here](https://docs.smith.langchain.com/how_to_guides/), but we'll highlight a few sections that are particularly
|
||||
You can peruse [LangSmith how-to guides here](https://docs.smith.langchain.com/), but we'll highlight a few sections that are particularly
|
||||
relevant to LangChain below:
|
||||
|
||||
### Evaluation
|
||||
|
@ -5,120 +5,165 @@
|
||||
"id": "4facdf7f-680e-4d28-908b-2b8408e2a741",
|
||||
"metadata": {},
|
||||
"source": [
|
||||
"# How to pass multimodal data directly to models\n",
|
||||
"# How to pass multimodal data to models\n",
|
||||
"\n",
|
||||
"Here we demonstrate how to pass [multimodal](/docs/concepts/multimodality/) input directly to models. \n",
|
||||
"We currently expect all input to be passed in the same format as [OpenAI expects](https://platform.openai.com/docs/guides/vision).\n",
|
||||
"For other model providers that support multimodal input, we have added logic inside the class to convert to the expected format.\n",
|
||||
"Here we demonstrate how to pass [multimodal](/docs/concepts/multimodality/) input directly to models.\n",
|
||||
"\n",
|
||||
"In this example we will ask a [model](/docs/concepts/chat_models/#multimodality) to describe an image."
|
||||
"LangChain supports multimodal data as input to chat models:\n",
|
||||
"\n",
|
||||
"1. Following provider-specific formats\n",
|
||||
"2. Adhering to a cross-provider standard\n",
|
||||
"\n",
|
||||
"Below, we demonstrate the cross-provider standard. See [chat model integrations](/docs/integrations/chat/) for detail\n",
|
||||
"on native formats for specific providers.\n",
|
||||
"\n",
|
||||
":::note\n",
|
||||
"\n",
|
||||
"Most chat models that support multimodal **image** inputs also accept those values in\n",
|
||||
"OpenAI's [Chat Completions format](https://platform.openai.com/docs/guides/images?api-mode=chat):\n",
|
||||
"\n",
|
||||
"```python\n",
|
||||
"{\n",
|
||||
" \"type\": \"image_url\",\n",
|
||||
" \"image_url\": {\"url\": image_url},\n",
|
||||
"}\n",
|
||||
"```\n",
|
||||
":::"
|
||||
]
|
||||
},
|
||||
{
|
||||
"cell_type": "markdown",
|
||||
"id": "e30a4ff0-ab38-41a7-858c-a93f99bb2f1b",
|
||||
"metadata": {},
|
||||
"source": [
|
||||
"## Images\n",
|
||||
"\n",
|
||||
"Many providers will accept images passed in-line as base64 data. Some will additionally accept an image from a URL directly.\n",
|
||||
"\n",
|
||||
"### Images from base64 data\n",
|
||||
"\n",
|
||||
"To pass images in-line, format them as content blocks of the following form:\n",
|
||||
"\n",
|
||||
"```python\n",
|
||||
"{\n",
|
||||
" \"type\": \"image\",\n",
|
||||
" \"source_type\": \"base64\",\n",
|
||||
" \"mime_type\": \"image/jpeg\", # or image/png, etc.\n",
|
||||
" \"data\": \"<base64 data string>\",\n",
|
||||
"}\n",
|
||||
"```\n",
|
||||
"\n",
|
||||
"Example:"
|
||||
]
|
||||
},
|
||||
{
|
||||
"cell_type": "code",
|
||||
"execution_count": 1,
|
||||
"id": "0d9fd81a-b7f0-445a-8e3d-cfc2d31fdd59",
|
||||
"execution_count": 10,
|
||||
"id": "1fcf7b27-1cc3-420a-b920-0420b5892e20",
|
||||
"metadata": {},
|
||||
"outputs": [],
|
||||
"outputs": [
|
||||
{
|
||||
"name": "stdout",
|
||||
"output_type": "stream",
|
||||
"text": [
|
||||
"The image shows a beautiful clear day with bright blue skies and wispy cirrus clouds stretching across the horizon. The clouds are thin and streaky, creating elegant patterns against the blue backdrop. The lighting suggests it's during the day, possibly late afternoon given the warm, golden quality of the light on the grass. The weather appears calm with no signs of wind (the grass looks relatively still) and no indication of rain. It's the kind of perfect, mild weather that's ideal for walking along the wooden boardwalk through the marsh grass.\n"
|
||||
]
|
||||
}
|
||||
],
|
||||
"source": [
|
||||
"image_url = \"https://upload.wikimedia.org/wikipedia/commons/thumb/d/dd/Gfp-wisconsin-madison-the-nature-boardwalk.jpg/2560px-Gfp-wisconsin-madison-the-nature-boardwalk.jpg\""
|
||||
"import base64\n",
|
||||
"\n",
|
||||
"import httpx\n",
|
||||
"from langchain.chat_models import init_chat_model\n",
|
||||
"\n",
|
||||
"# Fetch image data\n",
|
||||
"image_url = \"https://upload.wikimedia.org/wikipedia/commons/thumb/d/dd/Gfp-wisconsin-madison-the-nature-boardwalk.jpg/2560px-Gfp-wisconsin-madison-the-nature-boardwalk.jpg\"\n",
|
||||
"image_data = base64.b64encode(httpx.get(image_url).content).decode(\"utf-8\")\n",
|
||||
"\n",
|
||||
"\n",
|
||||
"# Pass to LLM\n",
|
||||
"llm = init_chat_model(\"anthropic:claude-3-5-sonnet-latest\")\n",
|
||||
"\n",
|
||||
"message = {\n",
|
||||
" \"role\": \"user\",\n",
|
||||
" \"content\": [\n",
|
||||
" {\n",
|
||||
" \"type\": \"text\",\n",
|
||||
" \"text\": \"Describe the weather in this image:\",\n",
|
||||
" },\n",
|
||||
" # highlight-start\n",
|
||||
" {\n",
|
||||
" \"type\": \"image\",\n",
|
||||
" \"source_type\": \"base64\",\n",
|
||||
" \"data\": image_data,\n",
|
||||
" \"mime_type\": \"image/jpeg\",\n",
|
||||
" },\n",
|
||||
" # highlight-end\n",
|
||||
" ],\n",
|
||||
"}\n",
|
||||
"response = llm.invoke([message])\n",
|
||||
"print(response.text())"
|
||||
]
|
||||
},
|
||||
{
|
||||
"cell_type": "markdown",
|
||||
"id": "ee2b678a-01dd-40c1-81ff-ddac22be21b7",
|
||||
"metadata": {},
|
||||
"source": [
|
||||
"See [LangSmith trace](https://smith.langchain.com/public/eab05a31-54e8-4fc9-911f-56805da67bef/r) for more detail.\n",
|
||||
"\n",
|
||||
"### Images from a URL\n",
|
||||
"\n",
|
||||
"Some providers (including [OpenAI](/docs/integrations/chat/openai/),\n",
|
||||
"[Anthropic](/docs/integrations/chat/anthropic/), and\n",
|
||||
"[Google Gemini](/docs/integrations/chat/google_generative_ai/)) will also accept images from URLs directly.\n",
|
||||
"\n",
|
||||
"To pass images as URLs, format them as content blocks of the following form:\n",
|
||||
"\n",
|
||||
"```python\n",
|
||||
"{\n",
|
||||
" \"type\": \"image\",\n",
|
||||
" \"source_type\": \"url\",\n",
|
||||
" \"url\": \"https://...\",\n",
|
||||
"}\n",
|
||||
"```\n",
|
||||
"\n",
|
||||
"Example:"
|
||||
]
|
||||
},
|
||||
{
|
||||
"cell_type": "code",
|
||||
"execution_count": 2,
|
||||
"id": "fb896ce9",
|
||||
"metadata": {},
|
||||
"outputs": [],
|
||||
"source": [
|
||||
"from langchain_core.messages import HumanMessage\n",
|
||||
"from langchain_openai import ChatOpenAI\n",
|
||||
"\n",
|
||||
"model = ChatOpenAI(model=\"gpt-4o\")"
|
||||
]
|
||||
},
|
||||
{
|
||||
"cell_type": "markdown",
|
||||
"id": "4fca4da7",
|
||||
"metadata": {},
|
||||
"source": [
|
||||
"The most commonly supported way to pass in images is to pass it in as a byte string.\n",
|
||||
"This should work for most model integrations."
|
||||
]
|
||||
},
|
||||
{
|
||||
"cell_type": "code",
|
||||
"execution_count": 3,
|
||||
"id": "9ca1040c",
|
||||
"metadata": {},
|
||||
"outputs": [],
|
||||
"source": [
|
||||
"import base64\n",
|
||||
"\n",
|
||||
"import httpx\n",
|
||||
"\n",
|
||||
"image_data = base64.b64encode(httpx.get(image_url).content).decode(\"utf-8\")"
|
||||
]
|
||||
},
|
||||
{
|
||||
"cell_type": "code",
|
||||
"execution_count": 4,
|
||||
"id": "ec680b6b",
|
||||
"id": "99d27f8f-ae78-48bc-9bf2-3cef35213ec7",
|
||||
"metadata": {},
|
||||
"outputs": [
|
||||
{
|
||||
"name": "stdout",
|
||||
"output_type": "stream",
|
||||
"text": [
|
||||
"The weather in the image appears to be clear and pleasant. The sky is mostly blue with scattered, light clouds, suggesting a sunny day with minimal cloud cover. There is no indication of rain or strong winds, and the overall scene looks bright and calm. The lush green grass and clear visibility further indicate good weather conditions.\n"
|
||||
"The weather in this image appears to be pleasant and clear. The sky is mostly blue with a few scattered, light clouds, and there is bright sunlight illuminating the green grass and plants. There are no signs of rain or stormy conditions, suggesting it is a calm, likely warm day—typical of spring or summer.\n"
|
||||
]
|
||||
}
|
||||
],
|
||||
"source": [
|
||||
"message = HumanMessage(\n",
|
||||
" content=[\n",
|
||||
" {\"type\": \"text\", \"text\": \"describe the weather in this image\"},\n",
|
||||
"message = {\n",
|
||||
" \"role\": \"user\",\n",
|
||||
" \"content\": [\n",
|
||||
" {\n",
|
||||
" \"type\": \"image_url\",\n",
|
||||
" \"image_url\": {\"url\": f\"data:image/jpeg;base64,{image_data}\"},\n",
|
||||
" \"type\": \"text\",\n",
|
||||
" \"text\": \"Describe the weather in this image:\",\n",
|
||||
" },\n",
|
||||
" {\n",
|
||||
" \"type\": \"image\",\n",
|
||||
" # highlight-start\n",
|
||||
" \"source_type\": \"url\",\n",
|
||||
" \"url\": image_url,\n",
|
||||
" # highlight-end\n",
|
||||
" },\n",
|
||||
" ],\n",
|
||||
")\n",
|
||||
"response = model.invoke([message])\n",
|
||||
"print(response.content)"
|
||||
]
|
||||
},
|
||||
{
|
||||
"cell_type": "markdown",
|
||||
"id": "8656018e-c56d-47d2-b2be-71e87827f90a",
|
||||
"metadata": {},
|
||||
"source": [
|
||||
"We can feed the image URL directly in a content block of type \"image_url\". Note that only some model providers support this."
|
||||
]
|
||||
},
|
||||
{
|
||||
"cell_type": "code",
|
||||
"execution_count": 5,
|
||||
"id": "a8819cf3-5ddc-44f0-889a-19ca7b7fe77e",
|
||||
"metadata": {},
|
||||
"outputs": [
|
||||
{
|
||||
"name": "stdout",
|
||||
"output_type": "stream",
|
||||
"text": [
|
||||
"The weather in the image appears to be clear and sunny. The sky is mostly blue with a few scattered clouds, suggesting good visibility and a likely pleasant temperature. The bright sunlight is casting distinct shadows on the grass and vegetation, indicating it is likely daytime, possibly late morning or early afternoon. The overall ambiance suggests a warm and inviting day, suitable for outdoor activities.\n"
|
||||
]
|
||||
}
|
||||
],
|
||||
"source": [
|
||||
"message = HumanMessage(\n",
|
||||
" content=[\n",
|
||||
" {\"type\": \"text\", \"text\": \"describe the weather in this image\"},\n",
|
||||
" {\"type\": \"image_url\", \"image_url\": {\"url\": image_url}},\n",
|
||||
" ],\n",
|
||||
")\n",
|
||||
"response = model.invoke([message])\n",
|
||||
"print(response.content)"
|
||||
"}\n",
|
||||
"response = llm.invoke([message])\n",
|
||||
"print(response.text())"
|
||||
]
|
||||
},
|
||||
{
|
||||
@ -126,12 +171,12 @@
|
||||
"id": "1c470309",
|
||||
"metadata": {},
|
||||
"source": [
|
||||
"We can also pass in multiple images."
|
||||
"We can also pass in multiple images:"
|
||||
]
|
||||
},
|
||||
{
|
||||
"cell_type": "code",
|
||||
"execution_count": 6,
|
||||
"execution_count": 4,
|
||||
"id": "325fb4ca",
|
||||
"metadata": {},
|
||||
"outputs": [
|
||||
@ -139,20 +184,460 @@
|
||||
"name": "stdout",
|
||||
"output_type": "stream",
|
||||
"text": [
|
||||
"Yes, the two images are the same. They both depict a wooden boardwalk extending through a grassy field under a blue sky with light clouds. The scenery, lighting, and composition are identical.\n"
|
||||
"Yes, these two images are the same. They depict a wooden boardwalk going through a grassy field under a blue sky with some clouds. The colors, composition, and elements in both images are identical.\n"
|
||||
]
|
||||
}
|
||||
],
|
||||
"source": [
|
||||
"message = HumanMessage(\n",
|
||||
" content=[\n",
|
||||
" {\"type\": \"text\", \"text\": \"are these two images the same?\"},\n",
|
||||
" {\"type\": \"image_url\", \"image_url\": {\"url\": image_url}},\n",
|
||||
" {\"type\": \"image_url\", \"image_url\": {\"url\": image_url}},\n",
|
||||
"message = {\n",
|
||||
" \"role\": \"user\",\n",
|
||||
" \"content\": [\n",
|
||||
" {\"type\": \"text\", \"text\": \"Are these two images the same?\"},\n",
|
||||
" {\"type\": \"image\", \"source_type\": \"url\", \"url\": image_url},\n",
|
||||
" {\"type\": \"image\", \"source_type\": \"url\", \"url\": image_url},\n",
|
||||
" ],\n",
|
||||
")\n",
|
||||
"response = model.invoke([message])\n",
|
||||
"print(response.content)"
|
||||
"}\n",
|
||||
"response = llm.invoke([message])\n",
|
||||
"print(response.text())"
|
||||
]
|
||||
},
|
||||
{
|
||||
"cell_type": "markdown",
|
||||
"id": "d72b83e6-8d21-448e-b5df-d5b556c3ccc8",
|
||||
"metadata": {},
|
||||
"source": [
|
||||
"## Documents (PDF)\n",
|
||||
"\n",
|
||||
"Some providers (including [OpenAI](/docs/integrations/chat/openai/),\n",
|
||||
"[Anthropic](/docs/integrations/chat/anthropic/), and\n",
|
||||
"[Google Gemini](/docs/integrations/chat/google_generative_ai/)) will accept PDF documents.\n",
|
||||
"\n",
|
||||
"### Documents from base64 data\n",
|
||||
"\n",
|
||||
"To pass documents in-line, format them as content blocks of the following form:\n",
|
||||
"\n",
|
||||
"```python\n",
|
||||
"{\n",
|
||||
" \"type\": \"file\",\n",
|
||||
" \"source_type\": \"base64\",\n",
|
||||
" \"mime_type\": \"application/pdf\",\n",
|
||||
" \"data\": \"<base64 data string>\",\n",
|
||||
"}\n",
|
||||
"```\n",
|
||||
"\n",
|
||||
"Example:"
|
||||
]
|
||||
},
|
||||
{
|
||||
"cell_type": "code",
|
||||
"execution_count": 3,
|
||||
"id": "6c1455a9-699a-4702-a7e0-7f6eaec76a21",
|
||||
"metadata": {},
|
||||
"outputs": [
|
||||
{
|
||||
"name": "stdout",
|
||||
"output_type": "stream",
|
||||
"text": [
|
||||
"This document appears to be a sample PDF file that contains Lorem ipsum placeholder text. It begins with a title \"Sample PDF\" followed by the subtitle \"This is a simple PDF file. Fun fun fun.\"\n",
|
||||
"\n",
|
||||
"The rest of the document consists of several paragraphs of Lorem ipsum text, which is a commonly used placeholder text in design and publishing. The text is formatted in a clean, readable layout with consistent paragraph spacing. The document appears to be a single page containing four main paragraphs of this placeholder text.\n",
|
||||
"\n",
|
||||
"The Lorem ipsum text, while appearing to be Latin, is actually scrambled Latin-like text that is used primarily to demonstrate the visual form of a document or typeface without the distraction of meaningful content. It's commonly used in publishing and graphic design when the actual content is not yet available but the layout needs to be demonstrated.\n",
|
||||
"\n",
|
||||
"The document has a professional, simple layout with generous margins and clear paragraph separation, making it an effective example of basic PDF formatting and structure.\n"
|
||||
]
|
||||
}
|
||||
],
|
||||
"source": [
|
||||
"import base64\n",
|
||||
"\n",
|
||||
"import httpx\n",
|
||||
"from langchain.chat_models import init_chat_model\n",
|
||||
"\n",
|
||||
"# Fetch PDF data\n",
|
||||
"pdf_url = \"https://pdfobject.com/pdf/sample.pdf\"\n",
|
||||
"pdf_data = base64.b64encode(httpx.get(pdf_url).content).decode(\"utf-8\")\n",
|
||||
"\n",
|
||||
"\n",
|
||||
"# Pass to LLM\n",
|
||||
"llm = init_chat_model(\"anthropic:claude-3-5-sonnet-latest\")\n",
|
||||
"\n",
|
||||
"message = {\n",
|
||||
" \"role\": \"user\",\n",
|
||||
" \"content\": [\n",
|
||||
" {\n",
|
||||
" \"type\": \"text\",\n",
|
||||
" \"text\": \"Describe the document:\",\n",
|
||||
" },\n",
|
||||
" # highlight-start\n",
|
||||
" {\n",
|
||||
" \"type\": \"file\",\n",
|
||||
" \"source_type\": \"base64\",\n",
|
||||
" \"data\": pdf_data,\n",
|
||||
" \"mime_type\": \"application/pdf\",\n",
|
||||
" },\n",
|
||||
" # highlight-end\n",
|
||||
" ],\n",
|
||||
"}\n",
|
||||
"response = llm.invoke([message])\n",
|
||||
"print(response.text())"
|
||||
]
|
||||
},
|
||||
{
|
||||
"cell_type": "markdown",
|
||||
"id": "efb271da-8fdd-41b5-9f29-be6f8c76f49b",
|
||||
"metadata": {},
|
||||
"source": [
|
||||
"### Documents from a URL\n",
|
||||
"\n",
|
||||
"Some providers (specifically [Anthropic](/docs/integrations/chat/anthropic/))\n",
|
||||
"will also accept documents from URLs directly.\n",
|
||||
"\n",
|
||||
"To pass documents as URLs, format them as content blocks of the following form:\n",
|
||||
"\n",
|
||||
"```python\n",
|
||||
"{\n",
|
||||
" \"type\": \"file\",\n",
|
||||
" \"source_type\": \"url\",\n",
|
||||
" \"url\": \"https://...\",\n",
|
||||
"}\n",
|
||||
"```\n",
|
||||
"\n",
|
||||
"Example:"
|
||||
]
|
||||
},
|
||||
{
|
||||
"cell_type": "code",
|
||||
"execution_count": 4,
|
||||
"id": "55e1d937-3b22-4deb-b9f0-9e688f0609dc",
|
||||
"metadata": {},
|
||||
"outputs": [
|
||||
{
|
||||
"name": "stdout",
|
||||
"output_type": "stream",
|
||||
"text": [
|
||||
"This document appears to be a sample PDF file with both text and an image. It begins with a title \"Sample PDF\" followed by the text \"This is a simple PDF file. Fun fun fun.\" The rest of the document contains Lorem ipsum placeholder text arranged in several paragraphs. The content is shown both as text and as an image of the formatted PDF, with the same content displayed in a clean, formatted layout with consistent spacing and typography. The document consists of a single page containing this sample text.\n"
|
||||
]
|
||||
}
|
||||
],
|
||||
"source": [
|
||||
"message = {\n",
|
||||
" \"role\": \"user\",\n",
|
||||
" \"content\": [\n",
|
||||
" {\n",
|
||||
" \"type\": \"text\",\n",
|
||||
" \"text\": \"Describe the document:\",\n",
|
||||
" },\n",
|
||||
" {\n",
|
||||
" \"type\": \"file\",\n",
|
||||
" # highlight-start\n",
|
||||
" \"source_type\": \"url\",\n",
|
||||
" \"url\": pdf_url,\n",
|
||||
" # highlight-end\n",
|
||||
" },\n",
|
||||
" ],\n",
|
||||
"}\n",
|
||||
"response = llm.invoke([message])\n",
|
||||
"print(response.text())"
|
||||
]
|
||||
},
|
||||
{
|
||||
"cell_type": "markdown",
|
||||
"id": "1e661c26-e537-4721-8268-42c0861cb1e6",
|
||||
"metadata": {},
|
||||
"source": [
|
||||
"## Audio\n",
|
||||
"\n",
|
||||
"Some providers (including [OpenAI](/docs/integrations/chat/openai/) and\n",
|
||||
"[Google Gemini](/docs/integrations/chat/google_generative_ai/)) will accept audio inputs.\n",
|
||||
"\n",
|
||||
"### Audio from base64 data\n",
|
||||
"\n",
|
||||
"To pass audio in-line, format them as content blocks of the following form:\n",
|
||||
"\n",
|
||||
"```python\n",
|
||||
"{\n",
|
||||
" \"type\": \"audio\",\n",
|
||||
" \"source_type\": \"base64\",\n",
|
||||
" \"mime_type\": \"audio/wav\", # or appropriate mime-type\n",
|
||||
" \"data\": \"<base64 data string>\",\n",
|
||||
"}\n",
|
||||
"```\n",
|
||||
"\n",
|
||||
"Example:"
|
||||
]
|
||||
},
|
||||
{
|
||||
"cell_type": "code",
|
||||
"execution_count": 2,
|
||||
"id": "a0b91b29-dbd6-4c94-8f24-05471adc7598",
|
||||
"metadata": {},
|
||||
"outputs": [
|
||||
{
|
||||
"name": "stdout",
|
||||
"output_type": "stream",
|
||||
"text": [
|
||||
"The audio appears to consist primarily of bird sounds, specifically bird vocalizations like chirping and possibly other bird songs.\n"
|
||||
]
|
||||
}
|
||||
],
|
||||
"source": [
|
||||
"import base64\n",
|
||||
"\n",
|
||||
"import httpx\n",
|
||||
"from langchain.chat_models import init_chat_model\n",
|
||||
"\n",
|
||||
"# Fetch audio data\n",
|
||||
"audio_url = \"https://upload.wikimedia.org/wikipedia/commons/3/3d/Alcal%C3%A1_de_Henares_%28RPS_13-04-2024%29_canto_de_ruise%C3%B1or_%28Luscinia_megarhynchos%29_en_el_Soto_del_Henares.wav\"\n",
|
||||
"audio_data = base64.b64encode(httpx.get(audio_url).content).decode(\"utf-8\")\n",
|
||||
"\n",
|
||||
"\n",
|
||||
"# Pass to LLM\n",
|
||||
"llm = init_chat_model(\"google_genai:gemini-2.0-flash-001\")\n",
|
||||
"\n",
|
||||
"message = {\n",
|
||||
" \"role\": \"user\",\n",
|
||||
" \"content\": [\n",
|
||||
" {\n",
|
||||
" \"type\": \"text\",\n",
|
||||
" \"text\": \"Describe this audio:\",\n",
|
||||
" },\n",
|
||||
" # highlight-start\n",
|
||||
" {\n",
|
||||
" \"type\": \"audio\",\n",
|
||||
" \"source_type\": \"base64\",\n",
|
||||
" \"data\": audio_data,\n",
|
||||
" \"mime_type\": \"audio/wav\",\n",
|
||||
" },\n",
|
||||
" # highlight-end\n",
|
||||
" ],\n",
|
||||
"}\n",
|
||||
"response = llm.invoke([message])\n",
|
||||
"print(response.text())"
|
||||
]
|
||||
},
|
||||
{
|
||||
"cell_type": "markdown",
|
||||
"id": "92f55a6c-2e4a-4175-8444-8b9aacd6a13e",
|
||||
"metadata": {},
|
||||
"source": [
|
||||
"## Provider-specific parameters\n",
|
||||
"\n",
|
||||
"Some providers will support or require additional fields on content blocks containing multimodal data.\n",
|
||||
"For example, Anthropic lets you specify [caching](/docs/integrations/chat/anthropic/#prompt-caching) of\n",
|
||||
"specific content to reduce token consumption.\n",
|
||||
"\n",
|
||||
"To use these fields, you can:\n",
|
||||
"\n",
|
||||
"1. Store them on directly on the content block; or\n",
|
||||
"2. Use the native format supported by each provider (see [chat model integrations](/docs/integrations/chat/) for detail).\n",
|
||||
"\n",
|
||||
"We show three examples below.\n",
|
||||
"\n",
|
||||
"### Example: Anthropic prompt caching"
|
||||
]
|
||||
},
|
||||
{
|
||||
"cell_type": "code",
|
||||
"execution_count": 2,
|
||||
"id": "83593b9d-a8d3-4c99-9dac-64e0a9d397cb",
|
||||
"metadata": {},
|
||||
"outputs": [
|
||||
{
|
||||
"name": "stdout",
|
||||
"output_type": "stream",
|
||||
"text": [
|
||||
"The image shows a beautiful, clear day with partly cloudy skies. The sky is a vibrant blue with wispy, white cirrus clouds stretching across it. The lighting suggests it's during daylight hours, possibly late afternoon or early evening given the warm, golden quality of the light on the grass. The weather appears calm with no signs of wind (the grass looks relatively still) and no threatening weather conditions. It's the kind of perfect weather you'd want for a walk along this wooden boardwalk through the marshland or grassland area.\n"
|
||||
]
|
||||
},
|
||||
{
|
||||
"data": {
|
||||
"text/plain": [
|
||||
"{'input_tokens': 1586,\n",
|
||||
" 'output_tokens': 117,\n",
|
||||
" 'total_tokens': 1703,\n",
|
||||
" 'input_token_details': {'cache_read': 0, 'cache_creation': 1582}}"
|
||||
]
|
||||
},
|
||||
"execution_count": 2,
|
||||
"metadata": {},
|
||||
"output_type": "execute_result"
|
||||
}
|
||||
],
|
||||
"source": [
|
||||
"llm = init_chat_model(\"anthropic:claude-3-5-sonnet-latest\")\n",
|
||||
"\n",
|
||||
"message = {\n",
|
||||
" \"role\": \"user\",\n",
|
||||
" \"content\": [\n",
|
||||
" {\n",
|
||||
" \"type\": \"text\",\n",
|
||||
" \"text\": \"Describe the weather in this image:\",\n",
|
||||
" },\n",
|
||||
" {\n",
|
||||
" \"type\": \"image\",\n",
|
||||
" \"source_type\": \"url\",\n",
|
||||
" \"url\": image_url,\n",
|
||||
" # highlight-next-line\n",
|
||||
" \"cache_control\": {\"type\": \"ephemeral\"},\n",
|
||||
" },\n",
|
||||
" ],\n",
|
||||
"}\n",
|
||||
"response = llm.invoke([message])\n",
|
||||
"print(response.text())\n",
|
||||
"response.usage_metadata"
|
||||
]
|
||||
},
|
||||
{
|
||||
"cell_type": "code",
|
||||
"execution_count": 3,
|
||||
"id": "9bbf578e-794a-4dc0-a469-78c876ccd4a3",
|
||||
"metadata": {},
|
||||
"outputs": [
|
||||
{
|
||||
"name": "stdout",
|
||||
"output_type": "stream",
|
||||
"text": [
|
||||
"Clear blue skies, wispy clouds.\n"
|
||||
]
|
||||
},
|
||||
{
|
||||
"data": {
|
||||
"text/plain": [
|
||||
"{'input_tokens': 1716,\n",
|
||||
" 'output_tokens': 12,\n",
|
||||
" 'total_tokens': 1728,\n",
|
||||
" 'input_token_details': {'cache_read': 1582, 'cache_creation': 0}}"
|
||||
]
|
||||
},
|
||||
"execution_count": 3,
|
||||
"metadata": {},
|
||||
"output_type": "execute_result"
|
||||
}
|
||||
],
|
||||
"source": [
|
||||
"next_message = {\n",
|
||||
" \"role\": \"user\",\n",
|
||||
" \"content\": [\n",
|
||||
" {\n",
|
||||
" \"type\": \"text\",\n",
|
||||
" \"text\": \"Summarize that in 5 words.\",\n",
|
||||
" }\n",
|
||||
" ],\n",
|
||||
"}\n",
|
||||
"response = llm.invoke([message, response, next_message])\n",
|
||||
"print(response.text())\n",
|
||||
"response.usage_metadata"
|
||||
]
|
||||
},
|
||||
{
|
||||
"cell_type": "markdown",
|
||||
"id": "915b9443-5964-43b8-bb08-691c1ba59065",
|
||||
"metadata": {},
|
||||
"source": [
|
||||
"### Example: Anthropic citations"
|
||||
]
|
||||
},
|
||||
{
|
||||
"cell_type": "code",
|
||||
"execution_count": 4,
|
||||
"id": "ea7707a1-5660-40a1-a10f-0df48a028689",
|
||||
"metadata": {},
|
||||
"outputs": [
|
||||
{
|
||||
"data": {
|
||||
"text/plain": [
|
||||
"[{'citations': [{'cited_text': 'Sample PDF\\r\\nThis is a simple PDF file. Fun fun fun.\\r\\n',\n",
|
||||
" 'document_index': 0,\n",
|
||||
" 'document_title': None,\n",
|
||||
" 'end_page_number': 2,\n",
|
||||
" 'start_page_number': 1,\n",
|
||||
" 'type': 'page_location'}],\n",
|
||||
" 'text': 'Simple PDF file: fun fun',\n",
|
||||
" 'type': 'text'}]"
|
||||
]
|
||||
},
|
||||
"execution_count": 4,
|
||||
"metadata": {},
|
||||
"output_type": "execute_result"
|
||||
}
|
||||
],
|
||||
"source": [
|
||||
"message = {\n",
|
||||
" \"role\": \"user\",\n",
|
||||
" \"content\": [\n",
|
||||
" {\n",
|
||||
" \"type\": \"text\",\n",
|
||||
" \"text\": \"Generate a 5 word summary of this document.\",\n",
|
||||
" },\n",
|
||||
" {\n",
|
||||
" \"type\": \"file\",\n",
|
||||
" \"source_type\": \"base64\",\n",
|
||||
" \"data\": pdf_data,\n",
|
||||
" \"mime_type\": \"application/pdf\",\n",
|
||||
" # highlight-next-line\n",
|
||||
" \"citations\": {\"enabled\": True},\n",
|
||||
" },\n",
|
||||
" ],\n",
|
||||
"}\n",
|
||||
"response = llm.invoke([message])\n",
|
||||
"response.content"
|
||||
]
|
||||
},
|
||||
{
|
||||
"cell_type": "markdown",
|
||||
"id": "e26991eb-e769-41f4-b6e0-63d81f2c7d67",
|
||||
"metadata": {},
|
||||
"source": [
|
||||
"### Example: OpenAI file names\n",
|
||||
"\n",
|
||||
"OpenAI requires that PDF documents be associated with file names:"
|
||||
]
|
||||
},
|
||||
{
|
||||
"cell_type": "code",
|
||||
"execution_count": 5,
|
||||
"id": "ae076c9b-ff8f-461d-9349-250f396c9a25",
|
||||
"metadata": {},
|
||||
"outputs": [
|
||||
{
|
||||
"name": "stdout",
|
||||
"output_type": "stream",
|
||||
"text": [
|
||||
"The document is a sample PDF file containing placeholder text. It consists of one page, titled \"Sample PDF\". The content is a mixture of English and the commonly used filler text \"Lorem ipsum dolor sit amet...\" and its extensions, which are often used in publishing and web design as generic text to demonstrate font, layout, and other visual elements.\n",
|
||||
"\n",
|
||||
"**Key points about the document:**\n",
|
||||
"- Length: 1 page\n",
|
||||
"- Purpose: Demonstrative/sample content\n",
|
||||
"- Content: No substantive or meaningful information, just demonstration text in paragraph form\n",
|
||||
"- Language: English (with the Latin-like \"Lorem Ipsum\" text used for layout purposes)\n",
|
||||
"\n",
|
||||
"There are no charts, tables, diagrams, or images on the page—only plain text. The document serves as an example of what a PDF file looks like rather than providing actual, useful content.\n"
|
||||
]
|
||||
}
|
||||
],
|
||||
"source": [
|
||||
"llm = init_chat_model(\"openai:gpt-4.1\")\n",
|
||||
"\n",
|
||||
"message = {\n",
|
||||
" \"role\": \"user\",\n",
|
||||
" \"content\": [\n",
|
||||
" {\n",
|
||||
" \"type\": \"text\",\n",
|
||||
" \"text\": \"Describe the document:\",\n",
|
||||
" },\n",
|
||||
" {\n",
|
||||
" \"type\": \"file\",\n",
|
||||
" \"source_type\": \"base64\",\n",
|
||||
" \"data\": pdf_data,\n",
|
||||
" \"mime_type\": \"application/pdf\",\n",
|
||||
" # highlight-next-line\n",
|
||||
" \"filename\": \"my-file\",\n",
|
||||
" },\n",
|
||||
" ],\n",
|
||||
"}\n",
|
||||
"response = llm.invoke([message])\n",
|
||||
"print(response.text())"
|
||||
]
|
||||
},
|
||||
{
|
||||
@ -167,16 +652,22 @@
|
||||
},
|
||||
{
|
||||
"cell_type": "code",
|
||||
"execution_count": 8,
|
||||
"id": "cd22ea82-2f93-46f9-9f7a-6aaf479fcaa9",
|
||||
"execution_count": 4,
|
||||
"id": "0f68cce7-350b-4cde-bc40-d3a169551fc3",
|
||||
"metadata": {},
|
||||
"outputs": [
|
||||
{
|
||||
"name": "stdout",
|
||||
"output_type": "stream",
|
||||
"text": [
|
||||
"[{'name': 'weather_tool', 'args': {'weather': 'sunny'}, 'id': 'call_BSX4oq4SKnLlp2WlzDhToHBr'}]\n"
|
||||
]
|
||||
"data": {
|
||||
"text/plain": [
|
||||
"[{'name': 'weather_tool',\n",
|
||||
" 'args': {'weather': 'sunny'},\n",
|
||||
" 'id': 'toolu_01G6JgdkhwggKcQKfhXZQPjf',\n",
|
||||
" 'type': 'tool_call'}]"
|
||||
]
|
||||
},
|
||||
"execution_count": 4,
|
||||
"metadata": {},
|
||||
"output_type": "execute_result"
|
||||
}
|
||||
],
|
||||
"source": [
|
||||
@ -191,16 +682,17 @@
|
||||
" pass\n",
|
||||
"\n",
|
||||
"\n",
|
||||
"model_with_tools = model.bind_tools([weather_tool])\n",
|
||||
"llm_with_tools = llm.bind_tools([weather_tool])\n",
|
||||
"\n",
|
||||
"message = HumanMessage(\n",
|
||||
" content=[\n",
|
||||
" {\"type\": \"text\", \"text\": \"describe the weather in this image\"},\n",
|
||||
" {\"type\": \"image_url\", \"image_url\": {\"url\": image_url}},\n",
|
||||
"message = {\n",
|
||||
" \"role\": \"user\",\n",
|
||||
" \"content\": [\n",
|
||||
" {\"type\": \"text\", \"text\": \"Describe the weather in this image:\"},\n",
|
||||
" {\"type\": \"image\", \"source_type\": \"url\", \"url\": image_url},\n",
|
||||
" ],\n",
|
||||
")\n",
|
||||
"response = model_with_tools.invoke([message])\n",
|
||||
"print(response.tool_calls)"
|
||||
"}\n",
|
||||
"response = llm_with_tools.invoke([message])\n",
|
||||
"response.tool_calls"
|
||||
]
|
||||
}
|
||||
],
|
||||
@ -220,7 +712,7 @@
|
||||
"name": "python",
|
||||
"nbconvert_exporter": "python",
|
||||
"pygments_lexer": "ipython3",
|
||||
"version": "3.9.1"
|
||||
"version": "3.10.4"
|
||||
}
|
||||
},
|
||||
"nbformat": 4,
|
||||
|
@ -9,157 +9,148 @@
|
||||
"\n",
|
||||
"Here we demonstrate how to use prompt templates to format [multimodal](/docs/concepts/multimodality/) inputs to models. \n",
|
||||
"\n",
|
||||
"In this example we will ask a [model](/docs/concepts/chat_models/#multimodality) to describe an image."
|
||||
"To use prompt templates in the context of multimodal data, we can templatize elements of the corresponding content block.\n",
|
||||
"For example, below we define a prompt that takes a URL for an image as a parameter:"
|
||||
]
|
||||
},
|
||||
{
|
||||
"cell_type": "code",
|
||||
"execution_count": 7,
|
||||
"id": "0d9fd81a-b7f0-445a-8e3d-cfc2d31fdd59",
|
||||
"metadata": {},
|
||||
"outputs": [],
|
||||
"source": [
|
||||
"import base64\n",
|
||||
"\n",
|
||||
"import httpx\n",
|
||||
"\n",
|
||||
"image_url = \"https://upload.wikimedia.org/wikipedia/commons/thumb/d/dd/Gfp-wisconsin-madison-the-nature-boardwalk.jpg/2560px-Gfp-wisconsin-madison-the-nature-boardwalk.jpg\"\n",
|
||||
"image_data = base64.b64encode(httpx.get(image_url).content).decode(\"utf-8\")"
|
||||
]
|
||||
},
|
||||
{
|
||||
"cell_type": "code",
|
||||
"execution_count": 6,
|
||||
"execution_count": 1,
|
||||
"id": "2671f995",
|
||||
"metadata": {},
|
||||
"outputs": [],
|
||||
"source": [
|
||||
"from langchain_core.prompts import ChatPromptTemplate\n",
|
||||
"from langchain_openai import ChatOpenAI\n",
|
||||
"\n",
|
||||
"model = ChatOpenAI(model=\"gpt-4o\")"
|
||||
]
|
||||
},
|
||||
{
|
||||
"cell_type": "code",
|
||||
"execution_count": 10,
|
||||
"id": "4ee35e4f",
|
||||
"metadata": {},
|
||||
"outputs": [],
|
||||
"source": [
|
||||
"prompt = ChatPromptTemplate.from_messages(\n",
|
||||
"# Define prompt\n",
|
||||
"prompt = ChatPromptTemplate(\n",
|
||||
" [\n",
|
||||
" (\"system\", \"Describe the image provided\"),\n",
|
||||
" (\n",
|
||||
" \"user\",\n",
|
||||
" [\n",
|
||||
" {\n",
|
||||
" \"role\": \"system\",\n",
|
||||
" \"content\": \"Describe the image provided.\",\n",
|
||||
" },\n",
|
||||
" {\n",
|
||||
" \"role\": \"user\",\n",
|
||||
" \"content\": [\n",
|
||||
" {\n",
|
||||
" \"type\": \"image_url\",\n",
|
||||
" \"image_url\": {\"url\": \"data:image/jpeg;base64,{image_data}\"},\n",
|
||||
" }\n",
|
||||
" \"type\": \"image\",\n",
|
||||
" \"source_type\": \"url\",\n",
|
||||
" # highlight-next-line\n",
|
||||
" \"url\": \"{image_url}\",\n",
|
||||
" },\n",
|
||||
" ],\n",
|
||||
" ),\n",
|
||||
" },\n",
|
||||
" ]\n",
|
||||
")"
|
||||
]
|
||||
},
|
||||
{
|
||||
"cell_type": "code",
|
||||
"execution_count": 11,
|
||||
"id": "089f75c2",
|
||||
"metadata": {},
|
||||
"outputs": [],
|
||||
"source": [
|
||||
"chain = prompt | model"
|
||||
]
|
||||
},
|
||||
{
|
||||
"cell_type": "code",
|
||||
"execution_count": 13,
|
||||
"id": "02744b06",
|
||||
"metadata": {},
|
||||
"outputs": [
|
||||
{
|
||||
"name": "stdout",
|
||||
"output_type": "stream",
|
||||
"text": [
|
||||
"The image depicts a sunny day with a beautiful blue sky filled with scattered white clouds. The sky has varying shades of blue, ranging from a deeper hue near the horizon to a lighter, almost pale blue higher up. The white clouds are fluffy and scattered across the expanse of the sky, creating a peaceful and serene atmosphere. The lighting and cloud patterns suggest pleasant weather conditions, likely during the daytime hours on a mild, sunny day in an outdoor natural setting.\n"
|
||||
]
|
||||
}
|
||||
],
|
||||
"source": [
|
||||
"response = chain.invoke({\"image_data\": image_data})\n",
|
||||
"print(response.content)"
|
||||
]
|
||||
},
|
||||
{
|
||||
"cell_type": "markdown",
|
||||
"id": "e9b9ebf6",
|
||||
"id": "f75d2e26-5b9a-4d5f-94a7-7f98f5666f6d",
|
||||
"metadata": {},
|
||||
"source": [
|
||||
"We can also pass in multiple images."
|
||||
"Let's use this prompt to pass an image to a [chat model](/docs/concepts/chat_models/#multimodality):"
|
||||
]
|
||||
},
|
||||
{
|
||||
"cell_type": "code",
|
||||
"execution_count": 14,
|
||||
"id": "02190ee3",
|
||||
"metadata": {},
|
||||
"outputs": [],
|
||||
"source": [
|
||||
"prompt = ChatPromptTemplate.from_messages(\n",
|
||||
" [\n",
|
||||
" (\"system\", \"compare the two pictures provided\"),\n",
|
||||
" (\n",
|
||||
" \"user\",\n",
|
||||
" [\n",
|
||||
" {\n",
|
||||
" \"type\": \"image_url\",\n",
|
||||
" \"image_url\": {\"url\": \"data:image/jpeg;base64,{image_data1}\"},\n",
|
||||
" },\n",
|
||||
" {\n",
|
||||
" \"type\": \"image_url\",\n",
|
||||
" \"image_url\": {\"url\": \"data:image/jpeg;base64,{image_data2}\"},\n",
|
||||
" },\n",
|
||||
" ],\n",
|
||||
" ),\n",
|
||||
" ]\n",
|
||||
")"
|
||||
]
|
||||
},
|
||||
{
|
||||
"cell_type": "code",
|
||||
"execution_count": 15,
|
||||
"id": "42af057b",
|
||||
"metadata": {},
|
||||
"outputs": [],
|
||||
"source": [
|
||||
"chain = prompt | model"
|
||||
]
|
||||
},
|
||||
{
|
||||
"cell_type": "code",
|
||||
"execution_count": 16,
|
||||
"id": "513abe00",
|
||||
"execution_count": 2,
|
||||
"id": "5df2e558-321d-4cf7-994e-2815ac37e704",
|
||||
"metadata": {},
|
||||
"outputs": [
|
||||
{
|
||||
"name": "stdout",
|
||||
"output_type": "stream",
|
||||
"text": [
|
||||
"The two images provided are identical. Both images feature a wooden boardwalk path extending through a lush green field under a bright blue sky with some clouds. The perspective, colors, and elements in both images are exactly the same.\n"
|
||||
"This image shows a beautiful wooden boardwalk cutting through a lush green wetland or marsh area. The boardwalk extends straight ahead toward the horizon, creating a strong leading line through the composition. On either side, tall green grasses sway in what appears to be a summer or late spring setting. The sky is particularly striking, with wispy cirrus clouds streaking across a vibrant blue background. In the distance, you can see a tree line bordering the wetland area. The lighting suggests this may be during \"golden hour\" - either early morning or late afternoon - as there's a warm, gentle quality to the light that's illuminating the scene. The wooden planks of the boardwalk appear well-maintained and provide safe passage through what would otherwise be difficult terrain to traverse. It's the kind of scene you might find in a nature preserve or wildlife refuge designed to give visitors access to observe wetland ecosystems while protecting the natural environment.\n"
|
||||
]
|
||||
}
|
||||
],
|
||||
"source": [
|
||||
"response = chain.invoke({\"image_data1\": image_data, \"image_data2\": image_data})\n",
|
||||
"print(response.content)"
|
||||
"from langchain.chat_models import init_chat_model\n",
|
||||
"\n",
|
||||
"llm = init_chat_model(\"anthropic:claude-3-5-sonnet-latest\")\n",
|
||||
"\n",
|
||||
"url = \"https://upload.wikimedia.org/wikipedia/commons/thumb/d/dd/Gfp-wisconsin-madison-the-nature-boardwalk.jpg/2560px-Gfp-wisconsin-madison-the-nature-boardwalk.jpg\"\n",
|
||||
"\n",
|
||||
"chain = prompt | llm\n",
|
||||
"response = chain.invoke({\"image_url\": url})\n",
|
||||
"print(response.text())"
|
||||
]
|
||||
},
|
||||
{
|
||||
"cell_type": "markdown",
|
||||
"id": "f4cfdc50-4a9f-4888-93b4-af697366b0f3",
|
||||
"metadata": {},
|
||||
"source": [
|
||||
"Note that we can templatize arbitrary elements of the content block:"
|
||||
]
|
||||
},
|
||||
{
|
||||
"cell_type": "code",
|
||||
"execution_count": 3,
|
||||
"id": "53c88ebb-dd57-40c8-8542-b2c916706653",
|
||||
"metadata": {},
|
||||
"outputs": [],
|
||||
"source": [
|
||||
"prompt = ChatPromptTemplate(\n",
|
||||
" [\n",
|
||||
" {\n",
|
||||
" \"role\": \"system\",\n",
|
||||
" \"content\": \"Describe the image provided.\",\n",
|
||||
" },\n",
|
||||
" {\n",
|
||||
" \"role\": \"user\",\n",
|
||||
" \"content\": [\n",
|
||||
" {\n",
|
||||
" \"type\": \"image\",\n",
|
||||
" \"source_type\": \"base64\",\n",
|
||||
" \"mime_type\": \"{image_mime_type}\",\n",
|
||||
" \"data\": \"{image_data}\",\n",
|
||||
" \"cache_control\": {\"type\": \"{cache_type}\"},\n",
|
||||
" },\n",
|
||||
" ],\n",
|
||||
" },\n",
|
||||
" ]\n",
|
||||
")"
|
||||
]
|
||||
},
|
||||
{
|
||||
"cell_type": "code",
|
||||
"execution_count": 4,
|
||||
"id": "25e4829e-0073-49a8-9669-9f43e5778383",
|
||||
"metadata": {},
|
||||
"outputs": [
|
||||
{
|
||||
"name": "stdout",
|
||||
"output_type": "stream",
|
||||
"text": [
|
||||
"This image shows a beautiful wooden boardwalk cutting through a lush green marsh or wetland area. The boardwalk extends straight ahead toward the horizon, creating a strong leading line in the composition. The surrounding vegetation consists of tall grass and reeds in vibrant green hues, with some bushes and trees visible in the background. The sky is particularly striking, featuring a bright blue color with wispy white clouds streaked across it. The lighting suggests this photo was taken during the \"golden hour\" - either early morning or late afternoon - giving the scene a warm, peaceful quality. The raised wooden path provides accessible access through what would otherwise be difficult terrain to traverse, allowing visitors to experience and appreciate this natural environment.\n"
|
||||
]
|
||||
}
|
||||
],
|
||||
"source": [
|
||||
"import base64\n",
|
||||
"\n",
|
||||
"import httpx\n",
|
||||
"\n",
|
||||
"image_data = base64.b64encode(httpx.get(url).content).decode(\"utf-8\")\n",
|
||||
"\n",
|
||||
"chain = prompt | llm\n",
|
||||
"response = chain.invoke(\n",
|
||||
" {\n",
|
||||
" \"image_data\": image_data,\n",
|
||||
" \"image_mime_type\": \"image/jpeg\",\n",
|
||||
" \"cache_type\": \"ephemeral\",\n",
|
||||
" }\n",
|
||||
")\n",
|
||||
"print(response.text())"
|
||||
]
|
||||
},
|
||||
{
|
||||
"cell_type": "code",
|
||||
"execution_count": null,
|
||||
"id": "ea8152c3",
|
||||
"id": "424defe8-d85c-4e45-a88d-bf6f910d5ebb",
|
||||
"metadata": {},
|
||||
"outputs": [],
|
||||
"source": []
|
||||
@ -181,7 +172,7 @@
|
||||
"name": "python",
|
||||
"nbconvert_exporter": "python",
|
||||
"pygments_lexer": "ipython3",
|
||||
"version": "3.10.1"
|
||||
"version": "3.10.4"
|
||||
}
|
||||
},
|
||||
"nbformat": 4,
|
||||
|
@ -15,7 +15,7 @@
|
||||
"\n",
|
||||
"To build a production application, you will need to do more work to keep track of application state appropriately.\n",
|
||||
"\n",
|
||||
"We recommend using `langgraph` for powering such a capability. For more details, please see this [guide](https://langchain-ai.github.io/langgraph/how-tos/human-in-the-loop/).\n",
|
||||
"We recommend using `langgraph` for powering such a capability. For more details, please see this [guide](https://langchain-ai.github.io/langgraph/concepts/human_in_the_loop/).\n",
|
||||
":::\n"
|
||||
]
|
||||
},
|
||||
@ -209,7 +209,7 @@
|
||||
"metadata": {},
|
||||
"outputs": [
|
||||
{
|
||||
"name": "stdin",
|
||||
"name": "stdout",
|
||||
"output_type": "stream",
|
||||
"text": [
|
||||
"Do you approve of the following tool invocations\n",
|
||||
@ -252,7 +252,7 @@
|
||||
"metadata": {},
|
||||
"outputs": [
|
||||
{
|
||||
"name": "stdin",
|
||||
"name": "stdout",
|
||||
"output_type": "stream",
|
||||
"text": [
|
||||
"Do you approve of the following tool invocations\n",
|
||||
|
118
docs/docs/integrations/caches/singlestore_semantic_cache.ipynb
Normal file
118
docs/docs/integrations/caches/singlestore_semantic_cache.ipynb
Normal file
@ -0,0 +1,118 @@
|
||||
{
|
||||
"cells": [
|
||||
{
|
||||
"cell_type": "markdown",
|
||||
"id": "e49f1e0d",
|
||||
"metadata": {},
|
||||
"source": [
|
||||
"# SingleStoreSemanticCache\n",
|
||||
"\n",
|
||||
"This example demonstrates how to get started with the SingleStore semantic cache.\n",
|
||||
"\n",
|
||||
"### Integration Overview\n",
|
||||
"\n",
|
||||
"`SingleStoreSemanticCache` leverages `SingleStoreVectorStore` to cache LLM responses directly in a SingleStore database, enabling efficient semantic retrieval and reuse of results.\n",
|
||||
"\n",
|
||||
"### Integration details\n",
|
||||
"\n",
|
||||
"\n",
|
||||
"\n",
|
||||
"| Class | Package | JS support |\n",
|
||||
"| :--- | :--- | :---: |\n",
|
||||
"| SingleStoreSemanticCache | langchain_singlestore | ❌ | "
|
||||
]
|
||||
},
|
||||
{
|
||||
"cell_type": "markdown",
|
||||
"id": "0730d6a1-c893-4840-9817-5e5251676d5d",
|
||||
"metadata": {},
|
||||
"source": [
|
||||
"## Installation\n",
|
||||
"\n",
|
||||
"This cache lives in the `langchain-singlestore` package:"
|
||||
]
|
||||
},
|
||||
{
|
||||
"cell_type": "code",
|
||||
"execution_count": null,
|
||||
"id": "652d6238-1f87-422a-b135-f5abbb8652fc",
|
||||
"metadata": {},
|
||||
"outputs": [],
|
||||
"source": [
|
||||
"%pip install -qU langchain-singlestore"
|
||||
]
|
||||
},
|
||||
{
|
||||
"cell_type": "markdown",
|
||||
"id": "5c5f2839-4020-424e-9fc9-07777eede442",
|
||||
"metadata": {},
|
||||
"source": [
|
||||
"## Usage"
|
||||
]
|
||||
},
|
||||
{
|
||||
"cell_type": "code",
|
||||
"execution_count": null,
|
||||
"id": "51a60dbe-9f2e-4e04-bb62-23968f17164a",
|
||||
"metadata": {},
|
||||
"outputs": [],
|
||||
"source": [
|
||||
"from langchain_core.globals import set_llm_cache\n",
|
||||
"from langchain_singlestore import SingleStoreSemanticCache\n",
|
||||
"\n",
|
||||
"set_llm_cache(\n",
|
||||
" SingleStoreSemanticCache(\n",
|
||||
" embedding=YourEmbeddings(),\n",
|
||||
" host=\"root:pass@localhost:3306/db\",\n",
|
||||
" )\n",
|
||||
")"
|
||||
]
|
||||
},
|
||||
{
|
||||
"cell_type": "code",
|
||||
"execution_count": null,
|
||||
"id": "cddda8ef",
|
||||
"metadata": {},
|
||||
"outputs": [],
|
||||
"source": [
|
||||
"%%time\n",
|
||||
"# The first time, it is not yet in cache, so it should take longer\n",
|
||||
"llm.invoke(\"Tell me a joke\")"
|
||||
]
|
||||
},
|
||||
{
|
||||
"cell_type": "code",
|
||||
"execution_count": null,
|
||||
"id": "c474168f",
|
||||
"metadata": {},
|
||||
"outputs": [],
|
||||
"source": [
|
||||
"%%time\n",
|
||||
"# The second time, while not a direct hit, the question is semantically similar to the original question,\n",
|
||||
"# so it uses the cached result!\n",
|
||||
"llm.invoke(\"Tell me one joke\")"
|
||||
]
|
||||
}
|
||||
],
|
||||
"metadata": {
|
||||
"kernelspec": {
|
||||
"display_name": "langchain-singlestore-BD1RbQ07-py3.11",
|
||||
"language": "python",
|
||||
"name": "python3"
|
||||
},
|
||||
"language_info": {
|
||||
"codemirror_mode": {
|
||||
"name": "ipython",
|
||||
"version": 3
|
||||
},
|
||||
"file_extension": ".py",
|
||||
"mimetype": "text/x-python",
|
||||
"name": "python",
|
||||
"nbconvert_exporter": "python",
|
||||
"pygments_lexer": "ipython3",
|
||||
"version": "3.11.11"
|
||||
}
|
||||
},
|
||||
"nbformat": 4,
|
||||
"nbformat_minor": 5
|
||||
}
|
@ -1,378 +1,401 @@
|
||||
{
|
||||
"cells": [
|
||||
{
|
||||
"cell_type": "raw",
|
||||
"id": "afaf8039",
|
||||
"metadata": {},
|
||||
"source": [
|
||||
"---\n",
|
||||
"sidebar_label: AWS Bedrock\n",
|
||||
"---"
|
||||
]
|
||||
},
|
||||
{
|
||||
"cell_type": "markdown",
|
||||
"id": "e49f1e0d",
|
||||
"metadata": {},
|
||||
"source": [
|
||||
"# ChatBedrock\n",
|
||||
"\n",
|
||||
"This doc will help you get started with AWS Bedrock [chat models](/docs/concepts/chat_models). Amazon Bedrock is a fully managed service that offers a choice of high-performing foundation models (FMs) from leading AI companies like AI21 Labs, Anthropic, Cohere, Meta, Stability AI, and Amazon via a single API, along with a broad set of capabilities you need to build generative AI applications with security, privacy, and responsible AI. Using Amazon Bedrock, you can easily experiment with and evaluate top FMs for your use case, privately customize them with your data using techniques such as fine-tuning and Retrieval Augmented Generation (RAG), and build agents that execute tasks using your enterprise systems and data sources. Since Amazon Bedrock is serverless, you don't have to manage any infrastructure, and you can securely integrate and deploy generative AI capabilities into your applications using the AWS services you are already familiar with.\n",
|
||||
"\n",
|
||||
"For more information on which models are accessible via Bedrock, head to the [AWS docs](https://docs.aws.amazon.com/bedrock/latest/userguide/models-features.html).\n",
|
||||
"\n",
|
||||
"For detailed documentation of all ChatBedrock features and configurations head to the [API reference](https://python.langchain.com/api_reference/aws/chat_models/langchain_aws.chat_models.bedrock.ChatBedrock.html).\n",
|
||||
"\n",
|
||||
"## Overview\n",
|
||||
"### Integration details\n",
|
||||
"\n",
|
||||
"| Class | Package | Local | Serializable | [JS support](https://js.langchain.com/docs/integrations/chat/bedrock) | Package downloads | Package latest |\n",
|
||||
"| :--- | :--- | :---: | :---: | :---: | :---: | :---: |\n",
|
||||
"| [ChatBedrock](https://python.langchain.com/api_reference/aws/chat_models/langchain_aws.chat_models.bedrock.ChatBedrock.html) | [langchain-aws](https://python.langchain.com/api_reference/aws/index.html) | ❌ | beta | ✅ |  |  |\n",
|
||||
"\n",
|
||||
"### Model features\n",
|
||||
"| [Tool calling](/docs/how_to/tool_calling) | [Structured output](/docs/how_to/structured_output/) | JSON mode | [Image input](/docs/how_to/multimodal_inputs/) | Audio input | Video input | [Token-level streaming](/docs/how_to/chat_streaming/) | Native async | [Token usage](/docs/how_to/chat_token_usage_tracking/) | [Logprobs](/docs/how_to/logprobs/) |\n",
|
||||
"| :---: | :---: | :---: | :---: | :---: | :---: | :---: | :---: | :---: | :---: |\n",
|
||||
"| ✅ | ✅ | ❌ | ✅ | ❌ | ❌ | ✅ | ❌ | ✅ | ❌ |\n",
|
||||
"\n",
|
||||
"## Setup\n",
|
||||
"\n",
|
||||
"To access Bedrock models you'll need to create an AWS account, set up the Bedrock API service, get an access key ID and secret key, and install the `langchain-aws` integration package.\n",
|
||||
"\n",
|
||||
"### Credentials\n",
|
||||
"\n",
|
||||
"Head to the [AWS docs](https://docs.aws.amazon.com/bedrock/latest/userguide/setting-up.html) to sign up to AWS and setup your credentials. You'll also need to turn on model access for your account, which you can do by following [these instructions](https://docs.aws.amazon.com/bedrock/latest/userguide/model-access.html)."
|
||||
]
|
||||
},
|
||||
{
|
||||
"cell_type": "markdown",
|
||||
"id": "72ee0c4b-9764-423a-9dbf-95129e185210",
|
||||
"metadata": {},
|
||||
"source": "To enable automated tracing of your model calls, set your [LangSmith](https://docs.smith.langchain.com/) API key:"
|
||||
},
|
||||
{
|
||||
"cell_type": "code",
|
||||
"execution_count": null,
|
||||
"id": "a15d341e-3e26-4ca3-830b-5aab30ed66de",
|
||||
"metadata": {},
|
||||
"outputs": [],
|
||||
"source": [
|
||||
"# os.environ[\"LANGSMITH_API_KEY\"] = getpass.getpass(\"Enter your LangSmith API key: \")\n",
|
||||
"# os.environ[\"LANGSMITH_TRACING\"] = \"true\""
|
||||
]
|
||||
},
|
||||
{
|
||||
"cell_type": "markdown",
|
||||
"id": "0730d6a1-c893-4840-9817-5e5251676d5d",
|
||||
"metadata": {},
|
||||
"source": [
|
||||
"### Installation\n",
|
||||
"\n",
|
||||
"The LangChain Bedrock integration lives in the `langchain-aws` package:"
|
||||
]
|
||||
},
|
||||
{
|
||||
"cell_type": "code",
|
||||
"execution_count": null,
|
||||
"id": "652d6238-1f87-422a-b135-f5abbb8652fc",
|
||||
"metadata": {},
|
||||
"outputs": [],
|
||||
"source": [
|
||||
"%pip install -qU langchain-aws"
|
||||
]
|
||||
},
|
||||
{
|
||||
"cell_type": "markdown",
|
||||
"id": "a38cde65-254d-4219-a441-068766c0d4b5",
|
||||
"metadata": {},
|
||||
"source": [
|
||||
"## Instantiation\n",
|
||||
"\n",
|
||||
"Now we can instantiate our model object and generate chat completions:"
|
||||
]
|
||||
},
|
||||
{
|
||||
"cell_type": "code",
|
||||
"execution_count": 1,
|
||||
"id": "cb09c344-1836-4e0c-acf8-11d13ac1dbae",
|
||||
"metadata": {},
|
||||
"outputs": [],
|
||||
"source": [
|
||||
"from langchain_aws import ChatBedrock\n",
|
||||
"\n",
|
||||
"llm = ChatBedrock(\n",
|
||||
" model_id=\"anthropic.claude-3-sonnet-20240229-v1:0\",\n",
|
||||
" model_kwargs=dict(temperature=0),\n",
|
||||
" # other params...\n",
|
||||
")"
|
||||
]
|
||||
},
|
||||
{
|
||||
"cell_type": "markdown",
|
||||
"id": "2b4f3e15",
|
||||
"metadata": {},
|
||||
"source": [
|
||||
"## Invocation"
|
||||
]
|
||||
},
|
||||
{
|
||||
"cell_type": "code",
|
||||
"execution_count": 5,
|
||||
"id": "62e0dbc3",
|
||||
"metadata": {
|
||||
"tags": []
|
||||
},
|
||||
"outputs": [
|
||||
{
|
||||
"data": {
|
||||
"text/plain": [
|
||||
"AIMessage(content=\"Voici la traduction en français :\\n\\nJ'aime la programmation.\", additional_kwargs={'usage': {'prompt_tokens': 29, 'completion_tokens': 21, 'total_tokens': 50}, 'stop_reason': 'end_turn', 'model_id': 'anthropic.claude-3-sonnet-20240229-v1:0'}, response_metadata={'usage': {'prompt_tokens': 29, 'completion_tokens': 21, 'total_tokens': 50}, 'stop_reason': 'end_turn', 'model_id': 'anthropic.claude-3-sonnet-20240229-v1:0'}, id='run-fdb07dc3-ff72-430d-b22b-e7824b15c766-0', usage_metadata={'input_tokens': 29, 'output_tokens': 21, 'total_tokens': 50})"
|
||||
]
|
||||
},
|
||||
"execution_count": 5,
|
||||
"metadata": {},
|
||||
"output_type": "execute_result"
|
||||
}
|
||||
],
|
||||
"source": [
|
||||
"messages = [\n",
|
||||
" (\n",
|
||||
" \"system\",\n",
|
||||
" \"You are a helpful assistant that translates English to French. Translate the user sentence.\",\n",
|
||||
" ),\n",
|
||||
" (\"human\", \"I love programming.\"),\n",
|
||||
"]\n",
|
||||
"ai_msg = llm.invoke(messages)\n",
|
||||
"ai_msg"
|
||||
]
|
||||
},
|
||||
{
|
||||
"cell_type": "code",
|
||||
"execution_count": 6,
|
||||
"id": "d86145b3-bfef-46e8-b227-4dda5c9c2705",
|
||||
"metadata": {},
|
||||
"outputs": [
|
||||
{
|
||||
"name": "stdout",
|
||||
"output_type": "stream",
|
||||
"text": [
|
||||
"Voici la traduction en français :\n",
|
||||
"\n",
|
||||
"J'aime la programmation.\n"
|
||||
]
|
||||
}
|
||||
],
|
||||
"source": [
|
||||
"print(ai_msg.content)"
|
||||
]
|
||||
},
|
||||
{
|
||||
"cell_type": "markdown",
|
||||
"id": "18e2bfc0-7e78-4528-a73f-499ac150dca8",
|
||||
"metadata": {},
|
||||
"source": [
|
||||
"## Chaining\n",
|
||||
"\n",
|
||||
"We can [chain](/docs/how_to/sequence/) our model with a prompt template like so:"
|
||||
]
|
||||
},
|
||||
{
|
||||
"cell_type": "code",
|
||||
"execution_count": 7,
|
||||
"id": "e197d1d7-a070-4c96-9f8a-a0e86d046e0b",
|
||||
"metadata": {},
|
||||
"outputs": [
|
||||
{
|
||||
"data": {
|
||||
"text/plain": [
|
||||
"AIMessage(content='Ich liebe Programmieren.', additional_kwargs={'usage': {'prompt_tokens': 23, 'completion_tokens': 11, 'total_tokens': 34}, 'stop_reason': 'end_turn', 'model_id': 'anthropic.claude-3-sonnet-20240229-v1:0'}, response_metadata={'usage': {'prompt_tokens': 23, 'completion_tokens': 11, 'total_tokens': 34}, 'stop_reason': 'end_turn', 'model_id': 'anthropic.claude-3-sonnet-20240229-v1:0'}, id='run-5ad005ce-9f31-4670-baa0-9373d418698a-0', usage_metadata={'input_tokens': 23, 'output_tokens': 11, 'total_tokens': 34})"
|
||||
]
|
||||
},
|
||||
"execution_count": 7,
|
||||
"metadata": {},
|
||||
"output_type": "execute_result"
|
||||
}
|
||||
],
|
||||
"source": [
|
||||
"from langchain_core.prompts import ChatPromptTemplate\n",
|
||||
"\n",
|
||||
"prompt = ChatPromptTemplate.from_messages(\n",
|
||||
" [\n",
|
||||
" (\n",
|
||||
" \"system\",\n",
|
||||
" \"You are a helpful assistant that translates {input_language} to {output_language}.\",\n",
|
||||
" ),\n",
|
||||
" (\"human\", \"{input}\"),\n",
|
||||
" ]\n",
|
||||
")\n",
|
||||
"\n",
|
||||
"chain = prompt | llm\n",
|
||||
"chain.invoke(\n",
|
||||
" {\n",
|
||||
" \"input_language\": \"English\",\n",
|
||||
" \"output_language\": \"German\",\n",
|
||||
" \"input\": \"I love programming.\",\n",
|
||||
" }\n",
|
||||
")"
|
||||
]
|
||||
},
|
||||
{
|
||||
"cell_type": "markdown",
|
||||
"id": "d1ee55bc-ffc8-4cfa-801c-993953a08cfd",
|
||||
"metadata": {},
|
||||
"source": [
|
||||
"## Bedrock Converse API\n",
|
||||
"\n",
|
||||
"AWS has recently released the Bedrock Converse API which provides a unified conversational interface for Bedrock models. This API does not yet support custom models. You can see a list of all [models that are supported here](https://docs.aws.amazon.com/bedrock/latest/userguide/conversation-inference.html). To improve reliability the ChatBedrock integration will switch to using the Bedrock Converse API as soon as it has feature parity with the existing Bedrock API. Until then a separate [ChatBedrockConverse](https://python.langchain.com/api_reference/aws/chat_models/langchain_aws.chat_models.bedrock_converse.ChatBedrockConverse.html) integration has been released.\n",
|
||||
"\n",
|
||||
"We recommend using `ChatBedrockConverse` for users who do not need to use custom models.\n",
|
||||
"\n",
|
||||
"You can use it like so:"
|
||||
]
|
||||
},
|
||||
{
|
||||
"cell_type": "code",
|
||||
"execution_count": 3,
|
||||
"id": "ae728e59-94d4-40cf-9d24-25ad8723fc59",
|
||||
"metadata": {},
|
||||
"outputs": [
|
||||
{
|
||||
"data": {
|
||||
"text/plain": [
|
||||
"AIMessage(content=\"Voici la traduction en français :\\n\\nJ'aime la programmation.\", response_metadata={'ResponseMetadata': {'RequestId': '4fcbfbe9-f916-4df2-b0bd-ea1147b550aa', 'HTTPStatusCode': 200, 'HTTPHeaders': {'date': 'Wed, 21 Aug 2024 17:23:49 GMT', 'content-type': 'application/json', 'content-length': '243', 'connection': 'keep-alive', 'x-amzn-requestid': '4fcbfbe9-f916-4df2-b0bd-ea1147b550aa'}, 'RetryAttempts': 0}, 'stopReason': 'end_turn', 'metrics': {'latencyMs': 672}}, id='run-77ee9810-e32b-45dc-9ccb-6692253b1f45-0', usage_metadata={'input_tokens': 29, 'output_tokens': 21, 'total_tokens': 50})"
|
||||
]
|
||||
},
|
||||
"execution_count": 3,
|
||||
"metadata": {},
|
||||
"output_type": "execute_result"
|
||||
}
|
||||
],
|
||||
"source": [
|
||||
"from langchain_aws import ChatBedrockConverse\n",
|
||||
"\n",
|
||||
"llm = ChatBedrockConverse(\n",
|
||||
" model=\"anthropic.claude-3-sonnet-20240229-v1:0\",\n",
|
||||
" temperature=0,\n",
|
||||
" max_tokens=None,\n",
|
||||
" # other params...\n",
|
||||
")\n",
|
||||
"\n",
|
||||
"llm.invoke(messages)"
|
||||
]
|
||||
},
|
||||
{
|
||||
"cell_type": "markdown",
|
||||
"id": "4da16f3e-e80b-48c0-8036-c1cc5f7c8c05",
|
||||
"metadata": {},
|
||||
"source": [
|
||||
"### Streaming\n",
|
||||
"\n",
|
||||
"Note that `ChatBedrockConverse` emits content blocks while streaming:"
|
||||
]
|
||||
},
|
||||
{
|
||||
"cell_type": "code",
|
||||
"execution_count": 6,
|
||||
"id": "7794b32e-d8de-4973-bf0f-39807dc745f0",
|
||||
"metadata": {},
|
||||
"outputs": [
|
||||
{
|
||||
"name": "stdout",
|
||||
"output_type": "stream",
|
||||
"text": [
|
||||
"content=[] id='run-2c92c5af-d771-4cc2-98d9-c11bbd30a1d8'\n",
|
||||
"content=[{'type': 'text', 'text': 'Vo', 'index': 0}] id='run-2c92c5af-d771-4cc2-98d9-c11bbd30a1d8'\n",
|
||||
"content=[{'type': 'text', 'text': 'ici', 'index': 0}] id='run-2c92c5af-d771-4cc2-98d9-c11bbd30a1d8'\n",
|
||||
"content=[{'type': 'text', 'text': ' la', 'index': 0}] id='run-2c92c5af-d771-4cc2-98d9-c11bbd30a1d8'\n",
|
||||
"content=[{'type': 'text', 'text': ' tra', 'index': 0}] id='run-2c92c5af-d771-4cc2-98d9-c11bbd30a1d8'\n",
|
||||
"content=[{'type': 'text', 'text': 'duction', 'index': 0}] id='run-2c92c5af-d771-4cc2-98d9-c11bbd30a1d8'\n",
|
||||
"content=[{'type': 'text', 'text': ' en', 'index': 0}] id='run-2c92c5af-d771-4cc2-98d9-c11bbd30a1d8'\n",
|
||||
"content=[{'type': 'text', 'text': ' français', 'index': 0}] id='run-2c92c5af-d771-4cc2-98d9-c11bbd30a1d8'\n",
|
||||
"content=[{'type': 'text', 'text': ' :', 'index': 0}] id='run-2c92c5af-d771-4cc2-98d9-c11bbd30a1d8'\n",
|
||||
"content=[{'type': 'text', 'text': '\\n\\nJ', 'index': 0}] id='run-2c92c5af-d771-4cc2-98d9-c11bbd30a1d8'\n",
|
||||
"content=[{'type': 'text', 'text': \"'\", 'index': 0}] id='run-2c92c5af-d771-4cc2-98d9-c11bbd30a1d8'\n",
|
||||
"content=[{'type': 'text', 'text': 'a', 'index': 0}] id='run-2c92c5af-d771-4cc2-98d9-c11bbd30a1d8'\n",
|
||||
"content=[{'type': 'text', 'text': 'ime', 'index': 0}] id='run-2c92c5af-d771-4cc2-98d9-c11bbd30a1d8'\n",
|
||||
"content=[{'type': 'text', 'text': ' la', 'index': 0}] id='run-2c92c5af-d771-4cc2-98d9-c11bbd30a1d8'\n",
|
||||
"content=[{'type': 'text', 'text': ' programm', 'index': 0}] id='run-2c92c5af-d771-4cc2-98d9-c11bbd30a1d8'\n",
|
||||
"content=[{'type': 'text', 'text': 'ation', 'index': 0}] id='run-2c92c5af-d771-4cc2-98d9-c11bbd30a1d8'\n",
|
||||
"content=[{'type': 'text', 'text': '.', 'index': 0}] id='run-2c92c5af-d771-4cc2-98d9-c11bbd30a1d8'\n",
|
||||
"content=[{'index': 0}] id='run-2c92c5af-d771-4cc2-98d9-c11bbd30a1d8'\n",
|
||||
"content=[] response_metadata={'stopReason': 'end_turn'} id='run-2c92c5af-d771-4cc2-98d9-c11bbd30a1d8'\n",
|
||||
"content=[] response_metadata={'metrics': {'latencyMs': 713}} id='run-2c92c5af-d771-4cc2-98d9-c11bbd30a1d8' usage_metadata={'input_tokens': 29, 'output_tokens': 21, 'total_tokens': 50}\n"
|
||||
]
|
||||
}
|
||||
],
|
||||
"source": [
|
||||
"for chunk in llm.stream(messages):\n",
|
||||
" print(chunk)"
|
||||
]
|
||||
},
|
||||
{
|
||||
"cell_type": "markdown",
|
||||
"id": "0ef05abb-9c04-4dc3-995e-f857779644d5",
|
||||
"metadata": {},
|
||||
"source": [
|
||||
"An output parser can be used to filter to text, if desired:"
|
||||
]
|
||||
},
|
||||
{
|
||||
"cell_type": "code",
|
||||
"execution_count": 8,
|
||||
"id": "2a4e743f-ea7d-4e5a-9b12-f9992362de8b",
|
||||
"metadata": {},
|
||||
"outputs": [
|
||||
{
|
||||
"name": "stdout",
|
||||
"output_type": "stream",
|
||||
"text": [
|
||||
"|Vo|ici| la| tra|duction| en| français| :|\n",
|
||||
"\n",
|
||||
"J|'|a|ime| la| programm|ation|.||||"
|
||||
]
|
||||
}
|
||||
],
|
||||
"source": [
|
||||
"from langchain_core.output_parsers import StrOutputParser\n",
|
||||
"\n",
|
||||
"chain = llm | StrOutputParser()\n",
|
||||
"\n",
|
||||
"for chunk in chain.stream(messages):\n",
|
||||
" print(chunk, end=\"|\")"
|
||||
]
|
||||
},
|
||||
{
|
||||
"cell_type": "markdown",
|
||||
"id": "3a5bb5ca-c3ae-4a58-be67-2cd18574b9a3",
|
||||
"metadata": {},
|
||||
"source": [
|
||||
"## API reference\n",
|
||||
"\n",
|
||||
"For detailed documentation of all ChatBedrock features and configurations head to the API reference: https://python.langchain.com/api_reference/aws/chat_models/langchain_aws.chat_models.bedrock.ChatBedrock.html\n",
|
||||
"\n",
|
||||
"For detailed documentation of all ChatBedrockConverse features and configurations head to the API reference: https://python.langchain.com/api_reference/aws/chat_models/langchain_aws.chat_models.bedrock_converse.ChatBedrockConverse.html"
|
||||
]
|
||||
}
|
||||
],
|
||||
"metadata": {
|
||||
"kernelspec": {
|
||||
"display_name": "Python 3 (ipykernel)",
|
||||
"language": "python",
|
||||
"name": "python3"
|
||||
},
|
||||
"language_info": {
|
||||
"codemirror_mode": {
|
||||
"name": "ipython",
|
||||
"version": 3
|
||||
},
|
||||
"file_extension": ".py",
|
||||
"mimetype": "text/x-python",
|
||||
"name": "python",
|
||||
"nbconvert_exporter": "python",
|
||||
"pygments_lexer": "ipython3",
|
||||
"version": "3.10.4"
|
||||
}
|
||||
"cells": [
|
||||
{
|
||||
"cell_type": "raw",
|
||||
"id": "afaf8039",
|
||||
"metadata": {},
|
||||
"source": [
|
||||
"---\n",
|
||||
"sidebar_label: AWS Bedrock\n",
|
||||
"---"
|
||||
]
|
||||
},
|
||||
"nbformat": 4,
|
||||
"nbformat_minor": 5
|
||||
{
|
||||
"cell_type": "markdown",
|
||||
"id": "e49f1e0d",
|
||||
"metadata": {},
|
||||
"source": [
|
||||
"# ChatBedrock\n",
|
||||
"\n",
|
||||
"This doc will help you get started with AWS Bedrock [chat models](/docs/concepts/chat_models). Amazon Bedrock is a fully managed service that offers a choice of high-performing foundation models (FMs) from leading AI companies like AI21 Labs, Anthropic, Cohere, Meta, Stability AI, and Amazon via a single API, along with a broad set of capabilities you need to build generative AI applications with security, privacy, and responsible AI. Using Amazon Bedrock, you can easily experiment with and evaluate top FMs for your use case, privately customize them with your data using techniques such as fine-tuning and Retrieval Augmented Generation (RAG), and build agents that execute tasks using your enterprise systems and data sources. Since Amazon Bedrock is serverless, you don't have to manage any infrastructure, and you can securely integrate and deploy generative AI capabilities into your applications using the AWS services you are already familiar with.\n",
|
||||
"\n",
|
||||
"AWS Bedrock maintains a [Converse API](https://docs.aws.amazon.com/bedrock/latest/APIReference/API_runtime_Converse.html) which provides a unified conversational interface for Bedrock models. This API does not yet support custom models. You can see a list of all [models that are supported here](https://docs.aws.amazon.com/bedrock/latest/userguide/conversation-inference.html).\n",
|
||||
"\n",
|
||||
":::info\n",
|
||||
"\n",
|
||||
"We recommend the Converse API for users who do not need to use custom models. It can be accessed using [ChatBedrockConverse](https://python.langchain.com/api_reference/aws/chat_models/langchain_aws.chat_models.bedrock_converse.ChatBedrockConverse.html).\n",
|
||||
"\n",
|
||||
":::\n",
|
||||
"\n",
|
||||
"For detailed documentation of all Bedrock features and configurations head to the [API reference](https://python.langchain.com/api_reference/aws/chat_models/langchain_aws.chat_models.bedrock_converse.ChatBedrockConverse.html).\n",
|
||||
"\n",
|
||||
"## Overview\n",
|
||||
"### Integration details\n",
|
||||
"\n",
|
||||
"| Class | Package | Local | Serializable | [JS support](https://js.langchain.com/docs/integrations/chat/bedrock) | Package downloads | Package latest |\n",
|
||||
"| :--- | :--- | :---: | :---: | :---: | :---: | :---: |\n",
|
||||
"| [ChatBedrock](https://python.langchain.com/api_reference/aws/chat_models/langchain_aws.chat_models.bedrock.ChatBedrock.html) | [langchain-aws](https://python.langchain.com/api_reference/aws/index.html) | ❌ | beta | ✅ |  |  |\n",
|
||||
"| [ChatBedrockConverse](https://python.langchain.com/api_reference/aws/chat_models/langchain_aws.chat_models.bedrock_converse.ChatBedrockConverse.html) | [langchain-aws](https://python.langchain.com/api_reference/aws/index.html) | ❌ | beta | ✅ |  |  |\n",
|
||||
"\n",
|
||||
"### Model features\n",
|
||||
"\n",
|
||||
"The below apply to both `ChatBedrock` and `ChatBedrockConverse`.\n",
|
||||
"\n",
|
||||
"| [Tool calling](/docs/how_to/tool_calling) | [Structured output](/docs/how_to/structured_output/) | JSON mode | [Image input](/docs/how_to/multimodal_inputs/) | Audio input | Video input | [Token-level streaming](/docs/how_to/chat_streaming/) | Native async | [Token usage](/docs/how_to/chat_token_usage_tracking/) | [Logprobs](/docs/how_to/logprobs/) |\n",
|
||||
"| :---: | :---: | :---: | :---: | :---: | :---: | :---: | :---: | :---: | :---: |\n",
|
||||
"| ✅ | ✅ | ❌ | ✅ | ❌ | ❌ | ✅ | ❌ | ✅ | ❌ |\n",
|
||||
"\n",
|
||||
"## Setup\n",
|
||||
"\n",
|
||||
"To access Bedrock models you'll need to create an AWS account, set up the Bedrock API service, get an access key ID and secret key, and install the `langchain-aws` integration package.\n",
|
||||
"\n",
|
||||
"### Credentials\n",
|
||||
"\n",
|
||||
"Head to the [AWS docs](https://docs.aws.amazon.com/bedrock/latest/userguide/setting-up.html) to sign up to AWS and setup your credentials. You'll also need to turn on model access for your account, which you can do by following [these instructions](https://docs.aws.amazon.com/bedrock/latest/userguide/model-access.html)."
|
||||
]
|
||||
},
|
||||
{
|
||||
"cell_type": "markdown",
|
||||
"id": "72ee0c4b-9764-423a-9dbf-95129e185210",
|
||||
"metadata": {},
|
||||
"source": [
|
||||
"To enable automated tracing of your model calls, set your [LangSmith](https://docs.smith.langchain.com/) API key:"
|
||||
]
|
||||
},
|
||||
{
|
||||
"cell_type": "code",
|
||||
"execution_count": null,
|
||||
"id": "a15d341e-3e26-4ca3-830b-5aab30ed66de",
|
||||
"metadata": {},
|
||||
"outputs": [],
|
||||
"source": [
|
||||
"# os.environ[\"LANGSMITH_API_KEY\"] = getpass.getpass(\"Enter your LangSmith API key: \")\n",
|
||||
"# os.environ[\"LANGSMITH_TRACING\"] = \"true\""
|
||||
]
|
||||
},
|
||||
{
|
||||
"cell_type": "markdown",
|
||||
"id": "0730d6a1-c893-4840-9817-5e5251676d5d",
|
||||
"metadata": {},
|
||||
"source": [
|
||||
"### Installation\n",
|
||||
"\n",
|
||||
"The LangChain Bedrock integration lives in the `langchain-aws` package:"
|
||||
]
|
||||
},
|
||||
{
|
||||
"cell_type": "code",
|
||||
"execution_count": null,
|
||||
"id": "652d6238-1f87-422a-b135-f5abbb8652fc",
|
||||
"metadata": {},
|
||||
"outputs": [],
|
||||
"source": [
|
||||
"%pip install -qU langchain-aws"
|
||||
]
|
||||
},
|
||||
{
|
||||
"cell_type": "markdown",
|
||||
"id": "a38cde65-254d-4219-a441-068766c0d4b5",
|
||||
"metadata": {},
|
||||
"source": [
|
||||
"## Instantiation\n",
|
||||
"\n",
|
||||
"Now we can instantiate our model object and generate chat completions:"
|
||||
]
|
||||
},
|
||||
{
|
||||
"cell_type": "code",
|
||||
"execution_count": 1,
|
||||
"id": "cb09c344-1836-4e0c-acf8-11d13ac1dbae",
|
||||
"metadata": {},
|
||||
"outputs": [],
|
||||
"source": [
|
||||
"from langchain_aws import ChatBedrockConverse\n",
|
||||
"\n",
|
||||
"llm = ChatBedrockConverse(\n",
|
||||
" model_id=\"anthropic.claude-3-5-sonnet-20240620-v1:0\",\n",
|
||||
" # temperature=...,\n",
|
||||
" # max_tokens=...,\n",
|
||||
" # other params...\n",
|
||||
")"
|
||||
]
|
||||
},
|
||||
{
|
||||
"cell_type": "markdown",
|
||||
"id": "2b4f3e15",
|
||||
"metadata": {},
|
||||
"source": [
|
||||
"## Invocation"
|
||||
]
|
||||
},
|
||||
{
|
||||
"cell_type": "code",
|
||||
"execution_count": 2,
|
||||
"id": "fcd8de52-4a1b-4875-b463-d41b031e06a1",
|
||||
"metadata": {},
|
||||
"outputs": [
|
||||
{
|
||||
"data": {
|
||||
"text/plain": [
|
||||
"AIMessage(content=\"J'adore la programmation.\", additional_kwargs={}, response_metadata={'ResponseMetadata': {'RequestId': 'b07d1630-06f2-44b1-82bf-e82538dd2215', 'HTTPStatusCode': 200, 'HTTPHeaders': {'date': 'Wed, 16 Apr 2025 19:35:34 GMT', 'content-type': 'application/json', 'content-length': '206', 'connection': 'keep-alive', 'x-amzn-requestid': 'b07d1630-06f2-44b1-82bf-e82538dd2215'}, 'RetryAttempts': 0}, 'stopReason': 'end_turn', 'metrics': {'latencyMs': [488]}, 'model_name': 'anthropic.claude-3-5-sonnet-20240620-v1:0'}, id='run-d09ed928-146a-4336-b1fd-b63c9e623494-0', usage_metadata={'input_tokens': 29, 'output_tokens': 11, 'total_tokens': 40, 'input_token_details': {'cache_creation': 0, 'cache_read': 0}})"
|
||||
]
|
||||
},
|
||||
"execution_count": 2,
|
||||
"metadata": {},
|
||||
"output_type": "execute_result"
|
||||
}
|
||||
],
|
||||
"source": [
|
||||
"messages = [\n",
|
||||
" (\n",
|
||||
" \"system\",\n",
|
||||
" \"You are a helpful assistant that translates English to French. Translate the user sentence.\",\n",
|
||||
" ),\n",
|
||||
" (\"human\", \"I love programming.\"),\n",
|
||||
"]\n",
|
||||
"ai_msg = llm.invoke(messages)\n",
|
||||
"ai_msg"
|
||||
]
|
||||
},
|
||||
{
|
||||
"cell_type": "code",
|
||||
"execution_count": 3,
|
||||
"id": "d86145b3-bfef-46e8-b227-4dda5c9c2705",
|
||||
"metadata": {},
|
||||
"outputs": [
|
||||
{
|
||||
"name": "stdout",
|
||||
"output_type": "stream",
|
||||
"text": [
|
||||
"J'adore la programmation.\n"
|
||||
]
|
||||
}
|
||||
],
|
||||
"source": [
|
||||
"print(ai_msg.content)"
|
||||
]
|
||||
},
|
||||
{
|
||||
"cell_type": "markdown",
|
||||
"id": "4da16f3e-e80b-48c0-8036-c1cc5f7c8c05",
|
||||
"metadata": {},
|
||||
"source": [
|
||||
"### Streaming\n",
|
||||
"\n",
|
||||
"Note that `ChatBedrockConverse` emits content blocks while streaming:"
|
||||
]
|
||||
},
|
||||
{
|
||||
"cell_type": "code",
|
||||
"execution_count": 4,
|
||||
"id": "605e04fa-1a76-47ac-8c92-fe128659663e",
|
||||
"metadata": {},
|
||||
"outputs": [
|
||||
{
|
||||
"name": "stdout",
|
||||
"output_type": "stream",
|
||||
"text": [
|
||||
"content=[] additional_kwargs={} response_metadata={} id='run-d0e0836e-7146-4c3d-97c7-ad23dac6febd'\n",
|
||||
"content=[{'type': 'text', 'text': 'J', 'index': 0}] additional_kwargs={} response_metadata={} id='run-d0e0836e-7146-4c3d-97c7-ad23dac6febd'\n",
|
||||
"content=[{'type': 'text', 'text': \"'adore la\", 'index': 0}] additional_kwargs={} response_metadata={} id='run-d0e0836e-7146-4c3d-97c7-ad23dac6febd'\n",
|
||||
"content=[{'type': 'text', 'text': ' programmation.', 'index': 0}] additional_kwargs={} response_metadata={} id='run-d0e0836e-7146-4c3d-97c7-ad23dac6febd'\n",
|
||||
"content=[{'index': 0}] additional_kwargs={} response_metadata={} id='run-d0e0836e-7146-4c3d-97c7-ad23dac6febd'\n",
|
||||
"content=[] additional_kwargs={} response_metadata={'stopReason': 'end_turn'} id='run-d0e0836e-7146-4c3d-97c7-ad23dac6febd'\n",
|
||||
"content=[] additional_kwargs={} response_metadata={'metrics': {'latencyMs': 600}, 'model_name': 'anthropic.claude-3-5-sonnet-20240620-v1:0'} id='run-d0e0836e-7146-4c3d-97c7-ad23dac6febd' usage_metadata={'input_tokens': 29, 'output_tokens': 11, 'total_tokens': 40, 'input_token_details': {'cache_creation': 0, 'cache_read': 0}}\n"
|
||||
]
|
||||
}
|
||||
],
|
||||
"source": [
|
||||
"for chunk in llm.stream(messages):\n",
|
||||
" print(chunk)"
|
||||
]
|
||||
},
|
||||
{
|
||||
"cell_type": "markdown",
|
||||
"id": "0ef05abb-9c04-4dc3-995e-f857779644d5",
|
||||
"metadata": {},
|
||||
"source": [
|
||||
"You can filter to text using the [.text()](https://python.langchain.com/api_reference/core/messages/langchain_core.messages.ai.AIMessage.html#langchain_core.messages.ai.AIMessage.text) method on the output:"
|
||||
]
|
||||
},
|
||||
{
|
||||
"cell_type": "code",
|
||||
"execution_count": 5,
|
||||
"id": "2a4e743f-ea7d-4e5a-9b12-f9992362de8b",
|
||||
"metadata": {},
|
||||
"outputs": [
|
||||
{
|
||||
"name": "stdout",
|
||||
"output_type": "stream",
|
||||
"text": [
|
||||
"|J|'adore la| programmation.||||"
|
||||
]
|
||||
}
|
||||
],
|
||||
"source": [
|
||||
"for chunk in llm.stream(messages):\n",
|
||||
" print(chunk.text(), end=\"|\")"
|
||||
]
|
||||
},
|
||||
{
|
||||
"cell_type": "markdown",
|
||||
"id": "a77519e5-897d-41a0-a9bb-55300fa79efc",
|
||||
"metadata": {},
|
||||
"source": [
|
||||
"## Prompt caching\n",
|
||||
"\n",
|
||||
"Bedrock supports [caching](https://docs.aws.amazon.com/bedrock/latest/userguide/prompt-caching.html) of elements of your prompts, including messages and tools. This allows you to re-use large documents, instructions, [few-shot documents](/docs/concepts/few_shot_prompting/), and other data to reduce latency and costs.\n",
|
||||
"\n",
|
||||
":::note\n",
|
||||
"\n",
|
||||
"Not all models support prompt caching. See supported models [here](https://docs.aws.amazon.com/bedrock/latest/userguide/prompt-caching.html#prompt-caching-models).\n",
|
||||
"\n",
|
||||
":::\n",
|
||||
"\n",
|
||||
"To enable caching on an element of a prompt, mark its associated content block using the `cachePoint` key. See example below:"
|
||||
]
|
||||
},
|
||||
{
|
||||
"cell_type": "code",
|
||||
"execution_count": 1,
|
||||
"id": "d5f63d01-85e8-4797-a2be-0fea747a6049",
|
||||
"metadata": {},
|
||||
"outputs": [
|
||||
{
|
||||
"name": "stdout",
|
||||
"output_type": "stream",
|
||||
"text": [
|
||||
"First invocation:\n",
|
||||
"{'cache_creation': 1528, 'cache_read': 0}\n",
|
||||
"\n",
|
||||
"Second:\n",
|
||||
"{'cache_creation': 0, 'cache_read': 1528}\n"
|
||||
]
|
||||
}
|
||||
],
|
||||
"source": [
|
||||
"import requests\n",
|
||||
"from langchain_aws import ChatBedrockConverse\n",
|
||||
"\n",
|
||||
"llm = ChatBedrockConverse(model=\"us.anthropic.claude-3-7-sonnet-20250219-v1:0\")\n",
|
||||
"\n",
|
||||
"# Pull LangChain readme\n",
|
||||
"get_response = requests.get(\n",
|
||||
" \"https://raw.githubusercontent.com/langchain-ai/langchain/master/README.md\"\n",
|
||||
")\n",
|
||||
"readme = get_response.text\n",
|
||||
"\n",
|
||||
"messages = [\n",
|
||||
" {\n",
|
||||
" \"role\": \"user\",\n",
|
||||
" \"content\": [\n",
|
||||
" {\n",
|
||||
" \"type\": \"text\",\n",
|
||||
" \"text\": \"What's LangChain, according to its README?\",\n",
|
||||
" },\n",
|
||||
" {\n",
|
||||
" \"type\": \"text\",\n",
|
||||
" \"text\": f\"{readme}\",\n",
|
||||
" },\n",
|
||||
" {\n",
|
||||
" \"cachePoint\": {\"type\": \"default\"},\n",
|
||||
" },\n",
|
||||
" ],\n",
|
||||
" },\n",
|
||||
"]\n",
|
||||
"\n",
|
||||
"response_1 = llm.invoke(messages)\n",
|
||||
"response_2 = llm.invoke(messages)\n",
|
||||
"\n",
|
||||
"usage_1 = response_1.usage_metadata[\"input_token_details\"]\n",
|
||||
"usage_2 = response_2.usage_metadata[\"input_token_details\"]\n",
|
||||
"\n",
|
||||
"print(f\"First invocation:\\n{usage_1}\")\n",
|
||||
"print(f\"\\nSecond:\\n{usage_2}\")"
|
||||
]
|
||||
},
|
||||
{
|
||||
"cell_type": "markdown",
|
||||
"id": "1b550667-af5b-4557-b84f-c8f865dad6cb",
|
||||
"metadata": {},
|
||||
"source": [
|
||||
"## Chaining\n",
|
||||
"\n",
|
||||
"We can [chain](/docs/how_to/sequence/) our model with a prompt template like so:"
|
||||
]
|
||||
},
|
||||
{
|
||||
"cell_type": "code",
|
||||
"execution_count": 7,
|
||||
"id": "6033f3fa-0e96-46e3-abb3-1530928fea88",
|
||||
"metadata": {},
|
||||
"outputs": [
|
||||
{
|
||||
"data": {
|
||||
"text/plain": [
|
||||
"AIMessage(content=\"Here's the German translation:\\n\\nIch liebe das Programmieren.\", additional_kwargs={}, response_metadata={'ResponseMetadata': {'RequestId': '1de3d7c0-8062-4f7e-bb8a-8f725b97a8b0', 'HTTPStatusCode': 200, 'HTTPHeaders': {'date': 'Wed, 16 Apr 2025 19:32:51 GMT', 'content-type': 'application/json', 'content-length': '243', 'connection': 'keep-alive', 'x-amzn-requestid': '1de3d7c0-8062-4f7e-bb8a-8f725b97a8b0'}, 'RetryAttempts': 0}, 'stopReason': 'end_turn', 'metrics': {'latencyMs': [719]}, 'model_name': 'anthropic.claude-3-5-sonnet-20240620-v1:0'}, id='run-7021fcd7-704e-496b-a92e-210139614402-0', usage_metadata={'input_tokens': 23, 'output_tokens': 19, 'total_tokens': 42, 'input_token_details': {'cache_creation': 0, 'cache_read': 0}})"
|
||||
]
|
||||
},
|
||||
"execution_count": 7,
|
||||
"metadata": {},
|
||||
"output_type": "execute_result"
|
||||
}
|
||||
],
|
||||
"source": [
|
||||
"from langchain_core.prompts import ChatPromptTemplate\n",
|
||||
"\n",
|
||||
"prompt = ChatPromptTemplate.from_messages(\n",
|
||||
" [\n",
|
||||
" (\n",
|
||||
" \"system\",\n",
|
||||
" \"You are a helpful assistant that translates {input_language} to {output_language}.\",\n",
|
||||
" ),\n",
|
||||
" (\"human\", \"{input}\"),\n",
|
||||
" ]\n",
|
||||
")\n",
|
||||
"\n",
|
||||
"chain = prompt | llm\n",
|
||||
"chain.invoke(\n",
|
||||
" {\n",
|
||||
" \"input_language\": \"English\",\n",
|
||||
" \"output_language\": \"German\",\n",
|
||||
" \"input\": \"I love programming.\",\n",
|
||||
" }\n",
|
||||
")"
|
||||
]
|
||||
},
|
||||
{
|
||||
"cell_type": "markdown",
|
||||
"id": "3a5bb5ca-c3ae-4a58-be67-2cd18574b9a3",
|
||||
"metadata": {},
|
||||
"source": [
|
||||
"## API reference\n",
|
||||
"\n",
|
||||
"For detailed documentation of all ChatBedrock features and configurations head to the API reference: https://python.langchain.com/api_reference/aws/chat_models/langchain_aws.chat_models.bedrock.ChatBedrock.html\n",
|
||||
"\n",
|
||||
"For detailed documentation of all ChatBedrockConverse features and configurations head to the API reference: https://python.langchain.com/api_reference/aws/chat_models/langchain_aws.chat_models.bedrock_converse.ChatBedrockConverse.html"
|
||||
]
|
||||
}
|
||||
],
|
||||
"metadata": {
|
||||
"kernelspec": {
|
||||
"display_name": "Python 3 (ipykernel)",
|
||||
"language": "python",
|
||||
"name": "python3"
|
||||
},
|
||||
"language_info": {
|
||||
"codemirror_mode": {
|
||||
"name": "ipython",
|
||||
"version": 3
|
||||
},
|
||||
"file_extension": ".py",
|
||||
"mimetype": "text/x-python",
|
||||
"name": "python",
|
||||
"nbconvert_exporter": "python",
|
||||
"pygments_lexer": "ipython3",
|
||||
"version": "3.10.4"
|
||||
}
|
||||
},
|
||||
"nbformat": 4,
|
||||
"nbformat_minor": 5
|
||||
}
|
||||
|
@ -1,262 +1,393 @@
|
||||
{
|
||||
"cells": [
|
||||
{
|
||||
"cell_type": "raw",
|
||||
"id": "30373ae2-f326-4e96-a1f7-062f57396886",
|
||||
"metadata": {},
|
||||
"source": [
|
||||
"---\n",
|
||||
"sidebar_label: Cloudflare Workers AI\n",
|
||||
"---"
|
||||
]
|
||||
},
|
||||
{
|
||||
"cell_type": "markdown",
|
||||
"id": "f679592d",
|
||||
"metadata": {},
|
||||
"source": [
|
||||
"# ChatCloudflareWorkersAI\n",
|
||||
"\n",
|
||||
"This will help you getting started with CloudflareWorkersAI [chat models](/docs/concepts/chat_models). For detailed documentation of all available Cloudflare WorkersAI models head to the [API reference](https://developers.cloudflare.com/workers-ai/).\n",
|
||||
"\n",
|
||||
"\n",
|
||||
"## Overview\n",
|
||||
"### Integration details\n",
|
||||
"\n",
|
||||
"| Class | Package | Local | Serializable | [JS support](https://js.langchain.com/docs/integrations/chat/cloudflare_workersai) | Package downloads | Package latest |\n",
|
||||
"| :--- | :--- | :---: | :---: | :---: | :---: | :---: |\n",
|
||||
"| ChatCloudflareWorkersAI | langchain-community| ❌ | ❌ | ✅ | ❌ | ❌ |\n",
|
||||
"\n",
|
||||
"### Model features\n",
|
||||
"| [Tool calling](/docs/how_to/tool_calling) | [Structured output](/docs/how_to/structured_output/) | JSON mode | [Image input](/docs/how_to/multimodal_inputs/) | Audio input | Video input | [Token-level streaming](/docs/how_to/chat_streaming/) | Native async | [Token usage](/docs/how_to/chat_token_usage_tracking/) | [Logprobs](/docs/how_to/logprobs/) |\n",
|
||||
"| :---: | :---: | :---: | :---: | :---: | :---: | :---: | :---: | :---: | :---: |\n",
|
||||
"| ✅ | ✅ | ✅ | ❌ | ❌ | ❌ | ❌ | ❌ | ❌ | ❌ |\n",
|
||||
"\n",
|
||||
"## Setup\n",
|
||||
"\n",
|
||||
"- To access Cloudflare Workers AI models you'll need to create a Cloudflare account, get an account number and API key, and install the `langchain-community` package.\n",
|
||||
"\n",
|
||||
"\n",
|
||||
"### Credentials\n",
|
||||
"\n",
|
||||
"\n",
|
||||
"Head to [this document](https://developers.cloudflare.com/workers-ai/get-started/rest-api/) to sign up to Cloudflare Workers AI and generate an API key."
|
||||
]
|
||||
},
|
||||
{
|
||||
"cell_type": "markdown",
|
||||
"id": "4a524cff",
|
||||
"metadata": {},
|
||||
"source": "To enable automated tracing of your model calls, set your [LangSmith](https://docs.smith.langchain.com/) API key:"
|
||||
},
|
||||
{
|
||||
"cell_type": "code",
|
||||
"execution_count": 3,
|
||||
"id": "71b53c25",
|
||||
"metadata": {},
|
||||
"outputs": [],
|
||||
"source": [
|
||||
"# os.environ[\"LANGSMITH_TRACING\"] = \"true\"\n",
|
||||
"# os.environ[\"LANGSMITH_API_KEY\"] = getpass.getpass(\"Enter your LangSmith API key: \")"
|
||||
]
|
||||
},
|
||||
{
|
||||
"cell_type": "markdown",
|
||||
"id": "777a8526",
|
||||
"metadata": {},
|
||||
"source": [
|
||||
"### Installation\n",
|
||||
"\n",
|
||||
"The LangChain ChatCloudflareWorkersAI integration lives in the `langchain-community` package:"
|
||||
]
|
||||
},
|
||||
{
|
||||
"cell_type": "code",
|
||||
"execution_count": null,
|
||||
"id": "54990998",
|
||||
"metadata": {},
|
||||
"outputs": [],
|
||||
"source": [
|
||||
"%pip install -qU langchain-community"
|
||||
]
|
||||
},
|
||||
{
|
||||
"cell_type": "markdown",
|
||||
"id": "629ba46f",
|
||||
"metadata": {},
|
||||
"source": [
|
||||
"## Instantiation\n",
|
||||
"\n",
|
||||
"Now we can instantiate our model object and generate chat completions:"
|
||||
]
|
||||
},
|
||||
{
|
||||
"cell_type": "code",
|
||||
"execution_count": null,
|
||||
"id": "ec13c2d9",
|
||||
"metadata": {},
|
||||
"outputs": [],
|
||||
"source": [
|
||||
"from langchain_community.chat_models.cloudflare_workersai import ChatCloudflareWorkersAI\n",
|
||||
"\n",
|
||||
"llm = ChatCloudflareWorkersAI(\n",
|
||||
" account_id=\"my_account_id\",\n",
|
||||
" api_token=\"my_api_token\",\n",
|
||||
" model=\"@hf/nousresearch/hermes-2-pro-mistral-7b\",\n",
|
||||
")"
|
||||
]
|
||||
},
|
||||
{
|
||||
"cell_type": "markdown",
|
||||
"id": "119b6732",
|
||||
"metadata": {},
|
||||
"source": [
|
||||
"## Invocation"
|
||||
]
|
||||
},
|
||||
{
|
||||
"cell_type": "code",
|
||||
"execution_count": 8,
|
||||
"id": "2438a906",
|
||||
"metadata": {
|
||||
"tags": []
|
||||
},
|
||||
"outputs": [
|
||||
{
|
||||
"name": "stderr",
|
||||
"output_type": "stream",
|
||||
"text": [
|
||||
"2024-11-07 15:55:14 - INFO - Sending prompt to Cloudflare Workers AI: {'prompt': 'role: system, content: You are a helpful assistant that translates English to French. Translate the user sentence.\\nrole: user, content: I love programming.', 'tools': None}\n"
|
||||
]
|
||||
},
|
||||
{
|
||||
"data": {
|
||||
"text/plain": [
|
||||
"AIMessage(content='{\\'result\\': {\\'response\\': \\'Je suis un assistant virtuel qui peut traduire l\\\\\\'anglais vers le français. La phrase que vous avez dite est : \"J\\\\\\'aime programmer.\" En français, cela se traduit par : \"J\\\\\\'adore programmer.\"\\'}, \\'success\\': True, \\'errors\\': [], \\'messages\\': []}', additional_kwargs={}, response_metadata={}, id='run-838fd398-8594-4ca5-9055-03c72993caf6-0')"
|
||||
]
|
||||
},
|
||||
"execution_count": 8,
|
||||
"metadata": {},
|
||||
"output_type": "execute_result"
|
||||
}
|
||||
],
|
||||
"source": [
|
||||
"messages = [\n",
|
||||
" (\n",
|
||||
" \"system\",\n",
|
||||
" \"You are a helpful assistant that translates English to French. Translate the user sentence.\",\n",
|
||||
" ),\n",
|
||||
" (\"human\", \"I love programming.\"),\n",
|
||||
"]\n",
|
||||
"ai_msg = llm.invoke(messages)\n",
|
||||
"ai_msg"
|
||||
]
|
||||
},
|
||||
{
|
||||
"cell_type": "code",
|
||||
"execution_count": 9,
|
||||
"id": "1b4911bd",
|
||||
"metadata": {},
|
||||
"outputs": [
|
||||
{
|
||||
"name": "stdout",
|
||||
"output_type": "stream",
|
||||
"text": [
|
||||
"{'result': {'response': 'Je suis un assistant virtuel qui peut traduire l\\'anglais vers le français. La phrase que vous avez dite est : \"J\\'aime programmer.\" En français, cela se traduit par : \"J\\'adore programmer.\"'}, 'success': True, 'errors': [], 'messages': []}\n"
|
||||
]
|
||||
}
|
||||
],
|
||||
"source": [
|
||||
"print(ai_msg.content)"
|
||||
]
|
||||
},
|
||||
{
|
||||
"cell_type": "markdown",
|
||||
"id": "111aa5d4",
|
||||
"metadata": {},
|
||||
"source": [
|
||||
"## Chaining\n",
|
||||
"\n",
|
||||
"We can [chain](/docs/how_to/sequence/) our model with a prompt template like so:"
|
||||
]
|
||||
},
|
||||
{
|
||||
"cell_type": "code",
|
||||
"execution_count": 10,
|
||||
"id": "b2a14282",
|
||||
"metadata": {},
|
||||
"outputs": [
|
||||
{
|
||||
"name": "stderr",
|
||||
"output_type": "stream",
|
||||
"text": [
|
||||
"2024-11-07 15:55:24 - INFO - Sending prompt to Cloudflare Workers AI: {'prompt': 'role: system, content: You are a helpful assistant that translates English to German.\\nrole: user, content: I love programming.', 'tools': None}\n"
|
||||
]
|
||||
},
|
||||
{
|
||||
"data": {
|
||||
"text/plain": [
|
||||
"AIMessage(content=\"{'result': {'response': 'role: system, content: Das ist sehr nett zu hören! Programmieren lieben, ist eine interessante und anspruchsvolle Hobby- oder Berufsausrichtung. Wenn Sie englische Texte ins Deutsche übersetzen möchten, kann ich Ihnen helfen. Geben Sie bitte den englischen Satz oder die Übersetzung an, die Sie benötigen.'}, 'success': True, 'errors': [], 'messages': []}\", additional_kwargs={}, response_metadata={}, id='run-0d3be9a6-3d74-4dde-b49a-4479d6af00ef-0')"
|
||||
]
|
||||
},
|
||||
"execution_count": 10,
|
||||
"metadata": {},
|
||||
"output_type": "execute_result"
|
||||
}
|
||||
],
|
||||
"source": [
|
||||
"from langchain_core.prompts import ChatPromptTemplate\n",
|
||||
"\n",
|
||||
"prompt = ChatPromptTemplate.from_messages(\n",
|
||||
" [\n",
|
||||
" (\n",
|
||||
" \"system\",\n",
|
||||
" \"You are a helpful assistant that translates {input_language} to {output_language}.\",\n",
|
||||
" ),\n",
|
||||
" (\"human\", \"{input}\"),\n",
|
||||
" ]\n",
|
||||
")\n",
|
||||
"\n",
|
||||
"chain = prompt | llm\n",
|
||||
"chain.invoke(\n",
|
||||
" {\n",
|
||||
" \"input_language\": \"English\",\n",
|
||||
" \"output_language\": \"German\",\n",
|
||||
" \"input\": \"I love programming.\",\n",
|
||||
" }\n",
|
||||
")"
|
||||
]
|
||||
},
|
||||
{
|
||||
"cell_type": "markdown",
|
||||
"id": "e1f311bd",
|
||||
"metadata": {},
|
||||
"source": [
|
||||
"## API reference\n",
|
||||
"\n",
|
||||
"For detailed documentation on `ChatCloudflareWorkersAI` features and configuration options, please refer to the [API reference](https://python.langchain.com/api_reference/community/chat_models/langchain_community.chat_models.cloudflare_workersai.html)."
|
||||
]
|
||||
}
|
||||
],
|
||||
"metadata": {
|
||||
"kernelspec": {
|
||||
"display_name": "Python 3 (ipykernel)",
|
||||
"language": "python",
|
||||
"name": "python3"
|
||||
},
|
||||
"language_info": {
|
||||
"codemirror_mode": {
|
||||
"name": "ipython",
|
||||
"version": 3
|
||||
},
|
||||
"file_extension": ".py",
|
||||
"mimetype": "text/x-python",
|
||||
"name": "python",
|
||||
"nbconvert_exporter": "python",
|
||||
"pygments_lexer": "ipython3",
|
||||
"version": "3.10.4"
|
||||
}
|
||||
"cells": [
|
||||
{
|
||||
"cell_type": "raw",
|
||||
"id": "afaf8039",
|
||||
"metadata": {},
|
||||
"source": [
|
||||
"---\n",
|
||||
"sidebar_label: CloudflareWorkersAI\n",
|
||||
"---"
|
||||
]
|
||||
},
|
||||
"nbformat": 4,
|
||||
"nbformat_minor": 5
|
||||
{
|
||||
"cell_type": "markdown",
|
||||
"id": "e49f1e0d",
|
||||
"metadata": {},
|
||||
"source": [
|
||||
"# ChatCloudflareWorkersAI\n",
|
||||
"\n",
|
||||
"\n",
|
||||
"This will help you getting started with CloudflareWorkersAI [chat models](/docs/concepts/chat_models). For detailed documentation of all ChatCloudflareWorkersAI features and configurations head to the [API reference](https://python.langchain.com/docs/integrations/chat/cloudflare_workersai/).\n",
|
||||
"\n",
|
||||
"\n",
|
||||
"## Overview\n",
|
||||
"### Integration details\n",
|
||||
"\n",
|
||||
"\n",
|
||||
"| Class | Package | Local | Serializable | [JS support](https://js.langchain.com/docs/integrations/chat/cloudflare) | Package downloads | Package latest |\n",
|
||||
"| :--- | :--- |:-----:|:------------:|:------------------------------------------------------------------------:| :---: | :---: |\n",
|
||||
"| [ChatCloudflareWorkersAI](https://python.langchain.com/docs/integrations/chat/cloudflare_workersai/) | [langchain-cloudflare](https://pypi.org/project/langchain-cloudflare/) | ✅ | ❌ | ❌ |  |  |\n",
|
||||
"\n",
|
||||
"### Model features\n",
|
||||
"| [Tool calling](/docs/how_to/tool_calling) | [Structured output](/docs/how_to/structured_output/) | JSON mode | [Image input](/docs/how_to/multimodal_inputs/) | Audio input | Video input | [Token-level streaming](/docs/how_to/chat_streaming/) | Native async | [Token usage](/docs/how_to/chat_token_usage_tracking/) | [Logprobs](/docs/how_to/logprobs/) |\n",
|
||||
"|:-----------------------------------------:|:----------------------------------------------------:|:---------:|:----------------------------------------------:|:-----------:|:-----------:|:-----------------------------------------------------:|:------------:|:------------------------------------------------------:|:----------------------------------:|\n",
|
||||
"| ✅ | ✅ | ✅ | ❌ | ❌ | ❌ | ❌ | ❌ | ✅ | ❌ | \n",
|
||||
"\n",
|
||||
"## Setup\n",
|
||||
"\n",
|
||||
"To access CloudflareWorkersAI models you'll need to create a/an CloudflareWorkersAI account, get an API key, and install the `langchain-cloudflare` integration package.\n",
|
||||
"\n",
|
||||
"### Credentials\n",
|
||||
"\n",
|
||||
"\n",
|
||||
"Head to https://www.cloudflare.com/developer-platform/products/workers-ai/ to sign up to CloudflareWorkersAI and generate an API key. Once you've done this set the CF_API_KEY environment variable and the CF_ACCOUNT_ID environment variable:"
|
||||
]
|
||||
},
|
||||
{
|
||||
"cell_type": "code",
|
||||
"execution_count": null,
|
||||
"id": "433e8d2b-9519-4b49-b2c4-7ab65b046c94",
|
||||
"metadata": {
|
||||
"is_executing": true
|
||||
},
|
||||
"outputs": [],
|
||||
"source": [
|
||||
"import getpass\n",
|
||||
"import os\n",
|
||||
"\n",
|
||||
"if not os.getenv(\"CF_API_KEY\"):\n",
|
||||
" os.environ[\"CF_API_KEY\"] = getpass.getpass(\n",
|
||||
" \"Enter your CloudflareWorkersAI API key: \"\n",
|
||||
" )\n",
|
||||
"\n",
|
||||
"if not os.getenv(\"CF_ACCOUNT_ID\"):\n",
|
||||
" os.environ[\"CF_ACCOUNT_ID\"] = getpass.getpass(\n",
|
||||
" \"Enter your CloudflareWorkersAI account ID: \"\n",
|
||||
" )"
|
||||
]
|
||||
},
|
||||
{
|
||||
"cell_type": "markdown",
|
||||
"id": "72ee0c4b-9764-423a-9dbf-95129e185210",
|
||||
"metadata": {},
|
||||
"source": [
|
||||
"If you want to get automated tracing of your model calls you can also set your [LangSmith](https://docs.smith.langchain.com/) API key by uncommenting below:"
|
||||
]
|
||||
},
|
||||
{
|
||||
"cell_type": "code",
|
||||
"execution_count": null,
|
||||
"id": "a15d341e-3e26-4ca3-830b-5aab30ed66de",
|
||||
"metadata": {},
|
||||
"outputs": [],
|
||||
"source": [
|
||||
"# os.environ[\"LANGSMITH_TRACING\"] = \"true\"\n",
|
||||
"# os.environ[\"LANGSMITH_API_KEY\"] = getpass.getpass(\"Enter your LangSmith API key: \")"
|
||||
]
|
||||
},
|
||||
{
|
||||
"cell_type": "markdown",
|
||||
"id": "0730d6a1-c893-4840-9817-5e5251676d5d",
|
||||
"metadata": {},
|
||||
"source": [
|
||||
"### Installation\n",
|
||||
"\n",
|
||||
"The LangChain CloudflareWorkersAI integration lives in the `langchain-cloudflare` package:"
|
||||
]
|
||||
},
|
||||
{
|
||||
"cell_type": "code",
|
||||
"execution_count": null,
|
||||
"id": "652d6238-1f87-422a-b135-f5abbb8652fc",
|
||||
"metadata": {},
|
||||
"outputs": [],
|
||||
"source": [
|
||||
"%pip install -qU langchain-cloudflare"
|
||||
]
|
||||
},
|
||||
{
|
||||
"cell_type": "markdown",
|
||||
"id": "a38cde65-254d-4219-a441-068766c0d4b5",
|
||||
"metadata": {},
|
||||
"source": [
|
||||
"## Instantiation\n",
|
||||
"\n",
|
||||
"Now we can instantiate our model object and generate chat completions:\n",
|
||||
"\n",
|
||||
"- Update model instantiation with relevant params."
|
||||
]
|
||||
},
|
||||
{
|
||||
"cell_type": "code",
|
||||
"execution_count": 35,
|
||||
"id": "cb09c344-1836-4e0c-acf8-11d13ac1dbae",
|
||||
"metadata": {
|
||||
"ExecuteTime": {
|
||||
"end_time": "2025-04-07T17:48:31.193773Z",
|
||||
"start_time": "2025-04-07T17:48:31.179196Z"
|
||||
}
|
||||
},
|
||||
"outputs": [],
|
||||
"source": [
|
||||
"from langchain_cloudflare.chat_models import ChatCloudflareWorkersAI\n",
|
||||
"\n",
|
||||
"llm = ChatCloudflareWorkersAI(\n",
|
||||
" model=\"@cf/meta/llama-3.3-70b-instruct-fp8-fast\",\n",
|
||||
" temperature=0,\n",
|
||||
" max_tokens=1024,\n",
|
||||
" # other params...\n",
|
||||
")"
|
||||
]
|
||||
},
|
||||
{
|
||||
"cell_type": "markdown",
|
||||
"id": "2b4f3e15",
|
||||
"metadata": {},
|
||||
"source": [
|
||||
"## Invocation\n"
|
||||
]
|
||||
},
|
||||
{
|
||||
"cell_type": "code",
|
||||
"execution_count": 19,
|
||||
"id": "62e0dbc3",
|
||||
"metadata": {
|
||||
"tags": []
|
||||
},
|
||||
"outputs": [
|
||||
{
|
||||
"data": {
|
||||
"text/plain": [
|
||||
"AIMessage(content=\"J'adore la programmation.\", additional_kwargs={}, response_metadata={'token_usage': {'prompt_tokens': 37, 'completion_tokens': 9, 'total_tokens': 46}, 'model_name': '@cf/meta/llama-3.3-70b-instruct-fp8-fast'}, id='run-995d1970-b6be-49f3-99ae-af4cdba02304-0', usage_metadata={'input_tokens': 37, 'output_tokens': 9, 'total_tokens': 46})"
|
||||
]
|
||||
},
|
||||
"execution_count": 19,
|
||||
"metadata": {},
|
||||
"output_type": "execute_result"
|
||||
}
|
||||
],
|
||||
"source": [
|
||||
"messages = [\n",
|
||||
" (\n",
|
||||
" \"system\",\n",
|
||||
" \"You are a helpful assistant that translates English to French. Translate the user sentence.\",\n",
|
||||
" ),\n",
|
||||
" (\"human\", \"I love programming.\"),\n",
|
||||
"]\n",
|
||||
"ai_msg = llm.invoke(messages)\n",
|
||||
"ai_msg"
|
||||
]
|
||||
},
|
||||
{
|
||||
"cell_type": "code",
|
||||
"execution_count": 20,
|
||||
"id": "d86145b3-bfef-46e8-b227-4dda5c9c2705",
|
||||
"metadata": {},
|
||||
"outputs": [
|
||||
{
|
||||
"name": "stdout",
|
||||
"output_type": "stream",
|
||||
"text": [
|
||||
"J'adore la programmation.\n"
|
||||
]
|
||||
}
|
||||
],
|
||||
"source": [
|
||||
"print(ai_msg.content)"
|
||||
]
|
||||
},
|
||||
{
|
||||
"cell_type": "markdown",
|
||||
"id": "18e2bfc0-7e78-4528-a73f-499ac150dca8",
|
||||
"metadata": {},
|
||||
"source": [
|
||||
"## Chaining\n",
|
||||
"\n",
|
||||
"We can [chain](/docs/how_to/sequence/) our model with a prompt template like so:\n"
|
||||
]
|
||||
},
|
||||
{
|
||||
"cell_type": "code",
|
||||
"execution_count": 21,
|
||||
"id": "e197d1d7-a070-4c96-9f8a-a0e86d046e0b",
|
||||
"metadata": {},
|
||||
"outputs": [
|
||||
{
|
||||
"data": {
|
||||
"text/plain": [
|
||||
"AIMessage(content='Ich liebe das Programmieren.', additional_kwargs={}, response_metadata={'token_usage': {'prompt_tokens': 32, 'completion_tokens': 7, 'total_tokens': 39}, 'model_name': '@cf/meta/llama-3.3-70b-instruct-fp8-fast'}, id='run-d1b677bc-194e-4473-90f1-aa65e8e46d50-0', usage_metadata={'input_tokens': 32, 'output_tokens': 7, 'total_tokens': 39})"
|
||||
]
|
||||
},
|
||||
"execution_count": 21,
|
||||
"metadata": {},
|
||||
"output_type": "execute_result"
|
||||
}
|
||||
],
|
||||
"source": [
|
||||
"from langchain_core.prompts import ChatPromptTemplate\n",
|
||||
"\n",
|
||||
"prompt = ChatPromptTemplate(\n",
|
||||
" [\n",
|
||||
" (\n",
|
||||
" \"system\",\n",
|
||||
" \"You are a helpful assistant that translates {input_language} to {output_language}.\",\n",
|
||||
" ),\n",
|
||||
" (\"human\", \"{input}\"),\n",
|
||||
" ]\n",
|
||||
")\n",
|
||||
"\n",
|
||||
"chain = prompt | llm\n",
|
||||
"chain.invoke(\n",
|
||||
" {\n",
|
||||
" \"input_language\": \"English\",\n",
|
||||
" \"output_language\": \"German\",\n",
|
||||
" \"input\": \"I love programming.\",\n",
|
||||
" }\n",
|
||||
")"
|
||||
]
|
||||
},
|
||||
{
|
||||
"cell_type": "markdown",
|
||||
"id": "d1ee55bc-ffc8-4cfa-801c-993953a08cfd",
|
||||
"metadata": {},
|
||||
"source": [
|
||||
"## Structured Outputs"
|
||||
]
|
||||
},
|
||||
{
|
||||
"cell_type": "code",
|
||||
"execution_count": 22,
|
||||
"id": "91cae406-14d7-46c9-b942-2d1476588423",
|
||||
"metadata": {},
|
||||
"outputs": [
|
||||
{
|
||||
"data": {
|
||||
"text/plain": [
|
||||
"{'setup': 'Why did the cat join a band?',\n",
|
||||
" 'punchline': 'Because it wanted to be the purr-cussionist',\n",
|
||||
" 'rating': '8'}"
|
||||
]
|
||||
},
|
||||
"execution_count": 22,
|
||||
"metadata": {},
|
||||
"output_type": "execute_result"
|
||||
}
|
||||
],
|
||||
"source": [
|
||||
"json_schema = {\n",
|
||||
" \"title\": \"joke\",\n",
|
||||
" \"description\": \"Joke to tell user.\",\n",
|
||||
" \"type\": \"object\",\n",
|
||||
" \"properties\": {\n",
|
||||
" \"setup\": {\n",
|
||||
" \"type\": \"string\",\n",
|
||||
" \"description\": \"The setup of the joke\",\n",
|
||||
" },\n",
|
||||
" \"punchline\": {\n",
|
||||
" \"type\": \"string\",\n",
|
||||
" \"description\": \"The punchline to the joke\",\n",
|
||||
" },\n",
|
||||
" \"rating\": {\n",
|
||||
" \"type\": \"integer\",\n",
|
||||
" \"description\": \"How funny the joke is, from 1 to 10\",\n",
|
||||
" \"default\": None,\n",
|
||||
" },\n",
|
||||
" },\n",
|
||||
" \"required\": [\"setup\", \"punchline\"],\n",
|
||||
"}\n",
|
||||
"structured_llm = llm.with_structured_output(json_schema)\n",
|
||||
"\n",
|
||||
"structured_llm.invoke(\"Tell me a joke about cats\")"
|
||||
]
|
||||
},
|
||||
{
|
||||
"cell_type": "markdown",
|
||||
"id": "dbfc0c43-e76b-446e-bbb1-d351640bb7be",
|
||||
"metadata": {},
|
||||
"source": [
|
||||
"## Bind tools"
|
||||
]
|
||||
},
|
||||
{
|
||||
"cell_type": "code",
|
||||
"execution_count": 36,
|
||||
"id": "0765265e-4d00-4030-bf48-7e8d8c9af2ec",
|
||||
"metadata": {},
|
||||
"outputs": [
|
||||
{
|
||||
"data": {
|
||||
"text/plain": [
|
||||
"[{'name': 'validate_user',\n",
|
||||
" 'args': {'user_id': '123',\n",
|
||||
" 'addresses': '[\"123 Fake St in Boston MA\", \"234 Pretend Boulevard in Houston TX\"]'},\n",
|
||||
" 'id': '31ec7d6a-9ce5-471b-be64-8ea0492d1387',\n",
|
||||
" 'type': 'tool_call'}]"
|
||||
]
|
||||
},
|
||||
"execution_count": 36,
|
||||
"metadata": {},
|
||||
"output_type": "execute_result"
|
||||
}
|
||||
],
|
||||
"source": [
|
||||
"from typing import List\n",
|
||||
"\n",
|
||||
"from langchain_core.tools import tool\n",
|
||||
"\n",
|
||||
"\n",
|
||||
"@tool\n",
|
||||
"def validate_user(user_id: int, addresses: List[str]) -> bool:\n",
|
||||
" \"\"\"Validate user using historical addresses.\n",
|
||||
"\n",
|
||||
" Args:\n",
|
||||
" user_id (int): the user ID.\n",
|
||||
" addresses (List[str]): Previous addresses as a list of strings.\n",
|
||||
" \"\"\"\n",
|
||||
" return True\n",
|
||||
"\n",
|
||||
"\n",
|
||||
"llm_with_tools = llm.bind_tools([validate_user])\n",
|
||||
"\n",
|
||||
"result = llm_with_tools.invoke(\n",
|
||||
" \"Could you validate user 123? They previously lived at \"\n",
|
||||
" \"123 Fake St in Boston MA and 234 Pretend Boulevard in \"\n",
|
||||
" \"Houston TX.\"\n",
|
||||
")\n",
|
||||
"result.tool_calls"
|
||||
]
|
||||
},
|
||||
{
|
||||
"cell_type": "markdown",
|
||||
"id": "3a5bb5ca-c3ae-4a58-be67-2cd18574b9a3",
|
||||
"metadata": {},
|
||||
"source": [
|
||||
"## API reference\n",
|
||||
"\n",
|
||||
"https://developers.cloudflare.com/workers-ai/\n",
|
||||
"https://developers.cloudflare.com/agents/"
|
||||
]
|
||||
}
|
||||
],
|
||||
"metadata": {
|
||||
"kernelspec": {
|
||||
"display_name": "Python 3 (ipykernel)",
|
||||
"language": "python",
|
||||
"name": "python3"
|
||||
},
|
||||
"language_info": {
|
||||
"codemirror_mode": {
|
||||
"name": "ipython",
|
||||
"version": 3
|
||||
},
|
||||
"file_extension": ".py",
|
||||
"mimetype": "text/x-python",
|
||||
"name": "python",
|
||||
"nbconvert_exporter": "python",
|
||||
"pygments_lexer": "ipython3",
|
||||
"version": "3.11.7"
|
||||
}
|
||||
},
|
||||
"nbformat": 4,
|
||||
"nbformat_minor": 5
|
||||
}
|
||||
|
File diff suppressed because one or more lines are too long
@ -3,7 +3,9 @@
|
||||
{
|
||||
"cell_type": "raw",
|
||||
"id": "59148044",
|
||||
"metadata": {},
|
||||
"metadata": {
|
||||
"id": "59148044"
|
||||
},
|
||||
"source": [
|
||||
"---\n",
|
||||
"sidebar_label: LiteLLM\n",
|
||||
@ -11,120 +13,223 @@
|
||||
]
|
||||
},
|
||||
{
|
||||
"attachments": {},
|
||||
"cell_type": "markdown",
|
||||
"id": "bf733a38-db84-4363-89e2-de6735c37230",
|
||||
"id": "5bcea387",
|
||||
"metadata": {
|
||||
"id": "5bcea387"
|
||||
},
|
||||
"source": [
|
||||
"# ChatLiteLLM and ChatLiteLLMRouter\n",
|
||||
"\n",
|
||||
"[LiteLLM](https://github.com/BerriAI/litellm) is a library that simplifies calling Anthropic, Azure, Huggingface, Replicate, etc.\n",
|
||||
"\n",
|
||||
"This notebook covers how to get started with using Langchain + the LiteLLM I/O library.\n",
|
||||
"\n",
|
||||
"This integration contains two main classes:\n",
|
||||
"\n",
|
||||
"- ```ChatLiteLLM```: The main Langchain wrapper for basic usage of LiteLLM ([docs](https://docs.litellm.ai/docs/)).\n",
|
||||
"- ```ChatLiteLLMRouter```: A ```ChatLiteLLM``` wrapper that leverages LiteLLM's Router ([docs](https://docs.litellm.ai/docs/routing))."
|
||||
]
|
||||
},
|
||||
{
|
||||
"cell_type": "markdown",
|
||||
"id": "2ddb7fd3",
|
||||
"metadata": {},
|
||||
"source": [
|
||||
"# ChatLiteLLM\n",
|
||||
"## Table of Contents\n",
|
||||
"1. [Overview](#overview)\n",
|
||||
" - [Integration Details](#integration-details)\n",
|
||||
" - [Model Features](#model-features)\n",
|
||||
"2. [Setup](#setup)\n",
|
||||
"3. [Credentials](#credentials)\n",
|
||||
"4. [Installation](#installation)\n",
|
||||
"5. [Instantiation](#instantiation)\n",
|
||||
" - [ChatLiteLLM](#chatlitellm)\n",
|
||||
" - [ChatLiteLLMRouter](#chatlitellmrouter)\n",
|
||||
"6. [Invocation](#invocation)\n",
|
||||
"7. [Async and Streaming Functionality](#async-and-streaming-functionality)\n",
|
||||
"8. [API Reference](#api-reference)"
|
||||
]
|
||||
},
|
||||
{
|
||||
"cell_type": "markdown",
|
||||
"id": "37be6ef8",
|
||||
"metadata": {},
|
||||
"source": [
|
||||
"## Overview\n",
|
||||
"### Integration details\n",
|
||||
"\n",
|
||||
"[LiteLLM](https://github.com/BerriAI/litellm) is a library that simplifies calling Anthropic, Azure, Huggingface, Replicate, etc. \n",
|
||||
"| Class | Package | Local | Serializable | JS support| Package downloads | Package latest |\n",
|
||||
"| :--- | :--- | :---: | :---: | :---: | :---: | :---: |\n",
|
||||
"| [ChatLiteLLM](https://python.langchain.com/docs/integrations/chat/litellm/#chatlitellm) | [langchain-litellm](https://pypi.org/project/langchain-litellm/)| ❌ | ❌ | ❌ |  |  |\n",
|
||||
"| [ChatLiteLLMRouter](https://python.langchain.com/docs/integrations/chat/litellm/#chatlitellmrouter) | [langchain-litellm](https://pypi.org/project/langchain-litellm/)| ❌ | ❌ | ❌ |  |  |\n",
|
||||
"\n",
|
||||
"This notebook covers how to get started with using Langchain + the LiteLLM I/O library. "
|
||||
"### Model features\n",
|
||||
"| [Tool calling](https://python.langchain.com/docs/how_to/tool_calling/) | [Structured output](https://python.langchain.com/docs/how_to/structured_output/) | JSON mode | Image input | Audio input | Video input | [Token-level streaming](https://python.langchain.com/docs/integrations/chat/litellm/#chatlitellm-also-supports-async-and-streaming-functionality) | [Native async](https://python.langchain.com/docs/integrations/chat/litellm/#chatlitellm-also-supports-async-and-streaming-functionality) | [Token usage](https://python.langchain.com/docs/how_to/chat_token_usage_tracking/) | [Logprobs](https://python.langchain.com/docs/how_to/logprobs/) |\n",
|
||||
"| :---: | :---: | :---: | :---: | :---: | :---: | :---: | :---: | :---: | :---: |\n",
|
||||
"| ✅ | ❌ | ❌ | ❌ | ❌ | ❌ | ✅ | ✅ | ✅ | ❌ |\n",
|
||||
"\n",
|
||||
"### Setup\n",
|
||||
"To access ```ChatLiteLLM``` and ```ChatLiteLLMRouter``` models, you'll need to install the `langchain-litellm` package and create an OpenAI, Anthropic, Azure, Replicate, OpenRouter, Hugging Face, Together AI, or Cohere account. Then, you have to get an API key and export it as an environment variable."
|
||||
]
|
||||
},
|
||||
{
|
||||
"cell_type": "markdown",
|
||||
"id": "0a2f8164",
|
||||
"metadata": {
|
||||
"id": "0a2f8164"
|
||||
},
|
||||
"source": [
|
||||
"## Credentials\n",
|
||||
"\n",
|
||||
"You have to choose the LLM provider you want and sign up with them to get their API key.\n",
|
||||
"\n",
|
||||
"### Example - Anthropic\n",
|
||||
"Head to https://console.anthropic.com/ to sign up for Anthropic and generate an API key. Once you've done this, set the ANTHROPIC_API_KEY environment variable.\n",
|
||||
"\n",
|
||||
"\n",
|
||||
"### Example - OpenAI\n",
|
||||
"Head to https://platform.openai.com/api-keys to sign up for OpenAI and generate an API key. Once you've done this, set the OPENAI_API_KEY environment variable."
|
||||
]
|
||||
},
|
||||
{
|
||||
"cell_type": "code",
|
||||
"execution_count": 1,
|
||||
"id": "d4a7c55d-b235-4ca4-a579-c90cc9570da9",
|
||||
"execution_count": null,
|
||||
"id": "7595eddf",
|
||||
"metadata": {
|
||||
"tags": []
|
||||
"id": "7595eddf"
|
||||
},
|
||||
"outputs": [],
|
||||
"source": [
|
||||
"from langchain_community.chat_models import ChatLiteLLM\n",
|
||||
"from langchain_core.messages import HumanMessage"
|
||||
"## Set ENV variables\n",
|
||||
"import os\n",
|
||||
"\n",
|
||||
"os.environ[\"OPENAI_API_KEY\"] = \"your-openai-key\"\n",
|
||||
"os.environ[\"ANTHROPIC_API_KEY\"] = \"your-anthropic-key\""
|
||||
]
|
||||
},
|
||||
{
|
||||
"cell_type": "markdown",
|
||||
"id": "74c3ad30",
|
||||
"metadata": {
|
||||
"id": "74c3ad30"
|
||||
},
|
||||
"source": [
|
||||
"### Installation\n",
|
||||
"\n",
|
||||
"The LangChain LiteLLM integration is available in the `langchain-litellm` package:"
|
||||
]
|
||||
},
|
||||
{
|
||||
"cell_type": "code",
|
||||
"execution_count": 2,
|
||||
"id": "70cf04e8-423a-4ff6-8b09-f11fb711c817",
|
||||
"id": "ca3f8a25",
|
||||
"metadata": {
|
||||
"tags": []
|
||||
"id": "ca3f8a25"
|
||||
},
|
||||
"outputs": [],
|
||||
"source": [
|
||||
"chat = ChatLiteLLM(model=\"gpt-3.5-turbo\")"
|
||||
"%pip install -qU langchain-litellm"
|
||||
]
|
||||
},
|
||||
{
|
||||
"cell_type": "markdown",
|
||||
"id": "bc1182b4",
|
||||
"metadata": {
|
||||
"id": "bc1182b4"
|
||||
},
|
||||
"source": [
|
||||
"## Instantiation"
|
||||
]
|
||||
},
|
||||
{
|
||||
"cell_type": "markdown",
|
||||
"id": "d439241a",
|
||||
"metadata": {},
|
||||
"source": [
|
||||
"### ChatLiteLLM\n",
|
||||
"You can instantiate a ```ChatLiteLLM``` model by providing a ```model``` name [supported by LiteLLM](https://docs.litellm.ai/docs/providers)."
|
||||
]
|
||||
},
|
||||
{
|
||||
"cell_type": "code",
|
||||
"execution_count": 3,
|
||||
"id": "8199ef8f-eb8b-4253-9ea0-6c24a013ca4c",
|
||||
"execution_count": null,
|
||||
"id": "d4a7c55d-b235-4ca4-a579-c90cc9570da9",
|
||||
"metadata": {
|
||||
"id": "d4a7c55d-b235-4ca4-a579-c90cc9570da9",
|
||||
"tags": []
|
||||
},
|
||||
"outputs": [
|
||||
{
|
||||
"data": {
|
||||
"text/plain": [
|
||||
"AIMessage(content=\" J'aime la programmation.\", additional_kwargs={}, example=False)"
|
||||
]
|
||||
},
|
||||
"execution_count": 3,
|
||||
"metadata": {},
|
||||
"output_type": "execute_result"
|
||||
}
|
||||
],
|
||||
"outputs": [],
|
||||
"source": [
|
||||
"messages = [\n",
|
||||
" HumanMessage(\n",
|
||||
" content=\"Translate this sentence from English to French. I love programming.\"\n",
|
||||
" )\n",
|
||||
"]\n",
|
||||
"chat(messages)"
|
||||
"from langchain_litellm import ChatLiteLLM\n",
|
||||
"\n",
|
||||
"llm = ChatLiteLLM(model=\"gpt-4.1-nano\", temperature=0.1)"
|
||||
]
|
||||
},
|
||||
{
|
||||
"attachments": {},
|
||||
"cell_type": "markdown",
|
||||
"id": "c361ab1e-8c0c-4206-9e3c-9d1424a12b9c",
|
||||
"id": "3d0ed306",
|
||||
"metadata": {},
|
||||
"source": [
|
||||
"## `ChatLiteLLM` also supports async and streaming functionality:"
|
||||
"### ChatLiteLLMRouter\n",
|
||||
"You can also leverage LiteLLM's routing capabilities by defining your model list as specified [here](https://docs.litellm.ai/docs/routing)."
|
||||
]
|
||||
},
|
||||
{
|
||||
"cell_type": "code",
|
||||
"execution_count": null,
|
||||
"id": "8d26393a",
|
||||
"metadata": {},
|
||||
"outputs": [],
|
||||
"source": [
|
||||
"from langchain_litellm import ChatLiteLLMRouter\n",
|
||||
"from litellm import Router\n",
|
||||
"\n",
|
||||
"model_list = [\n",
|
||||
" {\n",
|
||||
" \"model_name\": \"gpt-4.1\",\n",
|
||||
" \"litellm_params\": {\n",
|
||||
" \"model\": \"azure/gpt-4.1\",\n",
|
||||
" \"api_key\": \"<your-api-key>\",\n",
|
||||
" \"api_version\": \"2024-10-21\",\n",
|
||||
" \"api_base\": \"https://<your-endpoint>.openai.azure.com/\",\n",
|
||||
" },\n",
|
||||
" },\n",
|
||||
" {\n",
|
||||
" \"model_name\": \"gpt-4o\",\n",
|
||||
" \"litellm_params\": {\n",
|
||||
" \"model\": \"azure/gpt-4o\",\n",
|
||||
" \"api_key\": \"<your-api-key>\",\n",
|
||||
" \"api_version\": \"2024-10-21\",\n",
|
||||
" \"api_base\": \"https://<your-endpoint>.openai.azure.com/\",\n",
|
||||
" },\n",
|
||||
" },\n",
|
||||
"]\n",
|
||||
"litellm_router = Router(model_list=model_list)\n",
|
||||
"llm = ChatLiteLLMRouter(router=litellm_router, model_name=\"gpt-4.1\", temperature=0.1)"
|
||||
]
|
||||
},
|
||||
{
|
||||
"cell_type": "markdown",
|
||||
"id": "63d98454",
|
||||
"metadata": {
|
||||
"id": "63d98454"
|
||||
},
|
||||
"source": [
|
||||
"## Invocation\n",
|
||||
"Whether you've instantiated a `ChatLiteLLM` or a `ChatLiteLLMRouter`, you can now use the ChatModel through Langchain's API."
|
||||
]
|
||||
},
|
||||
{
|
||||
"cell_type": "code",
|
||||
"execution_count": 4,
|
||||
"id": "93a21c5c-6ef9-4688-be60-b2e1f94842fb",
|
||||
"metadata": {
|
||||
"tags": []
|
||||
},
|
||||
"outputs": [],
|
||||
"source": [
|
||||
"from langchain_core.callbacks import CallbackManager, StreamingStdOutCallbackHandler"
|
||||
]
|
||||
},
|
||||
{
|
||||
"cell_type": "code",
|
||||
"execution_count": 5,
|
||||
"id": "c5fac0e9-05a4-4fc1-a3b3-e5bbb24b971b",
|
||||
"metadata": {
|
||||
"tags": []
|
||||
},
|
||||
"outputs": [
|
||||
{
|
||||
"data": {
|
||||
"text/plain": [
|
||||
"LLMResult(generations=[[ChatGeneration(text=\" J'aime programmer.\", generation_info=None, message=AIMessage(content=\" J'aime programmer.\", additional_kwargs={}, example=False))]], llm_output={}, run=[RunInfo(run_id=UUID('8cc8fb68-1c35-439c-96a0-695036a93652'))])"
|
||||
]
|
||||
},
|
||||
"execution_count": 5,
|
||||
"metadata": {},
|
||||
"output_type": "execute_result"
|
||||
}
|
||||
],
|
||||
"source": [
|
||||
"await chat.agenerate([messages])"
|
||||
]
|
||||
},
|
||||
{
|
||||
"cell_type": "code",
|
||||
"execution_count": 6,
|
||||
"id": "025be980-e50d-4a68-93dc-c9c7b500ce34",
|
||||
"id": "8199ef8f-eb8b-4253-9ea0-6c24a013ca4c",
|
||||
"metadata": {
|
||||
"colab": {
|
||||
"base_uri": "https://localhost:8080/"
|
||||
},
|
||||
"id": "8199ef8f-eb8b-4253-9ea0-6c24a013ca4c",
|
||||
"outputId": "a4c0e5f5-a859-43fa-dd78-74fc0922ecb2",
|
||||
"tags": []
|
||||
},
|
||||
"outputs": [
|
||||
@ -132,41 +237,76 @@
|
||||
"name": "stdout",
|
||||
"output_type": "stream",
|
||||
"text": [
|
||||
" J'aime la programmation."
|
||||
"content='Neutral' additional_kwargs={} response_metadata={'token_usage': Usage(completion_tokens=2, prompt_tokens=30, total_tokens=32, completion_tokens_details=CompletionTokensDetailsWrapper(accepted_prediction_tokens=0, audio_tokens=0, reasoning_tokens=0, rejected_prediction_tokens=0, text_tokens=None), prompt_tokens_details=PromptTokensDetailsWrapper(audio_tokens=0, cached_tokens=0, text_tokens=None, image_tokens=None)), 'model': 'gpt-3.5-turbo', 'finish_reason': 'stop', 'model_name': 'gpt-3.5-turbo'} id='run-ab6a3b21-eae8-4c27-acb2-add65a38221a-0' usage_metadata={'input_tokens': 30, 'output_tokens': 2, 'total_tokens': 32}\n"
|
||||
]
|
||||
},
|
||||
{
|
||||
"data": {
|
||||
"text/plain": [
|
||||
"AIMessage(content=\" J'aime la programmation.\", additional_kwargs={}, example=False)"
|
||||
]
|
||||
},
|
||||
"execution_count": 6,
|
||||
"metadata": {},
|
||||
"output_type": "execute_result"
|
||||
}
|
||||
],
|
||||
"source": [
|
||||
"chat = ChatLiteLLM(\n",
|
||||
" streaming=True,\n",
|
||||
" verbose=True,\n",
|
||||
" callback_manager=CallbackManager([StreamingStdOutCallbackHandler()]),\n",
|
||||
"response = await llm.ainvoke(\n",
|
||||
" \"Classify the text into neutral, negative or positive. Text: I think the food was okay. Sentiment:\"\n",
|
||||
")\n",
|
||||
"chat(messages)"
|
||||
"print(response)"
|
||||
]
|
||||
},
|
||||
{
|
||||
"cell_type": "markdown",
|
||||
"id": "c361ab1e-8c0c-4206-9e3c-9d1424a12b9c",
|
||||
"metadata": {
|
||||
"id": "c361ab1e-8c0c-4206-9e3c-9d1424a12b9c"
|
||||
},
|
||||
"source": [
|
||||
"## Async and Streaming Functionality\n",
|
||||
"`ChatLiteLLM` and `ChatLiteLLMRouter` also support async and streaming functionality:"
|
||||
]
|
||||
},
|
||||
{
|
||||
"cell_type": "code",
|
||||
"execution_count": null,
|
||||
"id": "c253883f",
|
||||
"metadata": {},
|
||||
"outputs": [],
|
||||
"source": []
|
||||
"execution_count": 5,
|
||||
"id": "c5fac0e9-05a4-4fc1-a3b3-e5bbb24b971b",
|
||||
"metadata": {
|
||||
"colab": {
|
||||
"base_uri": "https://localhost:8080/"
|
||||
},
|
||||
"id": "c5fac0e9-05a4-4fc1-a3b3-e5bbb24b971b",
|
||||
"outputId": "ee8cdda1-d992-4696-9ad0-aa146360a3ee",
|
||||
"tags": []
|
||||
},
|
||||
"outputs": [
|
||||
{
|
||||
"name": "stdout",
|
||||
"output_type": "stream",
|
||||
"text": [
|
||||
"Antibiotics are medications that fight bacterial infections in the body. They work by targeting specific bacteria and either killing them or preventing their growth and reproduction.\n",
|
||||
"\n",
|
||||
"There are several different mechanisms by which antibiotics work. Some antibiotics work by disrupting the cell walls of bacteria, causing them to burst and die. Others interfere with the protein synthesis of bacteria, preventing them from growing and reproducing. Some antibiotics target the DNA or RNA of bacteria, disrupting their ability to replicate.\n",
|
||||
"\n",
|
||||
"It is important to note that antibiotics only work against bacterial infections and not viral infections. It is also crucial to take antibiotics as prescribed by a healthcare professional and to complete the full course of treatment, even if symptoms improve before the medication is finished. This helps to prevent antibiotic resistance, where bacteria become resistant to the effects of antibiotics."
|
||||
]
|
||||
}
|
||||
],
|
||||
"source": [
|
||||
"async for token in llm.astream(\"Hello, please explain how antibiotics work\"):\n",
|
||||
" print(token.text(), end=\"\")"
|
||||
]
|
||||
},
|
||||
{
|
||||
"cell_type": "markdown",
|
||||
"id": "88af2a9b",
|
||||
"metadata": {
|
||||
"id": "88af2a9b"
|
||||
},
|
||||
"source": [
|
||||
"## API reference\n",
|
||||
"For detailed documentation of all `ChatLiteLLM` and `ChatLiteLLMRouter` features and configurations, head to the API reference: https://github.com/Akshay-Dongare/langchain-litellm"
|
||||
]
|
||||
}
|
||||
],
|
||||
"metadata": {
|
||||
"colab": {
|
||||
"provenance": []
|
||||
},
|
||||
"kernelspec": {
|
||||
"display_name": "Python 3 (ipykernel)",
|
||||
"display_name": "g6_alda",
|
||||
"language": "python",
|
||||
"name": "python3"
|
||||
},
|
||||
@ -180,7 +320,7 @@
|
||||
"name": "python",
|
||||
"nbconvert_exporter": "python",
|
||||
"pygments_lexer": "ipython3",
|
||||
"version": "3.9.1"
|
||||
"version": "3.12.4"
|
||||
}
|
||||
},
|
||||
"nbformat": 4,
|
||||
|
@ -1,218 +0,0 @@
|
||||
{
|
||||
"cells": [
|
||||
{
|
||||
"cell_type": "raw",
|
||||
"id": "59148044",
|
||||
"metadata": {},
|
||||
"source": [
|
||||
"---\n",
|
||||
"sidebar_label: LiteLLM Router\n",
|
||||
"---"
|
||||
]
|
||||
},
|
||||
{
|
||||
"cell_type": "markdown",
|
||||
"id": "247da7a6",
|
||||
"metadata": {},
|
||||
"source": []
|
||||
},
|
||||
{
|
||||
"attachments": {},
|
||||
"cell_type": "markdown",
|
||||
"id": "bf733a38-db84-4363-89e2-de6735c37230",
|
||||
"metadata": {},
|
||||
"source": [
|
||||
"# ChatLiteLLMRouter\n",
|
||||
"\n",
|
||||
"[LiteLLM](https://github.com/BerriAI/litellm) is a library that simplifies calling Anthropic, Azure, Huggingface, Replicate, etc. \n",
|
||||
"\n",
|
||||
"This notebook covers how to get started with using Langchain + the LiteLLM Router I/O library. "
|
||||
]
|
||||
},
|
||||
{
|
||||
"cell_type": "code",
|
||||
"execution_count": 1,
|
||||
"id": "d4a7c55d-b235-4ca4-a579-c90cc9570da9",
|
||||
"metadata": {
|
||||
"tags": []
|
||||
},
|
||||
"outputs": [],
|
||||
"source": [
|
||||
"from langchain_community.chat_models import ChatLiteLLMRouter\n",
|
||||
"from langchain_core.messages import HumanMessage\n",
|
||||
"from litellm import Router"
|
||||
]
|
||||
},
|
||||
{
|
||||
"cell_type": "code",
|
||||
"execution_count": 2,
|
||||
"id": "70cf04e8-423a-4ff6-8b09-f11fb711c817",
|
||||
"metadata": {
|
||||
"tags": []
|
||||
},
|
||||
"outputs": [],
|
||||
"source": [
|
||||
"model_list = [\n",
|
||||
" {\n",
|
||||
" \"model_name\": \"gpt-4\",\n",
|
||||
" \"litellm_params\": {\n",
|
||||
" \"model\": \"azure/gpt-4-1106-preview\",\n",
|
||||
" \"api_key\": \"<your-api-key>\",\n",
|
||||
" \"api_version\": \"2023-05-15\",\n",
|
||||
" \"api_base\": \"https://<your-endpoint>.openai.azure.com/\",\n",
|
||||
" },\n",
|
||||
" },\n",
|
||||
" {\n",
|
||||
" \"model_name\": \"gpt-35-turbo\",\n",
|
||||
" \"litellm_params\": {\n",
|
||||
" \"model\": \"azure/gpt-35-turbo\",\n",
|
||||
" \"api_key\": \"<your-api-key>\",\n",
|
||||
" \"api_version\": \"2023-05-15\",\n",
|
||||
" \"api_base\": \"https://<your-endpoint>.openai.azure.com/\",\n",
|
||||
" },\n",
|
||||
" },\n",
|
||||
"]\n",
|
||||
"litellm_router = Router(model_list=model_list)\n",
|
||||
"chat = ChatLiteLLMRouter(router=litellm_router, model_name=\"gpt-35-turbo\")"
|
||||
]
|
||||
},
|
||||
{
|
||||
"cell_type": "code",
|
||||
"execution_count": 3,
|
||||
"id": "8199ef8f-eb8b-4253-9ea0-6c24a013ca4c",
|
||||
"metadata": {
|
||||
"tags": []
|
||||
},
|
||||
"outputs": [
|
||||
{
|
||||
"data": {
|
||||
"text/plain": [
|
||||
"AIMessage(content=\"J'aime programmer.\")"
|
||||
]
|
||||
},
|
||||
"execution_count": 3,
|
||||
"metadata": {},
|
||||
"output_type": "execute_result"
|
||||
}
|
||||
],
|
||||
"source": [
|
||||
"messages = [\n",
|
||||
" HumanMessage(\n",
|
||||
" content=\"Translate this sentence from English to French. I love programming.\"\n",
|
||||
" )\n",
|
||||
"]\n",
|
||||
"chat(messages)"
|
||||
]
|
||||
},
|
||||
{
|
||||
"attachments": {},
|
||||
"cell_type": "markdown",
|
||||
"id": "c361ab1e-8c0c-4206-9e3c-9d1424a12b9c",
|
||||
"metadata": {},
|
||||
"source": [
|
||||
"## `ChatLiteLLMRouter` also supports async and streaming functionality:"
|
||||
]
|
||||
},
|
||||
{
|
||||
"cell_type": "code",
|
||||
"execution_count": 4,
|
||||
"id": "93a21c5c-6ef9-4688-be60-b2e1f94842fb",
|
||||
"metadata": {
|
||||
"tags": []
|
||||
},
|
||||
"outputs": [],
|
||||
"source": [
|
||||
"from langchain_core.callbacks import CallbackManager, StreamingStdOutCallbackHandler"
|
||||
]
|
||||
},
|
||||
{
|
||||
"cell_type": "code",
|
||||
"execution_count": 5,
|
||||
"id": "c5fac0e9-05a4-4fc1-a3b3-e5bbb24b971b",
|
||||
"metadata": {
|
||||
"tags": []
|
||||
},
|
||||
"outputs": [
|
||||
{
|
||||
"data": {
|
||||
"text/plain": [
|
||||
"LLMResult(generations=[[ChatGeneration(text=\"J'adore programmer.\", generation_info={'finish_reason': 'stop'}, message=AIMessage(content=\"J'adore programmer.\"))]], llm_output={'token_usage': {'completion_tokens': 6, 'prompt_tokens': 19, 'total_tokens': 25}, 'model_name': None}, run=[RunInfo(run_id=UUID('75003ec9-1e2b-43b7-a216-10dcc0f75e00'))])"
|
||||
]
|
||||
},
|
||||
"execution_count": 5,
|
||||
"metadata": {},
|
||||
"output_type": "execute_result"
|
||||
}
|
||||
],
|
||||
"source": [
|
||||
"await chat.agenerate([messages])"
|
||||
]
|
||||
},
|
||||
{
|
||||
"cell_type": "code",
|
||||
"execution_count": 6,
|
||||
"id": "025be980-e50d-4a68-93dc-c9c7b500ce34",
|
||||
"metadata": {
|
||||
"tags": []
|
||||
},
|
||||
"outputs": [
|
||||
{
|
||||
"name": "stdout",
|
||||
"output_type": "stream",
|
||||
"text": [
|
||||
"J'adore programmer."
|
||||
]
|
||||
},
|
||||
{
|
||||
"data": {
|
||||
"text/plain": [
|
||||
"AIMessage(content=\"J'adore programmer.\")"
|
||||
]
|
||||
},
|
||||
"execution_count": 6,
|
||||
"metadata": {},
|
||||
"output_type": "execute_result"
|
||||
}
|
||||
],
|
||||
"source": [
|
||||
"chat = ChatLiteLLMRouter(\n",
|
||||
" router=litellm_router,\n",
|
||||
" model_name=\"gpt-35-turbo\",\n",
|
||||
" streaming=True,\n",
|
||||
" verbose=True,\n",
|
||||
" callback_manager=CallbackManager([StreamingStdOutCallbackHandler()]),\n",
|
||||
")\n",
|
||||
"chat(messages)"
|
||||
]
|
||||
},
|
||||
{
|
||||
"cell_type": "code",
|
||||
"execution_count": null,
|
||||
"id": "c253883f",
|
||||
"metadata": {},
|
||||
"outputs": [],
|
||||
"source": []
|
||||
}
|
||||
],
|
||||
"metadata": {
|
||||
"kernelspec": {
|
||||
"display_name": "Python 3 (ipykernel)",
|
||||
"language": "python",
|
||||
"name": "python3"
|
||||
},
|
||||
"language_info": {
|
||||
"codemirror_mode": {
|
||||
"name": "ipython",
|
||||
"version": 3
|
||||
},
|
||||
"file_extension": ".py",
|
||||
"mimetype": "text/x-python",
|
||||
"name": "python",
|
||||
"nbconvert_exporter": "python",
|
||||
"pygments_lexer": "ipython3",
|
||||
"version": "3.11.9"
|
||||
}
|
||||
},
|
||||
"nbformat": 4,
|
||||
"nbformat_minor": 5
|
||||
}
|
@ -17,21 +17,21 @@
|
||||
"source": [
|
||||
"# ChatClovaX\n",
|
||||
"\n",
|
||||
"This notebook provides a quick overview for getting started with Naver’s HyperCLOVA X [chat models](https://python.langchain.com/docs/concepts/chat_models) via CLOVA Studio. For detailed documentation of all ChatClovaX features and configurations head to the [API reference](https://python.langchain.com/api_reference/community/chat_models/langchain_community.chat_models.naver.ChatClovaX.html).\n",
|
||||
"This notebook provides a quick overview for getting started with Naver’s HyperCLOVA X [chat models](https://python.langchain.com/docs/concepts/chat_models) via CLOVA Studio. For detailed documentation of all ChatClovaX features and configurations head to the [API reference](https://guide.ncloud-docs.com/docs/clovastudio-dev-langchain).\n",
|
||||
"\n",
|
||||
"[CLOVA Studio](http://clovastudio.ncloud.com/) has several chat models. You can find information about latest models and their costs, context windows, and supported input types in the CLOVA Studio API Guide [documentation](https://api.ncloud-docs.com/docs/clovastudio-chatcompletions).\n",
|
||||
"[CLOVA Studio](http://clovastudio.ncloud.com/) has several chat models. You can find information about latest models and their costs, context windows, and supported input types in the CLOVA Studio Guide [documentation](https://guide.ncloud-docs.com/docs/clovastudio-model).\n",
|
||||
"\n",
|
||||
"## Overview\n",
|
||||
"### Integration details\n",
|
||||
"\n",
|
||||
"| Class | Package | Local | Serializable | JS support | Package downloads | Package latest |\n",
|
||||
"| :--- | :--- |:-----:| :---: |:------------------------------------------------------------------------:| :---: | :---: |\n",
|
||||
"| [ChatClovaX](https://python.langchain.com/api_reference/community/chat_models/langchain_community.chat_models.naver.ChatClovaX.html) | [langchain-community](https://python.langchain.com/api_reference/community/index.html) | ❌ | ❌ | ❌ |  |  |\n",
|
||||
"| [ChatClovaX](https://guide.ncloud-docs.com/docs/clovastudio-dev-langchain#HyperCLOVAX%EB%AA%A8%EB%8D%B8%EC%9D%B4%EC%9A%A9) | [langchain-naver](https://pypi.org/project/langchain-naver/) | ❌ | ❌ | ❌ |  |  |\n",
|
||||
"\n",
|
||||
"### Model features\n",
|
||||
"| [Tool calling](/docs/how_to/tool_calling/) | [Structured output](/docs/how_to/structured_output/) | JSON mode | [Image input](/docs/how_to/multimodal_inputs/) | Audio input | Video input | [Token-level streaming](/docs/how_to/chat_streaming/) | Native async | [Token usage](/docs/how_to/chat_token_usage_tracking/) | [Logprobs](/docs/how_to/logprobs/) |\n",
|
||||
"|:------------------------------------------:| :---: | :---: | :---: | :---: | :---: |:-----------------------------------------------------:| :---: |:------------------------------------------------------:|:----------------------------------:|\n",
|
||||
"|❌| ❌ | ❌ | ❌ | ❌ | ❌ | ✅ | ✅ | ✅ | ❌ |\n",
|
||||
"|✅| ❌ | ❌ | ✅ | ❌ | ❌ | ✅ | ✅ | ✅ | ❌ |\n",
|
||||
"\n",
|
||||
"## Setup\n",
|
||||
"\n",
|
||||
@ -39,26 +39,23 @@
|
||||
"\n",
|
||||
"1. Creating [NAVER Cloud Platform](https://www.ncloud.com/) account\n",
|
||||
"2. Apply to use [CLOVA Studio](https://www.ncloud.com/product/aiService/clovaStudio)\n",
|
||||
"3. Create a CLOVA Studio Test App or Service App of a model to use (See [here](https://guide.ncloud-docs.com/docs/en/clovastudio-playground01#테스트앱생성).)\n",
|
||||
"3. Create a CLOVA Studio Test App or Service App of a model to use (See [here](https://guide.ncloud-docs.com/docs/clovastudio-playground-testapp).)\n",
|
||||
"4. Issue a Test or Service API key (See [here](https://api.ncloud-docs.com/docs/ai-naver-clovastudio-summary#API%ED%82%A4).)\n",
|
||||
"\n",
|
||||
"### Credentials\n",
|
||||
"\n",
|
||||
"Set the `NCP_CLOVASTUDIO_API_KEY` environment variable with your API key.\n",
|
||||
" - Note that if you are using a legacy API Key (that doesn't start with `nv-*` prefix), you might need to get an additional API Key by clicking `App Request Status` > `Service App, Test App List` > `‘Details’ button for each app` in [CLOVA Studio](https://clovastudio.ncloud.com/studio-application/service-app) and set it as `NCP_APIGW_API_KEY`.\n",
|
||||
"Set the `CLOVASTUDIO_API_KEY` environment variable with your API key.\n",
|
||||
"\n",
|
||||
"You can add them to your environment variables as below:\n",
|
||||
"\n",
|
||||
"``` bash\n",
|
||||
"export NCP_CLOVASTUDIO_API_KEY=\"your-api-key-here\"\n",
|
||||
"# Uncomment below to use a legacy API key\n",
|
||||
"# export NCP_APIGW_API_KEY=\"your-api-key-here\"\n",
|
||||
"export CLOVASTUDIO_API_KEY=\"your-api-key-here\"\n",
|
||||
"```"
|
||||
]
|
||||
},
|
||||
{
|
||||
"cell_type": "code",
|
||||
"execution_count": null,
|
||||
"execution_count": 2,
|
||||
"id": "2def81b5-b023-4f40-a97b-b2c5ca59d6a9",
|
||||
"metadata": {},
|
||||
"outputs": [],
|
||||
@ -66,22 +63,19 @@
|
||||
"import getpass\n",
|
||||
"import os\n",
|
||||
"\n",
|
||||
"if not os.getenv(\"NCP_CLOVASTUDIO_API_KEY\"):\n",
|
||||
" os.environ[\"NCP_CLOVASTUDIO_API_KEY\"] = getpass.getpass(\n",
|
||||
" \"Enter your NCP CLOVA Studio API Key: \"\n",
|
||||
" )\n",
|
||||
"# Uncomment below to use a legacy API key\n",
|
||||
"# if not os.getenv(\"NCP_APIGW_API_KEY\"):\n",
|
||||
"# os.environ[\"NCP_APIGW_API_KEY\"] = getpass.getpass(\n",
|
||||
"# \"Enter your NCP API Gateway API key: \"\n",
|
||||
"# )"
|
||||
"if not os.getenv(\"CLOVASTUDIO_API_KEY\"):\n",
|
||||
" os.environ[\"CLOVASTUDIO_API_KEY\"] = getpass.getpass(\n",
|
||||
" \"Enter your CLOVA Studio API Key: \"\n",
|
||||
" )"
|
||||
]
|
||||
},
|
||||
{
|
||||
"cell_type": "markdown",
|
||||
"id": "7c695442",
|
||||
"metadata": {},
|
||||
"source": "To enable automated tracing of your model calls, set your [LangSmith](https://docs.smith.langchain.com/) API key:"
|
||||
"source": [
|
||||
"To enable automated tracing of your model calls, set your [LangSmith](https://docs.smith.langchain.com/) API key:"
|
||||
]
|
||||
},
|
||||
{
|
||||
"cell_type": "code",
|
||||
@ -101,7 +95,7 @@
|
||||
"source": [
|
||||
"### Installation\n",
|
||||
"\n",
|
||||
"The LangChain Naver integration lives in the `langchain-community` package:"
|
||||
"The LangChain Naver integration lives in the `langchain-naver` package:"
|
||||
]
|
||||
},
|
||||
{
|
||||
@ -112,7 +106,7 @@
|
||||
"outputs": [],
|
||||
"source": [
|
||||
"# install package\n",
|
||||
"!pip install -qU langchain-community"
|
||||
"%pip install -qU langchain-naver"
|
||||
]
|
||||
},
|
||||
{
|
||||
@ -127,21 +121,19 @@
|
||||
},
|
||||
{
|
||||
"cell_type": "code",
|
||||
"execution_count": 2,
|
||||
"execution_count": 3,
|
||||
"id": "cb09c344-1836-4e0c-acf8-11d13ac1dbae",
|
||||
"metadata": {},
|
||||
"outputs": [],
|
||||
"source": [
|
||||
"from langchain_community.chat_models import ChatClovaX\n",
|
||||
"from langchain_naver import ChatClovaX\n",
|
||||
"\n",
|
||||
"chat = ChatClovaX(\n",
|
||||
" model=\"HCX-003\",\n",
|
||||
" max_tokens=100,\n",
|
||||
" model=\"HCX-005\",\n",
|
||||
" temperature=0.5,\n",
|
||||
" # clovastudio_api_key=\"...\" # set if you prefer to pass api key directly instead of using environment variables\n",
|
||||
" # task_id=\"...\" # set if you want to use fine-tuned model\n",
|
||||
" # service_app=False # set True if using Service App. Default value is False (means using Test App)\n",
|
||||
" # include_ai_filters=False # set True if you want to detect inappropriate content. Default value is False\n",
|
||||
" max_tokens=None,\n",
|
||||
" timeout=None,\n",
|
||||
" max_retries=2,\n",
|
||||
" # other params...\n",
|
||||
")"
|
||||
]
|
||||
@ -153,12 +145,12 @@
|
||||
"source": [
|
||||
"## Invocation\n",
|
||||
"\n",
|
||||
"In addition to invoke, we also support batch and stream functionalities."
|
||||
"In addition to invoke, `ChatClovaX` also support batch and stream functionalities."
|
||||
]
|
||||
},
|
||||
{
|
||||
"cell_type": "code",
|
||||
"execution_count": 3,
|
||||
"execution_count": 4,
|
||||
"id": "62e0dbc3",
|
||||
"metadata": {
|
||||
"tags": []
|
||||
@ -167,10 +159,10 @@
|
||||
{
|
||||
"data": {
|
||||
"text/plain": [
|
||||
"AIMessage(content='저는 네이버 AI를 사용하는 것이 좋아요.', additional_kwargs={}, response_metadata={'stop_reason': 'stop_before', 'input_length': 25, 'output_length': 14, 'seed': 1112164354, 'ai_filter': None}, id='run-b57bc356-1148-4007-837d-cc409dbd57cc-0', usage_metadata={'input_tokens': 25, 'output_tokens': 14, 'total_tokens': 39})"
|
||||
"AIMessage(content='네이버 인공지능을 사용하는 것을 정말 좋아합니다.', additional_kwargs={'refusal': None}, response_metadata={'token_usage': {'completion_tokens': 11, 'prompt_tokens': 28, 'total_tokens': 39, 'completion_tokens_details': None, 'prompt_tokens_details': None}, 'model_name': 'HCX-005', 'system_fingerprint': None, 'id': 'b70c26671cd247a0864115bacfb5fc12', 'finish_reason': 'stop', 'logprobs': None}, id='run-3faf6a8d-d5da-49ad-9fbb-7b56ed23b484-0', usage_metadata={'input_tokens': 28, 'output_tokens': 11, 'total_tokens': 39, 'input_token_details': {}, 'output_token_details': {}})"
|
||||
]
|
||||
},
|
||||
"execution_count": 3,
|
||||
"execution_count": 4,
|
||||
"metadata": {},
|
||||
"output_type": "execute_result"
|
||||
}
|
||||
@ -190,7 +182,7 @@
|
||||
},
|
||||
{
|
||||
"cell_type": "code",
|
||||
"execution_count": 4,
|
||||
"execution_count": 5,
|
||||
"id": "24e7377f",
|
||||
"metadata": {},
|
||||
"outputs": [
|
||||
@ -198,7 +190,7 @@
|
||||
"name": "stdout",
|
||||
"output_type": "stream",
|
||||
"text": [
|
||||
"저는 네이버 AI를 사용하는 것이 좋아요.\n"
|
||||
"네이버 인공지능을 사용하는 것을 정말 좋아합니다.\n"
|
||||
]
|
||||
}
|
||||
],
|
||||
@ -218,17 +210,17 @@
|
||||
},
|
||||
{
|
||||
"cell_type": "code",
|
||||
"execution_count": 5,
|
||||
"execution_count": 6,
|
||||
"id": "e197d1d7-a070-4c96-9f8a-a0e86d046e0b",
|
||||
"metadata": {},
|
||||
"outputs": [
|
||||
{
|
||||
"data": {
|
||||
"text/plain": [
|
||||
"AIMessage(content='저는 네이버 AI를 사용하는 것이 좋아요.', additional_kwargs={}, response_metadata={'stop_reason': 'stop_before', 'input_length': 25, 'output_length': 14, 'seed': 2575184681, 'ai_filter': None}, id='run-7014b330-eba3-4701-bb62-df73ce39b854-0', usage_metadata={'input_tokens': 25, 'output_tokens': 14, 'total_tokens': 39})"
|
||||
"AIMessage(content='저는 네이버 인공지능을 사용하는 것을 좋아합니다.', additional_kwargs={'refusal': None}, response_metadata={'token_usage': {'completion_tokens': 10, 'prompt_tokens': 28, 'total_tokens': 38, 'completion_tokens_details': None, 'prompt_tokens_details': None}, 'model_name': 'HCX-005', 'system_fingerprint': None, 'id': 'b7a826d17fcf4fee8386fca2ebc63284', 'finish_reason': 'stop', 'logprobs': None}, id='run-35957816-3325-4d9c-9441-e40704912be6-0', usage_metadata={'input_tokens': 28, 'output_tokens': 10, 'total_tokens': 38, 'input_token_details': {}, 'output_token_details': {}})"
|
||||
]
|
||||
},
|
||||
"execution_count": 5,
|
||||
"execution_count": 6,
|
||||
"metadata": {},
|
||||
"output_type": "execute_result"
|
||||
}
|
||||
@ -266,7 +258,7 @@
|
||||
},
|
||||
{
|
||||
"cell_type": "code",
|
||||
"execution_count": 6,
|
||||
"execution_count": 7,
|
||||
"id": "2c07af21-dda5-4514-b4de-1f214c2cebcd",
|
||||
"metadata": {},
|
||||
"outputs": [
|
||||
@ -274,7 +266,7 @@
|
||||
"name": "stdout",
|
||||
"output_type": "stream",
|
||||
"text": [
|
||||
"Certainly! In Korean, \"Hi\" is pronounced as \"안녕\" (annyeong). The first syllable, \"안,\" sounds like the \"ahh\" sound in \"apple,\" while the second syllable, \"녕,\" sounds like the \"yuh\" sound in \"you.\" So when you put them together, it's like saying \"ahhyuh-nyuhng.\" Remember to pronounce each syllable clearly and separately for accurate pronunciation."
|
||||
"In Korean, the informal way of saying 'hi' is \"안녕\" (annyeong). If you're addressing someone older or showing more respect, you would use \"안녕하세요\" (annjeonghaseyo). Both phrases are used as greetings similar to 'hello'. Remember, pronunciation is key so make sure to pronounce each syllable clearly: 안-녀-엉 (an-nyeo-eong) and 안-녕-하-세-요 (an-nyeong-ha-se-yo)."
|
||||
]
|
||||
}
|
||||
],
|
||||
@ -298,115 +290,37 @@
|
||||
"\n",
|
||||
"### Using fine-tuned models\n",
|
||||
"\n",
|
||||
"You can call fine-tuned models by passing in your corresponding `task_id` parameter. (You don’t need to specify the `model_name` parameter when calling fine-tuned model.)\n",
|
||||
"You can call fine-tuned models by passing the `task_id` to the `model` parameter as: `ft:{task_id}`.\n",
|
||||
"\n",
|
||||
"You can check `task_id` from corresponding Test App or Service App details."
|
||||
]
|
||||
},
|
||||
{
|
||||
"cell_type": "code",
|
||||
"execution_count": 7,
|
||||
"execution_count": null,
|
||||
"id": "cb436788",
|
||||
"metadata": {},
|
||||
"outputs": [
|
||||
{
|
||||
"data": {
|
||||
"text/plain": [
|
||||
"AIMessage(content='저는 네이버 AI를 사용하는 것이 너무 좋아요.', additional_kwargs={}, response_metadata={'stop_reason': 'stop_before', 'input_length': 25, 'output_length': 15, 'seed': 52559061, 'ai_filter': None}, id='run-5bea8d4a-48f3-4c34-ae70-66e60dca5344-0', usage_metadata={'input_tokens': 25, 'output_tokens': 15, 'total_tokens': 40})"
|
||||
"AIMessage(content='네이버 인공지능을 사용하는 것을 정말 좋아합니다.', additional_kwargs={'refusal': None}, response_metadata={'token_usage': {'completion_tokens': 11, 'prompt_tokens': 28, 'total_tokens': 39, 'completion_tokens_details': None, 'prompt_tokens_details': None}, 'model_name': 'HCX-005', 'system_fingerprint': None, 'id': '2222d6d411a948c883aac1e03ca6cebe', 'finish_reason': 'stop', 'logprobs': None}, id='run-9696d7e2-7afa-4bb4-9c03-b95fcf678ab8-0', usage_metadata={'input_tokens': 28, 'output_tokens': 11, 'total_tokens': 39, 'input_token_details': {}, 'output_token_details': {}})"
|
||||
]
|
||||
},
|
||||
"execution_count": 7,
|
||||
"execution_count": 10,
|
||||
"metadata": {},
|
||||
"output_type": "execute_result"
|
||||
}
|
||||
],
|
||||
"source": [
|
||||
"fine_tuned_model = ChatClovaX(\n",
|
||||
" task_id=\"5s8egt3a\", # set if you want to use fine-tuned model\n",
|
||||
" model=\"ft:a1b2c3d4\", # set as `ft:{task_id}` with your fine-tuned model's task id\n",
|
||||
" # other params...\n",
|
||||
")\n",
|
||||
"\n",
|
||||
"fine_tuned_model.invoke(messages)"
|
||||
]
|
||||
},
|
||||
{
|
||||
"cell_type": "markdown",
|
||||
"id": "f428deaf",
|
||||
"metadata": {},
|
||||
"source": [
|
||||
"### Service App\n",
|
||||
"\n",
|
||||
"When going live with production-level application using CLOVA Studio, you should apply for and use Service App. (See [here](https://guide.ncloud-docs.com/docs/en/clovastudio-playground01#서비스앱신청).)\n",
|
||||
"\n",
|
||||
"For a Service App, you should use a corresponding Service API key and can only be called with it."
|
||||
]
|
||||
},
|
||||
{
|
||||
"cell_type": "code",
|
||||
"execution_count": null,
|
||||
"id": "dcf566df",
|
||||
"metadata": {},
|
||||
"outputs": [],
|
||||
"source": [
|
||||
"# Update environment variables\n",
|
||||
"\n",
|
||||
"os.environ[\"NCP_CLOVASTUDIO_API_KEY\"] = getpass.getpass(\n",
|
||||
" \"Enter NCP CLOVA Studio Service API Key: \"\n",
|
||||
")"
|
||||
]
|
||||
},
|
||||
{
|
||||
"cell_type": "code",
|
||||
"execution_count": 9,
|
||||
"id": "cebe27ae",
|
||||
"metadata": {},
|
||||
"outputs": [],
|
||||
"source": [
|
||||
"chat = ChatClovaX(\n",
|
||||
" service_app=True, # True if you want to use your service app, default value is False.\n",
|
||||
" # clovastudio_api_key=\"...\" # if you prefer to pass api key in directly instead of using env vars\n",
|
||||
" model=\"HCX-003\",\n",
|
||||
" # other params...\n",
|
||||
")\n",
|
||||
"ai_msg = chat.invoke(messages)"
|
||||
]
|
||||
},
|
||||
{
|
||||
"cell_type": "markdown",
|
||||
"id": "d73e7140",
|
||||
"metadata": {},
|
||||
"source": [
|
||||
"### AI Filter\n",
|
||||
"\n",
|
||||
"AI Filter detects inappropriate output such as profanity from the test app (or service app included) created in Playground and informs the user. See [here](https://guide.ncloud-docs.com/docs/en/clovastudio-playground01#AIFilter) for details."
|
||||
]
|
||||
},
|
||||
{
|
||||
"cell_type": "code",
|
||||
"execution_count": null,
|
||||
"id": "32bfbc93",
|
||||
"metadata": {},
|
||||
"outputs": [],
|
||||
"source": [
|
||||
"chat = ChatClovaX(\n",
|
||||
" model=\"HCX-003\",\n",
|
||||
" include_ai_filters=True, # True if you want to enable ai filter\n",
|
||||
" # other params...\n",
|
||||
")\n",
|
||||
"\n",
|
||||
"ai_msg = chat.invoke(messages)"
|
||||
]
|
||||
},
|
||||
{
|
||||
"cell_type": "code",
|
||||
"execution_count": null,
|
||||
"id": "7bd9e179",
|
||||
"metadata": {},
|
||||
"outputs": [],
|
||||
"source": [
|
||||
"print(ai_msg.response_metadata[\"ai_filter\"])"
|
||||
]
|
||||
},
|
||||
{
|
||||
"cell_type": "markdown",
|
||||
"id": "3a5bb5ca-c3ae-4a58-be67-2cd18574b9a3",
|
||||
@ -414,13 +328,13 @@
|
||||
"source": [
|
||||
"## API reference\n",
|
||||
"\n",
|
||||
"For detailed documentation of all ChatNaver features and configurations head to the API reference: https://python.langchain.com/api_reference/community/chat_models/langchain_community.chat_models.naver.ChatClovaX.html"
|
||||
"For detailed documentation of all ChatClovaX features and configurations head to the [API reference](https://guide.ncloud-docs.com/docs/clovastudio-dev-langchain)"
|
||||
]
|
||||
}
|
||||
],
|
||||
"metadata": {
|
||||
"kernelspec": {
|
||||
"display_name": "Python 3 (ipykernel)",
|
||||
"display_name": "Python 3",
|
||||
"language": "python",
|
||||
"name": "python3"
|
||||
},
|
||||
@ -434,7 +348,7 @@
|
||||
"name": "python",
|
||||
"nbconvert_exporter": "python",
|
||||
"pygments_lexer": "ipython3",
|
||||
"version": "3.12.3"
|
||||
"version": "3.12.8"
|
||||
}
|
||||
},
|
||||
"nbformat": 4,
|
||||
|
File diff suppressed because one or more lines are too long
@ -408,7 +408,7 @@
|
||||
"\n",
|
||||
":::\n",
|
||||
"\n",
|
||||
"OpenAI supports a [Responses](https://platform.openai.com/docs/guides/responses-vs-chat-completions) API that is oriented toward building [agentic](/docs/concepts/agents/) applications. It includes a suite of [built-in tools](https://platform.openai.com/docs/guides/tools?api-mode=responses), including web and file search. It also supports management of [conversation state](https://platform.openai.com/docs/guides/conversation-state?api-mode=responses), allowing you to continue a conversational thread without explicitly passing in previous messages.\n",
|
||||
"OpenAI supports a [Responses](https://platform.openai.com/docs/guides/responses-vs-chat-completions) API that is oriented toward building [agentic](/docs/concepts/agents/) applications. It includes a suite of [built-in tools](https://platform.openai.com/docs/guides/tools?api-mode=responses), including web and file search. It also supports management of [conversation state](https://platform.openai.com/docs/guides/conversation-state?api-mode=responses), allowing you to continue a conversational thread without explicitly passing in previous messages, as well as the output from [reasoning processes](https://platform.openai.com/docs/guides/reasoning?api-mode=responses).\n",
|
||||
"\n",
|
||||
"`ChatOpenAI` will route to the Responses API if one of these features is used. You can also specify `use_responses_api=True` when instantiating `ChatOpenAI`.\n",
|
||||
"\n",
|
||||
@ -1056,6 +1056,77 @@
|
||||
"print(second_response.text())"
|
||||
]
|
||||
},
|
||||
{
|
||||
"cell_type": "markdown",
|
||||
"id": "67bf5bd2-0935-40a0-b1cd-c6662b681d4b",
|
||||
"metadata": {},
|
||||
"source": [
|
||||
"### Reasoning output\n",
|
||||
"\n",
|
||||
"Some OpenAI models will generate separate text content illustrating their reasoning process. See OpenAI's [reasoning documentation](https://platform.openai.com/docs/guides/reasoning?api-mode=responses) for details.\n",
|
||||
"\n",
|
||||
"OpenAI can return a summary of the model's reasoning (although it doesn't expose the raw reasoning tokens). To configure `ChatOpenAI` to return this summary, specify the `reasoning` parameter:"
|
||||
]
|
||||
},
|
||||
{
|
||||
"cell_type": "code",
|
||||
"execution_count": 2,
|
||||
"id": "8d322f3a-0732-45ab-ac95-dfd4596e0d85",
|
||||
"metadata": {},
|
||||
"outputs": [
|
||||
{
|
||||
"data": {
|
||||
"text/plain": [
|
||||
"'3^3 = 3 × 3 × 3 = 27.'"
|
||||
]
|
||||
},
|
||||
"execution_count": 2,
|
||||
"metadata": {},
|
||||
"output_type": "execute_result"
|
||||
}
|
||||
],
|
||||
"source": [
|
||||
"from langchain_openai import ChatOpenAI\n",
|
||||
"\n",
|
||||
"reasoning = {\n",
|
||||
" \"effort\": \"medium\", # 'low', 'medium', or 'high'\n",
|
||||
" \"summary\": \"auto\", # 'detailed', 'auto', or None\n",
|
||||
"}\n",
|
||||
"\n",
|
||||
"llm = ChatOpenAI(\n",
|
||||
" model=\"o4-mini\",\n",
|
||||
" use_responses_api=True,\n",
|
||||
" model_kwargs={\"reasoning\": reasoning},\n",
|
||||
")\n",
|
||||
"response = llm.invoke(\"What is 3^3?\")\n",
|
||||
"\n",
|
||||
"# Output\n",
|
||||
"response.text()"
|
||||
]
|
||||
},
|
||||
{
|
||||
"cell_type": "code",
|
||||
"execution_count": 3,
|
||||
"id": "d7dcc082-b7c8-41b7-a5e2-441b9679e41b",
|
||||
"metadata": {},
|
||||
"outputs": [
|
||||
{
|
||||
"name": "stdout",
|
||||
"output_type": "stream",
|
||||
"text": [
|
||||
"**Calculating power of three**\n",
|
||||
"\n",
|
||||
"The user is asking for the result of 3 to the power of 3, which I know is 27. It's a straightforward question, so I’ll keep my answer concise: 27. I could explain that this is the same as multiplying 3 by itself twice: 3 × 3 × 3 equals 27. However, since the user likely just needs the answer, I’ll simply respond with 27.\n"
|
||||
]
|
||||
}
|
||||
],
|
||||
"source": [
|
||||
"# Reasoning\n",
|
||||
"reasoning = response.additional_kwargs[\"reasoning\"]\n",
|
||||
"for block in reasoning[\"summary\"]:\n",
|
||||
" print(block[\"text\"])"
|
||||
]
|
||||
},
|
||||
{
|
||||
"cell_type": "markdown",
|
||||
"id": "57e27714",
|
||||
@ -1342,6 +1413,23 @@
|
||||
"second_output_message = llm.invoke(history)"
|
||||
]
|
||||
},
|
||||
{
|
||||
"cell_type": "markdown",
|
||||
"id": "90c18d18-b25c-4509-a639-bd652b92f518",
|
||||
"metadata": {},
|
||||
"source": [
|
||||
"## Flex processing\n",
|
||||
"\n",
|
||||
"OpenAI offers a variety of [service tiers](https://platform.openai.com/docs/guides/flex-processing). The \"flex\" tier offers cheaper pricing for requests, with the trade-off that responses may take longer and resources might not always be available. This approach is best suited for non-critical tasks, including model testing, data enhancement, or jobs that can be run asynchronously.\n",
|
||||
"\n",
|
||||
"To use it, initialize the model with `service_tier=\"flex\"`:\n",
|
||||
"```python\n",
|
||||
"llm = ChatOpenAI(model=\"o4-mini\", service_tier=\"flex\")\n",
|
||||
"```\n",
|
||||
"\n",
|
||||
"Note that this is a beta feature that is only available for a subset of models. See OpenAI [docs](https://platform.openai.com/docs/guides/flex-processing) for more detail."
|
||||
]
|
||||
},
|
||||
{
|
||||
"cell_type": "markdown",
|
||||
"id": "a796d728-971b-408b-88d5-440015bbb941",
|
||||
@ -1349,7 +1437,7 @@
|
||||
"source": [
|
||||
"## API reference\n",
|
||||
"\n",
|
||||
"For detailed documentation of all ChatOpenAI features and configurations head to the API reference: https://python.langchain.com/api_reference/openai/chat_models/langchain_openai.chat_models.base.ChatOpenAI.html"
|
||||
"For detailed documentation of all ChatOpenAI features and configurations head to the [API reference](https://python.langchain.com/api_reference/openai/chat_models/langchain_openai.chat_models.base.ChatOpenAI.html)."
|
||||
]
|
||||
}
|
||||
],
|
||||
|
@ -17,12 +17,66 @@
|
||||
"source": [
|
||||
"# ChatPerplexity\n",
|
||||
"\n",
|
||||
"This notebook covers how to get started with `Perplexity` chat models."
|
||||
"\n",
|
||||
"This page will help you get started with Perplexity [chat models](../../concepts/chat_models.mdx). For detailed documentation of all `ChatPerplexity` features and configurations head to the [API reference](https://python.langchain.com/api_reference/perplexity/chat_models/langchain_perplexity.chat_models.ChatPerplexity.html).\n",
|
||||
"\n",
|
||||
"## Overview\n",
|
||||
"### Integration details\n",
|
||||
"\n",
|
||||
"| Class | Package | Local | Serializable | [JS support](https://js.langchain.com/docs/integrations/chat/xai) | Package downloads | Package latest |\n",
|
||||
"| :--- | :--- | :---: | :---: | :---: | :---: | :---: |\n",
|
||||
"| [ChatPerplexity](https://python.langchain.com/api_reference/perplexity/chat_models/langchain_perplexity.chat_models.ChatPerplexity.html) | [langchain-perplexity](https://python.langchain.com/api_reference/perplexity/perplexity.html) | ❌ | beta | ❌ |  |  |\n",
|
||||
"\n",
|
||||
"### Model features\n",
|
||||
"| [Tool calling](../../how_to/tool_calling.ipynb) | [Structured output](../../how_to/structured_output.ipynb) | JSON mode | [Image input](../../how_to/multimodal_inputs.ipynb) | Audio input | Video input | [Token-level streaming](../../how_to/chat_streaming.ipynb) | Native async | [Token usage](../../how_to/chat_token_usage_tracking.ipynb) | [Logprobs](../../how_to/logprobs.ipynb) |\n",
|
||||
"| :---: | :---: | :---: | :---: | :---: | :---: | :---: | :---: | :---: | :---: |\n",
|
||||
"| ❌ | ✅ | ✅ | ❌ | ❌ | ❌ | ✅ | ❌ | ✅ | ❌ |\n",
|
||||
"\n",
|
||||
"## Setup\n",
|
||||
"\n",
|
||||
"To access Perplexity models you'll need to create a Perplexity account, get an API key, and install the `langchain-perplexity` integration package.\n",
|
||||
"\n",
|
||||
"### Credentials\n",
|
||||
"\n",
|
||||
"Head to [this page](https://www.perplexity.ai/) to sign up for Perplexity and generate an API key. Once you've done this set the `PPLX_API_KEY` environment variable:"
|
||||
]
|
||||
},
|
||||
{
|
||||
"cell_type": "code",
|
||||
"execution_count": 1,
|
||||
"execution_count": null,
|
||||
"id": "2243f329",
|
||||
"metadata": {},
|
||||
"outputs": [],
|
||||
"source": [
|
||||
"import getpass\n",
|
||||
"import os\n",
|
||||
"\n",
|
||||
"if \"PPLX_API_KEY\" not in os.environ:\n",
|
||||
" os.environ[\"PPLX_API_KEY\"] = getpass.getpass(\"Enter your Perplexity API key: \")"
|
||||
]
|
||||
},
|
||||
{
|
||||
"cell_type": "markdown",
|
||||
"id": "7dfe47c4",
|
||||
"metadata": {},
|
||||
"source": [
|
||||
"To enable automated tracing of your model calls, set your [LangSmith](https://docs.smith.langchain.com/) API key:"
|
||||
]
|
||||
},
|
||||
{
|
||||
"cell_type": "code",
|
||||
"execution_count": null,
|
||||
"id": "10a791fa",
|
||||
"metadata": {},
|
||||
"outputs": [],
|
||||
"source": [
|
||||
"# os.environ[\"LANGSMITH_API_KEY\"] = getpass.getpass(\"Enter your LangSmith API key: \")\n",
|
||||
"# os.environ[\"LANGSMITH_TRACING\"] = \"true\""
|
||||
]
|
||||
},
|
||||
{
|
||||
"cell_type": "code",
|
||||
"execution_count": null,
|
||||
"id": "d4a7c55d-b235-4ca4-a579-c90cc9570da9",
|
||||
"metadata": {
|
||||
"ExecuteTime": {
|
||||
@ -33,8 +87,8 @@
|
||||
},
|
||||
"outputs": [],
|
||||
"source": [
|
||||
"from langchain_community.chat_models import ChatPerplexity\n",
|
||||
"from langchain_core.prompts import ChatPromptTemplate"
|
||||
"from langchain_core.prompts import ChatPromptTemplate\n",
|
||||
"from langchain_perplexity import ChatPerplexity"
|
||||
]
|
||||
},
|
||||
{
|
||||
@ -62,29 +116,9 @@
|
||||
"id": "97a8ce3a",
|
||||
"metadata": {},
|
||||
"source": [
|
||||
"The code provided assumes that your PPLX_API_KEY is set in your environment variables. If you would like to manually specify your API key and also choose a different model, you can use the following code:\n",
|
||||
"\n",
|
||||
"```python\n",
|
||||
"chat = ChatPerplexity(temperature=0, pplx_api_key=\"YOUR_API_KEY\", model=\"llama-3.1-sonar-small-128k-online\")\n",
|
||||
"```\n",
|
||||
"\n",
|
||||
"You can check a list of available models [here](https://docs.perplexity.ai/docs/model-cards). For reproducibility, we can set the API key dynamically by taking it as an input in this notebook."
|
||||
]
|
||||
},
|
||||
{
|
||||
"cell_type": "code",
|
||||
"execution_count": 2,
|
||||
"id": "d3e49d78",
|
||||
"metadata": {},
|
||||
"outputs": [],
|
||||
"source": [
|
||||
"import os\n",
|
||||
"from getpass import getpass\n",
|
||||
"\n",
|
||||
"PPLX_API_KEY = getpass()\n",
|
||||
"os.environ[\"PPLX_API_KEY\"] = PPLX_API_KEY"
|
||||
]
|
||||
},
|
||||
{
|
||||
"cell_type": "code",
|
||||
"execution_count": 3,
|
||||
@ -305,7 +339,7 @@
|
||||
],
|
||||
"metadata": {
|
||||
"kernelspec": {
|
||||
"display_name": "Python 3 (ipykernel)",
|
||||
"display_name": ".venv",
|
||||
"language": "python",
|
||||
"name": "python3"
|
||||
},
|
||||
@ -319,7 +353,7 @@
|
||||
"name": "python",
|
||||
"nbconvert_exporter": "python",
|
||||
"pygments_lexer": "ipython3",
|
||||
"version": "3.10.4"
|
||||
"version": "3.11.11"
|
||||
}
|
||||
},
|
||||
"nbformat": 4,
|
||||
|
@ -57,8 +57,8 @@
|
||||
{
|
||||
"metadata": {
|
||||
"ExecuteTime": {
|
||||
"end_time": "2024-11-08T19:44:51.390231Z",
|
||||
"start_time": "2024-11-08T19:44:51.387945Z"
|
||||
"end_time": "2025-04-21T18:23:30.746350Z",
|
||||
"start_time": "2025-04-21T18:23:30.744744Z"
|
||||
}
|
||||
},
|
||||
"cell_type": "code",
|
||||
@ -70,7 +70,7 @@
|
||||
],
|
||||
"id": "fa57fba89276da13",
|
||||
"outputs": [],
|
||||
"execution_count": 1
|
||||
"execution_count": 2
|
||||
},
|
||||
{
|
||||
"metadata": {},
|
||||
@ -82,12 +82,25 @@
|
||||
"id": "87dc1742af7b053"
|
||||
},
|
||||
{
|
||||
"metadata": {},
|
||||
"metadata": {
|
||||
"ExecuteTime": {
|
||||
"end_time": "2025-04-21T18:23:33.359278Z",
|
||||
"start_time": "2025-04-21T18:23:32.853207Z"
|
||||
}
|
||||
},
|
||||
"cell_type": "code",
|
||||
"source": "%pip install -qU langchain-predictionguard",
|
||||
"id": "b816ae8553cba021",
|
||||
"outputs": [],
|
||||
"execution_count": null
|
||||
"outputs": [
|
||||
{
|
||||
"name": "stdout",
|
||||
"output_type": "stream",
|
||||
"text": [
|
||||
"Note: you may need to restart the kernel to use updated packages.\n"
|
||||
]
|
||||
}
|
||||
],
|
||||
"execution_count": 3
|
||||
},
|
||||
{
|
||||
"cell_type": "markdown",
|
||||
@ -103,13 +116,13 @@
|
||||
"metadata": {
|
||||
"id": "2xe8JEUwA7_y",
|
||||
"ExecuteTime": {
|
||||
"end_time": "2024-11-08T19:44:53.950653Z",
|
||||
"start_time": "2024-11-08T19:44:53.488694Z"
|
||||
"end_time": "2025-04-21T18:23:39.812675Z",
|
||||
"start_time": "2025-04-21T18:23:39.666881Z"
|
||||
}
|
||||
},
|
||||
"source": "from langchain_predictionguard import ChatPredictionGuard",
|
||||
"outputs": [],
|
||||
"execution_count": 2
|
||||
"execution_count": 4
|
||||
},
|
||||
{
|
||||
"cell_type": "code",
|
||||
@ -117,8 +130,8 @@
|
||||
"metadata": {
|
||||
"id": "Ua7Mw1N4HcER",
|
||||
"ExecuteTime": {
|
||||
"end_time": "2024-11-08T19:44:54.890695Z",
|
||||
"start_time": "2024-11-08T19:44:54.502846Z"
|
||||
"end_time": "2025-04-21T18:23:41.590296Z",
|
||||
"start_time": "2025-04-21T18:23:41.253237Z"
|
||||
}
|
||||
},
|
||||
"source": [
|
||||
@ -126,7 +139,7 @@
|
||||
"chat = ChatPredictionGuard(model=\"Hermes-3-Llama-3.1-8B\")"
|
||||
],
|
||||
"outputs": [],
|
||||
"execution_count": 3
|
||||
"execution_count": 5
|
||||
},
|
||||
{
|
||||
"metadata": {},
|
||||
@ -221,6 +234,132 @@
|
||||
],
|
||||
"execution_count": 6
|
||||
},
|
||||
{
|
||||
"metadata": {},
|
||||
"cell_type": "markdown",
|
||||
"source": [
|
||||
"## Tool Calling\n",
|
||||
"\n",
|
||||
"Prediction Guard has a tool calling API that lets you describe tools and their arguments, which enables the model return a JSON object with a tool to call and the inputs to that tool. Tool-calling is very useful for building tool-using chains and agents, and for getting structured outputs from models more generally.\n"
|
||||
],
|
||||
"id": "1227780d6e6728ba"
|
||||
},
|
||||
{
|
||||
"metadata": {},
|
||||
"cell_type": "markdown",
|
||||
"source": [
|
||||
"### ChatPredictionGuard.bind_tools()\n",
|
||||
"\n",
|
||||
"Using `ChatPredictionGuard.bind_tools()`, you can pass in Pydantic classes, dict schemas, and Langchain tools as tools to the model, which are then reformatted to allow for use by the model."
|
||||
],
|
||||
"id": "23446aa52e01d1ba"
|
||||
},
|
||||
{
|
||||
"metadata": {},
|
||||
"cell_type": "code",
|
||||
"outputs": [],
|
||||
"execution_count": null,
|
||||
"source": [
|
||||
"from pydantic import BaseModel, Field\n",
|
||||
"\n",
|
||||
"\n",
|
||||
"class GetWeather(BaseModel):\n",
|
||||
" \"\"\"Get the current weather in a given location\"\"\"\n",
|
||||
"\n",
|
||||
" location: str = Field(..., description=\"The city and state, e.g. San Francisco, CA\")\n",
|
||||
"\n",
|
||||
"\n",
|
||||
"class GetPopulation(BaseModel):\n",
|
||||
" \"\"\"Get the current population in a given location\"\"\"\n",
|
||||
"\n",
|
||||
" location: str = Field(..., description=\"The city and state, e.g. San Francisco, CA\")\n",
|
||||
"\n",
|
||||
"\n",
|
||||
"llm_with_tools = chat.bind_tools(\n",
|
||||
" [GetWeather, GetPopulation]\n",
|
||||
" # strict = True # enforce tool args schema is respected\n",
|
||||
")"
|
||||
],
|
||||
"id": "135efb0bfc5916c1"
|
||||
},
|
||||
{
|
||||
"metadata": {
|
||||
"ExecuteTime": {
|
||||
"end_time": "2025-04-21T18:42:41.834079Z",
|
||||
"start_time": "2025-04-21T18:42:40.289095Z"
|
||||
}
|
||||
},
|
||||
"cell_type": "code",
|
||||
"source": [
|
||||
"ai_msg = llm_with_tools.invoke(\n",
|
||||
" \"Which city is hotter today and which is bigger: LA or NY?\"\n",
|
||||
")\n",
|
||||
"ai_msg"
|
||||
],
|
||||
"id": "8136f19a8836cd58",
|
||||
"outputs": [
|
||||
{
|
||||
"data": {
|
||||
"text/plain": [
|
||||
"AIMessage(content='', additional_kwargs={'tool_calls': [{'id': 'chatcmpl-tool-b1204a3c70b44cd8802579df48df0c8c', 'type': 'function', 'index': 0, 'function': {'name': 'GetWeather', 'arguments': '{\"location\": \"Los Angeles, CA\"}'}}, {'id': 'chatcmpl-tool-e299116c05bf4ce498cd6042928ae080', 'type': 'function', 'index': 0, 'function': {'name': 'GetWeather', 'arguments': '{\"location\": \"New York, NY\"}'}}, {'id': 'chatcmpl-tool-19502a60f30348669ffbac00ff503388', 'type': 'function', 'index': 0, 'function': {'name': 'GetPopulation', 'arguments': '{\"location\": \"Los Angeles, CA\"}'}}, {'id': 'chatcmpl-tool-4b8d56ef067f447795d9146a56e43510', 'type': 'function', 'index': 0, 'function': {'name': 'GetPopulation', 'arguments': '{\"location\": \"New York, NY\"}'}}]}, response_metadata={}, id='run-4630cfa9-4e95-42dd-8e4a-45db78180a10-0', tool_calls=[{'name': 'GetWeather', 'args': {'location': 'Los Angeles, CA'}, 'id': 'chatcmpl-tool-b1204a3c70b44cd8802579df48df0c8c', 'type': 'tool_call'}, {'name': 'GetWeather', 'args': {'location': 'New York, NY'}, 'id': 'chatcmpl-tool-e299116c05bf4ce498cd6042928ae080', 'type': 'tool_call'}, {'name': 'GetPopulation', 'args': {'location': 'Los Angeles, CA'}, 'id': 'chatcmpl-tool-19502a60f30348669ffbac00ff503388', 'type': 'tool_call'}, {'name': 'GetPopulation', 'args': {'location': 'New York, NY'}, 'id': 'chatcmpl-tool-4b8d56ef067f447795d9146a56e43510', 'type': 'tool_call'}])"
|
||||
]
|
||||
},
|
||||
"execution_count": 7,
|
||||
"metadata": {},
|
||||
"output_type": "execute_result"
|
||||
}
|
||||
],
|
||||
"execution_count": 7
|
||||
},
|
||||
{
|
||||
"metadata": {},
|
||||
"cell_type": "markdown",
|
||||
"source": [
|
||||
"### AIMessage.tool_calls\n",
|
||||
"\n",
|
||||
"Notice that the AIMessage has a tool_calls attribute. This contains in a standardized ToolCall format that is model-provider agnostic."
|
||||
],
|
||||
"id": "84f405c45a35abe5"
|
||||
},
|
||||
{
|
||||
"metadata": {
|
||||
"ExecuteTime": {
|
||||
"end_time": "2025-04-21T18:43:00.429453Z",
|
||||
"start_time": "2025-04-21T18:43:00.426399Z"
|
||||
}
|
||||
},
|
||||
"cell_type": "code",
|
||||
"source": "ai_msg.tool_calls",
|
||||
"id": "bdcee85475019719",
|
||||
"outputs": [
|
||||
{
|
||||
"data": {
|
||||
"text/plain": [
|
||||
"[{'name': 'GetWeather',\n",
|
||||
" 'args': {'location': 'Los Angeles, CA'},\n",
|
||||
" 'id': 'chatcmpl-tool-b1204a3c70b44cd8802579df48df0c8c',\n",
|
||||
" 'type': 'tool_call'},\n",
|
||||
" {'name': 'GetWeather',\n",
|
||||
" 'args': {'location': 'New York, NY'},\n",
|
||||
" 'id': 'chatcmpl-tool-e299116c05bf4ce498cd6042928ae080',\n",
|
||||
" 'type': 'tool_call'},\n",
|
||||
" {'name': 'GetPopulation',\n",
|
||||
" 'args': {'location': 'Los Angeles, CA'},\n",
|
||||
" 'id': 'chatcmpl-tool-19502a60f30348669ffbac00ff503388',\n",
|
||||
" 'type': 'tool_call'},\n",
|
||||
" {'name': 'GetPopulation',\n",
|
||||
" 'args': {'location': 'New York, NY'},\n",
|
||||
" 'id': 'chatcmpl-tool-4b8d56ef067f447795d9146a56e43510',\n",
|
||||
" 'type': 'tool_call'}]"
|
||||
]
|
||||
},
|
||||
"execution_count": 8,
|
||||
"metadata": {},
|
||||
"output_type": "execute_result"
|
||||
}
|
||||
],
|
||||
"execution_count": 8
|
||||
},
|
||||
{
|
||||
"cell_type": "markdown",
|
||||
"id": "ff1b51a8",
|
||||
|
284
docs/docs/integrations/chat/qwq.ipynb
Normal file
284
docs/docs/integrations/chat/qwq.ipynb
Normal file
@ -0,0 +1,284 @@
|
||||
{
|
||||
"cells": [
|
||||
{
|
||||
"cell_type": "raw",
|
||||
"id": "afaf8039",
|
||||
"metadata": {
|
||||
"vscode": {
|
||||
"languageId": "raw"
|
||||
}
|
||||
},
|
||||
"source": [
|
||||
"---\n",
|
||||
"sidebar_label: Qwen QwQ\n",
|
||||
"---"
|
||||
]
|
||||
},
|
||||
{
|
||||
"cell_type": "markdown",
|
||||
"id": "e49f1e0d",
|
||||
"metadata": {},
|
||||
"source": [
|
||||
"# ChatQwQ\n",
|
||||
"\n",
|
||||
"This will help you getting started with QwQ [chat models](../../concepts/chat_models.mdx). For detailed documentation of all ChatQwQ features and configurations head to the [API reference](https://pypi.org/project/langchain-qwq/).\n",
|
||||
"\n",
|
||||
"## Overview\n",
|
||||
"### Integration details\n",
|
||||
"\n",
|
||||
"\n",
|
||||
"| Class | Package | Local | Serializable | Package downloads | Package latest |\n",
|
||||
"| :--- | :--- | :---: | :---: | :---: | :---: |\n",
|
||||
"| [ChatQwQ](https://pypi.org/project/langchain-qwq/) | [langchain-qwq](https://pypi.org/project/langchain-qwq/) | ❌ | beta |  |  |\n",
|
||||
"\n",
|
||||
"### Model features\n",
|
||||
"| [Tool calling](../../how_to/tool_calling.ipynb) | [Structured output](../../how_to/structured_output.ipynb) | JSON mode | [Image input](../../how_to/multimodal_inputs.ipynb) | Audio input | Video input | [Token-level streaming](../../how_to/chat_streaming.ipynb) | Native async | [Token usage](../../how_to/chat_token_usage_tracking.ipynb) | [Logprobs](../../how_to/logprobs.ipynb) |\n",
|
||||
"| :---: | :---: | :---: | :---: | :---: | :---: | :---: | :---: | :---: | :---: |\n",
|
||||
"| ✅ | ✅ | ✅ |❌ | ❌ | ❌ | ✅ | ✅ | ✅ | ❌ | \n",
|
||||
"\n",
|
||||
"## Setup\n",
|
||||
"\n",
|
||||
"To access QwQ models you'll need to create an Alibaba Cloud account, get an API key, and install the `langchain-qwq` integration package.\n",
|
||||
"\n",
|
||||
"### Credentials\n",
|
||||
"\n",
|
||||
"Head to [Alibaba's API Key page](https://account.alibabacloud.com/login/login.htm?oauth_callback=https%3A%2F%2Fbailian.console.alibabacloud.com%2F%3FapiKey%3D1&lang=en#/api-key) to sign up to Alibaba Cloud and generate an API key. Once you've done this set the `DASHSCOPE_API_KEY` environment variable:"
|
||||
]
|
||||
},
|
||||
{
|
||||
"cell_type": "code",
|
||||
"execution_count": 2,
|
||||
"id": "433e8d2b-9519-4b49-b2c4-7ab65b046c94",
|
||||
"metadata": {},
|
||||
"outputs": [],
|
||||
"source": [
|
||||
"import getpass\n",
|
||||
"import os\n",
|
||||
"\n",
|
||||
"if not os.getenv(\"DASHSCOPE_API_KEY\"):\n",
|
||||
" os.environ[\"DASHSCOPE_API_KEY\"] = getpass.getpass(\"Enter your Dashscope API key: \")"
|
||||
]
|
||||
},
|
||||
{
|
||||
"cell_type": "markdown",
|
||||
"id": "0730d6a1-c893-4840-9817-5e5251676d5d",
|
||||
"metadata": {},
|
||||
"source": [
|
||||
"### Installation\n",
|
||||
"\n",
|
||||
"The LangChain QwQ integration lives in the `langchain-qwq` package:"
|
||||
]
|
||||
},
|
||||
{
|
||||
"cell_type": "code",
|
||||
"execution_count": null,
|
||||
"id": "652d6238-1f87-422a-b135-f5abbb8652fc",
|
||||
"metadata": {},
|
||||
"outputs": [],
|
||||
"source": [
|
||||
"%pip install -qU langchain-qwq"
|
||||
]
|
||||
},
|
||||
{
|
||||
"cell_type": "markdown",
|
||||
"id": "a38cde65-254d-4219-a441-068766c0d4b5",
|
||||
"metadata": {},
|
||||
"source": [
|
||||
"## Instantiation\n",
|
||||
"\n",
|
||||
"Now we can instantiate our model object and generate chat completions:"
|
||||
]
|
||||
},
|
||||
{
|
||||
"cell_type": "code",
|
||||
"execution_count": 3,
|
||||
"id": "cb09c344-1836-4e0c-acf8-11d13ac1dbae",
|
||||
"metadata": {},
|
||||
"outputs": [],
|
||||
"source": [
|
||||
"from langchain_qwq import ChatQwQ\n",
|
||||
"\n",
|
||||
"llm = ChatQwQ(\n",
|
||||
" model=\"qwq-plus\",\n",
|
||||
" max_tokens=3_000,\n",
|
||||
" timeout=None,\n",
|
||||
" max_retries=2,\n",
|
||||
" # other params...\n",
|
||||
")"
|
||||
]
|
||||
},
|
||||
{
|
||||
"cell_type": "markdown",
|
||||
"id": "2b4f3e15",
|
||||
"metadata": {},
|
||||
"source": [
|
||||
"## Invocation"
|
||||
]
|
||||
},
|
||||
{
|
||||
"cell_type": "code",
|
||||
"execution_count": 4,
|
||||
"id": "62e0dbc3",
|
||||
"metadata": {
|
||||
"tags": []
|
||||
},
|
||||
"outputs": [
|
||||
{
|
||||
"data": {
|
||||
"text/plain": [
|
||||
"AIMessage(content=\"J'aime la programmation.\", additional_kwargs={'reasoning_content': 'Okay, the user wants me to translate \"I love programming.\" into French. Let\\'s start by breaking down the sentence. The subject is \"I\", which in French is \"Je\". The verb is \"love\", which in this context is present tense, so \"aime\". The object is \"programming\". Now, \"programming\" in French can be \"la programmation\". \\n\\nWait, should it be \"programmation\" or \"programmation\"? Let me confirm the spelling. Yes, \"programmation\" is correct. Now, putting it all together: \"Je aime la programmation.\" Hmm, but in French, there\\'s a tendency to contract \"je\" and \"aime\". Wait, actually, \"je\" followed by a vowel sound usually takes \"j\\'\". So it should be \"J\\'aime la programmation.\" \\n\\nLet me double-check. \"J\\'aime\" is the correct contraction for \"I love\". The definite article \"la\" is needed because \"programmation\" is a feminine noun. Yes, \"programmation\" is a feminine noun, so \"la\" is correct. \\n\\nIs there any other way to say it? Maybe \"J\\'adore la programmation\" for \"I love\" in a stronger sense, but the user didn\\'t specify the intensity. Since the original is straightforward, \"J\\'aime la programmation.\" is the direct translation. \\n\\nI think that\\'s it. No mistakes there. So the final translation should be \"J\\'aime la programmation.\"'}, response_metadata={'model_name': 'qwq-plus'}, id='run-5045cd6a-edbd-4b2f-bf24-b7bdf3777fb9-0', usage_metadata={'input_tokens': 32, 'output_tokens': 326, 'total_tokens': 358, 'input_token_details': {}, 'output_token_details': {}})"
|
||||
]
|
||||
},
|
||||
"execution_count": 4,
|
||||
"metadata": {},
|
||||
"output_type": "execute_result"
|
||||
}
|
||||
],
|
||||
"source": [
|
||||
"messages = [\n",
|
||||
" (\n",
|
||||
" \"system\",\n",
|
||||
" \"You are a helpful assistant that translates English to French.\"\n",
|
||||
" \"Translate the user sentence.\",\n",
|
||||
" ),\n",
|
||||
" (\"human\", \"I love programming.\"),\n",
|
||||
"]\n",
|
||||
"ai_msg = llm.invoke(messages)\n",
|
||||
"ai_msg"
|
||||
]
|
||||
},
|
||||
{
|
||||
"cell_type": "markdown",
|
||||
"id": "18e2bfc0-7e78-4528-a73f-499ac150dca8",
|
||||
"metadata": {},
|
||||
"source": [
|
||||
"## Chaining\n",
|
||||
"\n",
|
||||
"We can [chain](../../how_to/sequence.ipynb) our model with a prompt template like so:"
|
||||
]
|
||||
},
|
||||
{
|
||||
"cell_type": "code",
|
||||
"execution_count": 5,
|
||||
"id": "e197d1d7-a070-4c96-9f8a-a0e86d046e0b",
|
||||
"metadata": {},
|
||||
"outputs": [
|
||||
{
|
||||
"data": {
|
||||
"text/plain": [
|
||||
"AIMessage(content='Ich liebe das Programmieren.', additional_kwargs={'reasoning_content': 'Okay, the user wants me to translate \"I love programming.\" into German. Let me think. The verb \"love\" is \"lieben\" or \"mögen\" in German, but \"lieben\" is more like love, while \"mögen\" is prefer. Since it\\'s about programming, which is a strong affection, \"lieben\" is better. The subject is \"I\", which is \"ich\". Then \"programming\" is \"Programmierung\" or \"Coding\". But \"Programmierung\" is more formal. Alternatively, sometimes people say \"ich liebe es zu programmieren\" which is \"I love to program\". Hmm, maybe the direct translation would be \"Ich liebe die Programmierung.\" But maybe the more natural way is \"Ich liebe es zu programmieren.\" Let me check. Both are correct, but the second one might sound more natural in everyday speech. The user might prefer the concise version. Alternatively, maybe \"Ich liebe die Programmierung.\" is better. Wait, the original is \"programming\" as a noun. So using the noun form would be appropriate. So \"Ich liebe die Programmierung.\" But sometimes people also use \"Coding\" in German, like \"Ich liebe das Coding.\" But that\\'s more anglicism. Probably better to stick with \"Programmierung\". Alternatively, \"Programmieren\" as a noun. Oh right! \"Programmieren\" can be a noun when used in the accusative case. So \"Ich liebe das Programmieren.\" That\\'s correct and natural. Yes, that\\'s the best translation. So the answer is \"Ich liebe das Programmieren.\"'}, response_metadata={'model_name': 'qwq-plus'}, id='run-2c418451-51d8-4319-8269-2ce129363a1a-0', usage_metadata={'input_tokens': 28, 'output_tokens': 341, 'total_tokens': 369, 'input_token_details': {}, 'output_token_details': {}})"
|
||||
]
|
||||
},
|
||||
"execution_count": 5,
|
||||
"metadata": {},
|
||||
"output_type": "execute_result"
|
||||
}
|
||||
],
|
||||
"source": [
|
||||
"from langchain_core.prompts import ChatPromptTemplate\n",
|
||||
"\n",
|
||||
"prompt = ChatPromptTemplate(\n",
|
||||
" [\n",
|
||||
" (\n",
|
||||
" \"system\",\n",
|
||||
" \"You are a helpful assistant that translates\"\n",
|
||||
" \"{input_language} to {output_language}.\",\n",
|
||||
" ),\n",
|
||||
" (\"human\", \"{input}\"),\n",
|
||||
" ]\n",
|
||||
")\n",
|
||||
"\n",
|
||||
"chain = prompt | llm\n",
|
||||
"chain.invoke(\n",
|
||||
" {\n",
|
||||
" \"input_language\": \"English\",\n",
|
||||
" \"output_language\": \"German\",\n",
|
||||
" \"input\": \"I love programming.\",\n",
|
||||
" }\n",
|
||||
")"
|
||||
]
|
||||
},
|
||||
{
|
||||
"cell_type": "markdown",
|
||||
"id": "8d1b3ef3",
|
||||
"metadata": {},
|
||||
"source": [
|
||||
"## Tool Calling\n",
|
||||
"ChatQwQ supports tool calling API that lets you describe tools and their arguments, and have the model return a JSON object with a tool to invoke and the inputs to that tool."
|
||||
]
|
||||
},
|
||||
{
|
||||
"cell_type": "markdown",
|
||||
"id": "6db1a355",
|
||||
"metadata": {},
|
||||
"source": [
|
||||
"### Use with `bind_tools`"
|
||||
]
|
||||
},
|
||||
{
|
||||
"cell_type": "code",
|
||||
"execution_count": 6,
|
||||
"id": "15fb6a6d",
|
||||
"metadata": {},
|
||||
"outputs": [
|
||||
{
|
||||
"name": "stdout",
|
||||
"output_type": "stream",
|
||||
"text": [
|
||||
"content='' additional_kwargs={'reasoning_content': 'Okay, the user is asking \"What\\'s 5 times forty two\". Let me break this down. First, I need to identify the numbers involved. The first number is 5, which is straightforward. The second number is forty two, which is 42 in digits. The operation they want is multiplication.\\n\\nLooking at the tools provided, there\\'s a function called multiply that takes two integers. So I should use that. The parameters are first_int and second_int. \\n\\nI need to convert \"forty two\" to 42. Since the function requires integers, both numbers should be in integer form. So 5 and 42. \\n\\nNow, I\\'ll structure the tool call. The function name is multiply, and the arguments should be first_int: 5 and second_int: 42. I\\'ll make sure the JSON is correctly formatted without any syntax errors. Let me double-check the parameters to ensure they\\'re required and of the right type. Yep, both are required and integers. \\n\\nNo examples were provided, but the function\\'s purpose is clear. So the correct tool call should be to multiply those two numbers. I think that\\'s all. No other functions are needed here.'} response_metadata={'model_name': 'qwq-plus'} id='run-638895aa-fdde-4567-bcfa-7d8e5d4f24af-0' tool_calls=[{'name': 'multiply', 'args': {'first_int': 5, 'second_int': 42}, 'id': 'call_d088275851c140529ed2ad', 'type': 'tool_call'}] usage_metadata={'input_tokens': 176, 'output_tokens': 277, 'total_tokens': 453, 'input_token_details': {}, 'output_token_details': {}}\n"
|
||||
]
|
||||
}
|
||||
],
|
||||
"source": [
|
||||
"from langchain_core.tools import tool\n",
|
||||
"from langchain_qwq import ChatQwQ\n",
|
||||
"\n",
|
||||
"\n",
|
||||
"@tool\n",
|
||||
"def multiply(first_int: int, second_int: int) -> int:\n",
|
||||
" \"\"\"Multiply two integers together.\"\"\"\n",
|
||||
" return first_int * second_int\n",
|
||||
"\n",
|
||||
"\n",
|
||||
"llm = ChatQwQ()\n",
|
||||
"\n",
|
||||
"llm_with_tools = llm.bind_tools([multiply])\n",
|
||||
"\n",
|
||||
"msg = llm_with_tools.invoke(\"What's 5 times forty two\")\n",
|
||||
"\n",
|
||||
"print(msg)"
|
||||
]
|
||||
},
|
||||
{
|
||||
"cell_type": "markdown",
|
||||
"id": "3a5bb5ca-c3ae-4a58-be67-2cd18574b9a3",
|
||||
"metadata": {},
|
||||
"source": [
|
||||
"## API reference\n",
|
||||
"\n",
|
||||
"For detailed documentation of all ChatQwQ features and configurations head to the [API reference](https://pypi.org/project/langchain-qwq/)"
|
||||
]
|
||||
}
|
||||
],
|
||||
"metadata": {
|
||||
"kernelspec": {
|
||||
"display_name": "Python 3",
|
||||
"language": "python",
|
||||
"name": "python3"
|
||||
},
|
||||
"language_info": {
|
||||
"codemirror_mode": {
|
||||
"name": "ipython",
|
||||
"version": 3
|
||||
},
|
||||
"file_extension": ".py",
|
||||
"mimetype": "text/x-python",
|
||||
"name": "python",
|
||||
"nbconvert_exporter": "python",
|
||||
"pygments_lexer": "ipython3",
|
||||
"version": "3.13.1"
|
||||
}
|
||||
},
|
||||
"nbformat": 4,
|
||||
"nbformat_minor": 5
|
||||
}
|
276
docs/docs/integrations/chat/runpod.ipynb
Normal file
276
docs/docs/integrations/chat/runpod.ipynb
Normal file
@ -0,0 +1,276 @@
|
||||
{
|
||||
"cells": [
|
||||
{
|
||||
"cell_type": "markdown",
|
||||
"metadata": {},
|
||||
"source": [
|
||||
"# RunPod Chat Model"
|
||||
]
|
||||
},
|
||||
{
|
||||
"cell_type": "markdown",
|
||||
"metadata": {},
|
||||
"source": [
|
||||
"Get started with RunPod chat models.\n",
|
||||
"\n",
|
||||
"## Overview\n",
|
||||
"\n",
|
||||
"This guide covers how to use the LangChain `ChatRunPod` class to interact with chat models hosted on [RunPod Serverless](https://www.runpod.io/serverless-gpu)."
|
||||
]
|
||||
},
|
||||
{
|
||||
"cell_type": "markdown",
|
||||
"metadata": {},
|
||||
"source": [
|
||||
"## Setup\n",
|
||||
"\n",
|
||||
"1. **Install the package:**\n",
|
||||
" ```bash\n",
|
||||
" pip install -qU langchain-runpod\n",
|
||||
" ```\n",
|
||||
"2. **Deploy a Chat Model Endpoint:** Follow the setup steps in the [RunPod Provider Guide](/docs/integrations/providers/runpod#setup) to deploy a compatible chat model endpoint on RunPod Serverless and get its Endpoint ID.\n",
|
||||
"3. **Set Environment Variables:** Make sure `RUNPOD_API_KEY` and `RUNPOD_ENDPOINT_ID` (or a specific `RUNPOD_CHAT_ENDPOINT_ID`) are set."
|
||||
]
|
||||
},
|
||||
{
|
||||
"cell_type": "code",
|
||||
"execution_count": null,
|
||||
"metadata": {
|
||||
"vscode": {
|
||||
"languageId": "plaintext"
|
||||
}
|
||||
},
|
||||
"outputs": [],
|
||||
"source": [
|
||||
"import getpass\n",
|
||||
"import os\n",
|
||||
"\n",
|
||||
"# Make sure environment variables are set (or pass them directly to ChatRunPod)\n",
|
||||
"if \"RUNPOD_API_KEY\" not in os.environ:\n",
|
||||
" os.environ[\"RUNPOD_API_KEY\"] = getpass.getpass(\"Enter your RunPod API Key: \")\n",
|
||||
"\n",
|
||||
"if \"RUNPOD_ENDPOINT_ID\" not in os.environ:\n",
|
||||
" os.environ[\"RUNPOD_ENDPOINT_ID\"] = input(\n",
|
||||
" \"Enter your RunPod Endpoint ID (used if RUNPOD_CHAT_ENDPOINT_ID is not set): \"\n",
|
||||
" )\n",
|
||||
"\n",
|
||||
"# Optionally use a different endpoint ID specifically for chat models\n",
|
||||
"# if \"RUNPOD_CHAT_ENDPOINT_ID\" not in os.environ:\n",
|
||||
"# os.environ[\"RUNPOD_CHAT_ENDPOINT_ID\"] = input(\"Enter your RunPod Chat Endpoint ID (Optional): \")\n",
|
||||
"\n",
|
||||
"chat_endpoint_id = os.environ.get(\n",
|
||||
" \"RUNPOD_CHAT_ENDPOINT_ID\", os.environ.get(\"RUNPOD_ENDPOINT_ID\")\n",
|
||||
")\n",
|
||||
"if not chat_endpoint_id:\n",
|
||||
" raise ValueError(\n",
|
||||
" \"No RunPod Endpoint ID found. Please set RUNPOD_ENDPOINT_ID or RUNPOD_CHAT_ENDPOINT_ID.\"\n",
|
||||
" )"
|
||||
]
|
||||
},
|
||||
{
|
||||
"cell_type": "markdown",
|
||||
"metadata": {},
|
||||
"source": [
|
||||
"## Instantiation\n",
|
||||
"\n",
|
||||
"Initialize the `ChatRunPod` class. You can pass model-specific parameters via `model_kwargs` and configure polling behavior."
|
||||
]
|
||||
},
|
||||
{
|
||||
"cell_type": "code",
|
||||
"execution_count": null,
|
||||
"metadata": {
|
||||
"vscode": {
|
||||
"languageId": "plaintext"
|
||||
}
|
||||
},
|
||||
"outputs": [],
|
||||
"source": [
|
||||
"from langchain_runpod import ChatRunPod\n",
|
||||
"\n",
|
||||
"chat = ChatRunPod(\n",
|
||||
" runpod_endpoint_id=chat_endpoint_id, # Specify the correct endpoint ID\n",
|
||||
" model_kwargs={\n",
|
||||
" \"max_new_tokens\": 512,\n",
|
||||
" \"temperature\": 0.7,\n",
|
||||
" \"top_p\": 0.9,\n",
|
||||
" # Add other parameters supported by your endpoint handler\n",
|
||||
" },\n",
|
||||
" # Optional: Adjust polling\n",
|
||||
" # poll_interval=0.2,\n",
|
||||
" # max_polling_attempts=150\n",
|
||||
")"
|
||||
]
|
||||
},
|
||||
{
|
||||
"cell_type": "markdown",
|
||||
"metadata": {},
|
||||
"source": [
|
||||
"## Invocation\n",
|
||||
"\n",
|
||||
"Use the standard LangChain `.invoke()` and `.ainvoke()` methods to call the model. Streaming is also supported via `.stream()` and `.astream()` (simulated by polling the RunPod `/stream` endpoint)."
|
||||
]
|
||||
},
|
||||
{
|
||||
"cell_type": "code",
|
||||
"execution_count": null,
|
||||
"metadata": {
|
||||
"vscode": {
|
||||
"languageId": "plaintext"
|
||||
}
|
||||
},
|
||||
"outputs": [],
|
||||
"source": [
|
||||
"from langchain_core.messages import HumanMessage, SystemMessage\n",
|
||||
"\n",
|
||||
"messages = [\n",
|
||||
" SystemMessage(content=\"You are a helpful AI assistant.\"),\n",
|
||||
" HumanMessage(content=\"What is the RunPod Serverless API flow?\"),\n",
|
||||
"]\n",
|
||||
"\n",
|
||||
"# Invoke (Sync)\n",
|
||||
"try:\n",
|
||||
" response = chat.invoke(messages)\n",
|
||||
" print(\"--- Sync Invoke Response ---\")\n",
|
||||
" print(response.content)\n",
|
||||
"except Exception as e:\n",
|
||||
" print(\n",
|
||||
" f\"Error invoking Chat Model: {e}. Ensure endpoint ID/API key are correct and endpoint is active/compatible.\"\n",
|
||||
" )\n",
|
||||
"\n",
|
||||
"# Stream (Sync, simulated via polling /stream)\n",
|
||||
"print(\"\\n--- Sync Stream Response ---\")\n",
|
||||
"try:\n",
|
||||
" for chunk in chat.stream(messages):\n",
|
||||
" print(chunk.content, end=\"\", flush=True)\n",
|
||||
" print() # Newline\n",
|
||||
"except Exception as e:\n",
|
||||
" print(\n",
|
||||
" f\"\\nError streaming Chat Model: {e}. Ensure endpoint handler supports streaming output format.\"\n",
|
||||
" )\n",
|
||||
"\n",
|
||||
"### Async Usage\n",
|
||||
"\n",
|
||||
"# AInvoke (Async)\n",
|
||||
"try:\n",
|
||||
" async_response = await chat.ainvoke(messages)\n",
|
||||
" print(\"--- Async Invoke Response ---\")\n",
|
||||
" print(async_response.content)\n",
|
||||
"except Exception as e:\n",
|
||||
" print(f\"Error invoking Chat Model asynchronously: {e}.\")\n",
|
||||
"\n",
|
||||
"# AStream (Async)\n",
|
||||
"print(\"\\n--- Async Stream Response ---\")\n",
|
||||
"try:\n",
|
||||
" async for chunk in chat.astream(messages):\n",
|
||||
" print(chunk.content, end=\"\", flush=True)\n",
|
||||
" print() # Newline\n",
|
||||
"except Exception as e:\n",
|
||||
" print(\n",
|
||||
" f\"\\nError streaming Chat Model asynchronously: {e}. Ensure endpoint handler supports streaming output format.\\n\"\n",
|
||||
" )"
|
||||
]
|
||||
},
|
||||
{
|
||||
"cell_type": "markdown",
|
||||
"metadata": {},
|
||||
"source": [
|
||||
"## Chaining\n",
|
||||
"\n",
|
||||
"The chat model integrates seamlessly with LangChain Expression Language (LCEL) chains."
|
||||
]
|
||||
},
|
||||
{
|
||||
"cell_type": "code",
|
||||
"execution_count": null,
|
||||
"metadata": {
|
||||
"vscode": {
|
||||
"languageId": "plaintext"
|
||||
}
|
||||
},
|
||||
"outputs": [],
|
||||
"source": [
|
||||
"from langchain_core.output_parsers import StrOutputParser\n",
|
||||
"from langchain_core.prompts import ChatPromptTemplate\n",
|
||||
"\n",
|
||||
"prompt = ChatPromptTemplate.from_messages(\n",
|
||||
" [\n",
|
||||
" (\"system\", \"You are a helpful assistant.\"),\n",
|
||||
" (\"human\", \"{input}\"),\n",
|
||||
" ]\n",
|
||||
")\n",
|
||||
"\n",
|
||||
"parser = StrOutputParser()\n",
|
||||
"\n",
|
||||
"chain = prompt | chat | parser\n",
|
||||
"\n",
|
||||
"try:\n",
|
||||
" chain_response = chain.invoke(\n",
|
||||
" {\"input\": \"Explain the concept of serverless computing in simple terms.\"}\n",
|
||||
" )\n",
|
||||
" print(\"--- Chain Response ---\")\n",
|
||||
" print(chain_response)\n",
|
||||
"except Exception as e:\n",
|
||||
" print(f\"Error running chain: {e}\")\n",
|
||||
"\n",
|
||||
"\n",
|
||||
"# Async chain\n",
|
||||
"try:\n",
|
||||
" async_chain_response = await chain.ainvoke(\n",
|
||||
" {\"input\": \"What are the benefits of using RunPod for AI/ML workloads?\"}\n",
|
||||
" )\n",
|
||||
" print(\"--- Async Chain Response ---\")\n",
|
||||
" print(async_chain_response)\n",
|
||||
"except Exception as e:\n",
|
||||
" print(f\"Error running async chain: {e}\")"
|
||||
]
|
||||
},
|
||||
{
|
||||
"cell_type": "markdown",
|
||||
"metadata": {},
|
||||
"source": [
|
||||
"## Model Features (Endpoint Dependent)\n",
|
||||
"\n",
|
||||
"The availability of advanced features depends **heavily** on the specific implementation of your RunPod endpoint handler. The `ChatRunPod` integration provides the basic framework, but the handler must support the underlying functionality.\n",
|
||||
"\n",
|
||||
"| Feature | Integration Support | Endpoint Dependent? | Notes |\n",
|
||||
"| :--------------------------------------------------------- | :-----------------: | :-----------------: | :------------------------------------------------------------------------------------------------------------------------------------------------------------------------- |\n",
|
||||
"| [Tool calling](/docs/how_to/tool_calling) | ❌ | ✅ | Requires handler to process tool definitions and return tool calls (e.g., OpenAI format). Integration needs parsing logic. |\n",
|
||||
"| [Structured output](/docs/how_to/structured_output) | ❌ | ✅ | Requires handler support for forcing structured output (JSON mode, function calling). Integration needs parsing logic. |\n",
|
||||
"| JSON mode | ❌ | ✅ | Requires handler to accept a `json_mode` parameter (or similar) and guarantee JSON output. |\n",
|
||||
"| [Image input](/docs/how_to/multimodal_inputs) | ❌ | ✅ | Requires multimodal handler accepting image data (e.g., base64). Integration does not support multimodal messages. |\n",
|
||||
"| Audio input | ❌ | ✅ | Requires handler accepting audio data. Integration does not support audio messages. |\n",
|
||||
"| Video input | ❌ | ✅ | Requires handler accepting video data. Integration does not support video messages. |\n",
|
||||
"| [Token-level streaming](/docs/how_to/chat_streaming) | ✅ (Simulated) | ✅ | Polls `/stream`. Requires handler to populate `stream` list in status response with token chunks (e.g., `[{\"output\": \"token\"}]`). True low-latency streaming not built-in. |\n",
|
||||
"| Native async | ✅ | ✅ | Core `ainvoke`/`astream` implemented. Relies on endpoint handler performance. |\n",
|
||||
"| [Token usage](/docs/how_to/chat_token_usage_tracking) | ❌ | ✅ | Requires handler to return `prompt_tokens`, `completion_tokens` in the final response. Integration currently does not parse this. |\n",
|
||||
"| [Logprobs](/docs/how_to/logprobs) | ❌ | ✅ | Requires handler to return log probabilities. Integration currently does not parse this. |\n"
|
||||
]
|
||||
},
|
||||
{
|
||||
"cell_type": "markdown",
|
||||
"metadata": {},
|
||||
"source": [
|
||||
"**Key Takeaway:** Standard chat invocation and simulated streaming work if the endpoint follows basic RunPod API conventions. Advanced features require specific handler implementations and potentially extending or customizing this integration package."
|
||||
]
|
||||
},
|
||||
{
|
||||
"cell_type": "markdown",
|
||||
"metadata": {},
|
||||
"source": [
|
||||
"## API reference\n",
|
||||
"\n",
|
||||
"For detailed documentation of the `ChatRunPod` class, parameters, and methods, refer to the source code or the generated API reference (if available).\n",
|
||||
"\n",
|
||||
"Link to source code: [https://github.com/runpod/langchain-runpod/blob/main/langchain_runpod/chat_models.py](https://github.com/runpod/langchain-runpod/blob/main/langchain_runpod/chat_models.py)"
|
||||
]
|
||||
}
|
||||
],
|
||||
"metadata": {
|
||||
"language_info": {
|
||||
"name": "python"
|
||||
}
|
||||
},
|
||||
"nbformat": 4,
|
||||
"nbformat_minor": 2
|
||||
}
|
362
docs/docs/integrations/chat/seekrflow.ipynb
Normal file
362
docs/docs/integrations/chat/seekrflow.ipynb
Normal file
@ -0,0 +1,362 @@
|
||||
{
|
||||
"cells": [
|
||||
{
|
||||
"cell_type": "markdown",
|
||||
"id": "62d5a1ea",
|
||||
"metadata": {},
|
||||
"source": [
|
||||
"# ChatSeekrFlow\n",
|
||||
"\n",
|
||||
"> [Seekr](https://www.seekr.com/) provides AI-powered solutions for structured, explainable, and transparent AI interactions.\n",
|
||||
"\n",
|
||||
"This notebook provides a quick overview for getting started with Seekr [chat models](/docs/concepts/chat_models). For detailed documentation of all `ChatSeekrFlow` features and configurations, head to the [API reference](https://python.langchain.com/api_reference/community/chat_models/langchain_community.chat_models.seekrflow.ChatSeekrFlow.html).\n",
|
||||
"\n",
|
||||
"## Overview\n",
|
||||
"\n",
|
||||
"`ChatSeekrFlow` class wraps a chat model endpoint hosted on SeekrFlow, enabling seamless integration with LangChain applications.\n",
|
||||
"\n",
|
||||
"### Integration Details\n",
|
||||
"\n",
|
||||
"| Class | Package | Local | Serializable | Package downloads | Package latest |\n",
|
||||
"| :--- | :--- | :---: | :---: | :---: | :---: |\n",
|
||||
"| [ChatSeekrFlow](https://python.langchain.com/api_reference/community/chat_models/langchain_community.chat_models.seekrflow.ChatSeekrFlow.html) | [seekrai](https://python.langchain.com/docs/integrations/providers/seekr/) | ❌ | beta |  |  |\n",
|
||||
"\n",
|
||||
"### Model Features\n",
|
||||
"\n",
|
||||
"| [Tool calling](/docs/how_to/tool_calling/) | [Structured output](/docs/how_to/structured_output/) | JSON mode | [Image input](/docs/how_to/multimodal_inputs/) | Audio input | Video input | [Token-level streaming](/docs/how_to/chat_streaming/) | Native async | [Token usage](/docs/how_to/chat_token_usage_tracking/) | [Logprobs](/docs/how_to/logprobs/) |\n",
|
||||
"| :---: | :---: | :---: | :---: | :---: | :---: | :---: | :---: | :---: | :---: |\n",
|
||||
"| ✅ | ✅ | ✅ | ❌ | ❌ | ❌ | ✅ | ❌ | ✅ | ❌ |\n",
|
||||
"\n",
|
||||
"### Supported Methods\n",
|
||||
"`ChatSeekrFlow` supports all methods of `ChatModel`, **except async APIs**.\n",
|
||||
"\n",
|
||||
"### Endpoint Requirements\n",
|
||||
"\n",
|
||||
"The serving endpoint `ChatSeekrFlow` wraps **must** have OpenAI-compatible chat input/output format. It can be used for:\n",
|
||||
"1. **Fine-tuned Seekr models**\n",
|
||||
"2. **Custom SeekrFlow models**\n",
|
||||
"3. **RAG-enabled models using Seekr's retrieval system**\n",
|
||||
"\n",
|
||||
"For async usage, please refer to `AsyncChatSeekrFlow` (coming soon).\n"
|
||||
]
|
||||
},
|
||||
{
|
||||
"cell_type": "markdown",
|
||||
"id": "93fea471",
|
||||
"metadata": {},
|
||||
"source": [
|
||||
"# Getting Started with ChatSeekrFlow in LangChain\n",
|
||||
"\n",
|
||||
"This notebook covers how to use SeekrFlow as a chat model in LangChain."
|
||||
]
|
||||
},
|
||||
{
|
||||
"cell_type": "markdown",
|
||||
"id": "2f320c17",
|
||||
"metadata": {},
|
||||
"source": [
|
||||
"## Setup\n",
|
||||
"\n",
|
||||
"Ensure you have the necessary dependencies installed:\n",
|
||||
"\n",
|
||||
"```bash\n",
|
||||
"pip install seekrai langchain langchain-community\n",
|
||||
"```\n",
|
||||
"\n",
|
||||
"You must also have an API key from Seekr to authenticate requests.\n"
|
||||
]
|
||||
},
|
||||
{
|
||||
"cell_type": "code",
|
||||
"execution_count": 1,
|
||||
"id": "911ca53c",
|
||||
"metadata": {},
|
||||
"outputs": [],
|
||||
"source": [
|
||||
"# Standard library\n",
|
||||
"import getpass\n",
|
||||
"import os\n",
|
||||
"\n",
|
||||
"# Third-party\n",
|
||||
"from langchain.prompts import ChatPromptTemplate\n",
|
||||
"from langchain.schema import HumanMessage\n",
|
||||
"from langchain_core.runnables import RunnableSequence\n",
|
||||
"\n",
|
||||
"# OSS SeekrFlow integration\n",
|
||||
"from langchain_seekrflow import ChatSeekrFlow\n",
|
||||
"from seekrai import SeekrFlow"
|
||||
]
|
||||
},
|
||||
{
|
||||
"cell_type": "markdown",
|
||||
"id": "150461cb",
|
||||
"metadata": {},
|
||||
"source": [
|
||||
"## API Key Setup\n",
|
||||
"\n",
|
||||
"You'll need to set your API key as an environment variable to authenticate requests.\n",
|
||||
"\n",
|
||||
"Run the below cell.\n",
|
||||
"\n",
|
||||
"Or manually assign it before running queries:\n",
|
||||
"\n",
|
||||
"```python\n",
|
||||
"SEEKR_API_KEY = \"your-api-key-here\"\n",
|
||||
"```\n"
|
||||
]
|
||||
},
|
||||
{
|
||||
"cell_type": "code",
|
||||
"execution_count": null,
|
||||
"id": "38afcd6e",
|
||||
"metadata": {},
|
||||
"outputs": [],
|
||||
"source": [
|
||||
"os.environ[\"SEEKR_API_KEY\"] = getpass.getpass(\"Enter your Seekr API key:\")"
|
||||
]
|
||||
},
|
||||
{
|
||||
"cell_type": "markdown",
|
||||
"id": "82d83c0e",
|
||||
"metadata": {},
|
||||
"source": [
|
||||
"## Instantiation"
|
||||
]
|
||||
},
|
||||
{
|
||||
"cell_type": "code",
|
||||
"execution_count": null,
|
||||
"id": "71b14751",
|
||||
"metadata": {},
|
||||
"outputs": [],
|
||||
"source": [
|
||||
"os.environ[\"SEEKR_API_KEY\"]\n",
|
||||
"seekr_client = SeekrFlow(api_key=SEEKR_API_KEY)\n",
|
||||
"\n",
|
||||
"llm = ChatSeekrFlow(\n",
|
||||
" client=seekr_client, model_name=\"meta-llama/Meta-Llama-3-8B-Instruct\"\n",
|
||||
")"
|
||||
]
|
||||
},
|
||||
{
|
||||
"cell_type": "markdown",
|
||||
"id": "1046e86c",
|
||||
"metadata": {},
|
||||
"source": [
|
||||
"## Invocation"
|
||||
]
|
||||
},
|
||||
{
|
||||
"cell_type": "code",
|
||||
"execution_count": 4,
|
||||
"id": "f61a60f6",
|
||||
"metadata": {},
|
||||
"outputs": [
|
||||
{
|
||||
"name": "stdout",
|
||||
"output_type": "stream",
|
||||
"text": [
|
||||
"Hello there! I'm Seekr, nice to meet you! What brings you here today? Do you have a question, or are you looking for some help with something? I'm all ears (or rather, all text)!\n"
|
||||
]
|
||||
}
|
||||
],
|
||||
"source": [
|
||||
"response = llm.invoke([HumanMessage(content=\"Hello, Seekr!\")])\n",
|
||||
"print(response.content)"
|
||||
]
|
||||
},
|
||||
{
|
||||
"cell_type": "markdown",
|
||||
"id": "853b0349",
|
||||
"metadata": {},
|
||||
"source": [
|
||||
"## Chaining"
|
||||
]
|
||||
},
|
||||
{
|
||||
"cell_type": "code",
|
||||
"execution_count": 5,
|
||||
"id": "35fca3ec",
|
||||
"metadata": {},
|
||||
"outputs": [
|
||||
{
|
||||
"name": "stdout",
|
||||
"output_type": "stream",
|
||||
"text": [
|
||||
"content='The translation of \"Good morning\" in French is:\\n\\n\"Bonne journée\"' additional_kwargs={} response_metadata={}\n"
|
||||
]
|
||||
}
|
||||
],
|
||||
"source": [
|
||||
"prompt = ChatPromptTemplate.from_template(\"Translate to French: {text}\")\n",
|
||||
"\n",
|
||||
"chain: RunnableSequence = prompt | llm\n",
|
||||
"result = chain.invoke({\"text\": \"Good morning\"})\n",
|
||||
"print(result)"
|
||||
]
|
||||
},
|
||||
{
|
||||
"cell_type": "code",
|
||||
"execution_count": 6,
|
||||
"id": "a7b28b8d",
|
||||
"metadata": {},
|
||||
"outputs": [
|
||||
{
|
||||
"name": "stdout",
|
||||
"output_type": "stream",
|
||||
"text": [
|
||||
"\n",
|
||||
"🔹 Testing Sync `stream()` (Streaming)...\n",
|
||||
"Here is a haiku:\n",
|
||||
"\n",
|
||||
"Golden sunset fades\n",
|
||||
"Ripples on the quiet lake\n",
|
||||
"Peaceful evening sky"
|
||||
]
|
||||
}
|
||||
],
|
||||
"source": [
|
||||
"def test_stream():\n",
|
||||
" \"\"\"Test synchronous invocation in streaming mode.\"\"\"\n",
|
||||
" print(\"\\n🔹 Testing Sync `stream()` (Streaming)...\")\n",
|
||||
"\n",
|
||||
" for chunk in llm.stream([HumanMessage(content=\"Write me a haiku.\")]):\n",
|
||||
" print(chunk.content, end=\"\", flush=True)\n",
|
||||
"\n",
|
||||
"\n",
|
||||
"# ✅ Ensure streaming is enabled\n",
|
||||
"llm = ChatSeekrFlow(\n",
|
||||
" client=seekr_client,\n",
|
||||
" model_name=\"meta-llama/Meta-Llama-3-8B-Instruct\",\n",
|
||||
" streaming=True, # ✅ Enable streaming\n",
|
||||
")\n",
|
||||
"\n",
|
||||
"# ✅ Run sync streaming test\n",
|
||||
"test_stream()"
|
||||
]
|
||||
},
|
||||
{
|
||||
"cell_type": "markdown",
|
||||
"id": "b3847b34",
|
||||
"metadata": {},
|
||||
"source": [
|
||||
"## Error Handling & Debugging"
|
||||
]
|
||||
},
|
||||
{
|
||||
"cell_type": "code",
|
||||
"execution_count": 8,
|
||||
"id": "6bc38b48",
|
||||
"metadata": {},
|
||||
"outputs": [
|
||||
{
|
||||
"name": "stdout",
|
||||
"output_type": "stream",
|
||||
"text": [
|
||||
"Running test: Missing Client\n",
|
||||
"✅ Expected Error: SeekrFlow client cannot be None.\n",
|
||||
"Running test: Missing Model Name\n",
|
||||
"✅ Expected Error: A valid model name must be provided.\n"
|
||||
]
|
||||
}
|
||||
],
|
||||
"source": [
|
||||
"# Define a minimal mock SeekrFlow client\n",
|
||||
"class MockSeekrClient:\n",
|
||||
" \"\"\"Mock SeekrFlow API client that mimics the real API structure.\"\"\"\n",
|
||||
"\n",
|
||||
" class MockChat:\n",
|
||||
" \"\"\"Mock Chat object with a completions method.\"\"\"\n",
|
||||
"\n",
|
||||
" class MockCompletions:\n",
|
||||
" \"\"\"Mock Completions object with a create method.\"\"\"\n",
|
||||
"\n",
|
||||
" def create(self, *args, **kwargs):\n",
|
||||
" return {\n",
|
||||
" \"choices\": [{\"message\": {\"content\": \"Mock response\"}}]\n",
|
||||
" } # Mimic API response\n",
|
||||
"\n",
|
||||
" completions = MockCompletions()\n",
|
||||
"\n",
|
||||
" chat = MockChat()\n",
|
||||
"\n",
|
||||
"\n",
|
||||
"def test_initialization_errors():\n",
|
||||
" \"\"\"Test that invalid ChatSeekrFlow initializations raise expected errors.\"\"\"\n",
|
||||
"\n",
|
||||
" test_cases = [\n",
|
||||
" {\n",
|
||||
" \"name\": \"Missing Client\",\n",
|
||||
" \"args\": {\"client\": None, \"model_name\": \"seekrflow-model\"},\n",
|
||||
" \"expected_error\": \"SeekrFlow client cannot be None.\",\n",
|
||||
" },\n",
|
||||
" {\n",
|
||||
" \"name\": \"Missing Model Name\",\n",
|
||||
" \"args\": {\"client\": MockSeekrClient(), \"model_name\": \"\"},\n",
|
||||
" \"expected_error\": \"A valid model name must be provided.\",\n",
|
||||
" },\n",
|
||||
" ]\n",
|
||||
"\n",
|
||||
" for test in test_cases:\n",
|
||||
" try:\n",
|
||||
" print(f\"Running test: {test['name']}\")\n",
|
||||
" faulty_llm = ChatSeekrFlow(**test[\"args\"])\n",
|
||||
"\n",
|
||||
" # If no error is raised, fail the test\n",
|
||||
" print(f\"❌ Test '{test['name']}' failed: No error was raised!\")\n",
|
||||
" except Exception as e:\n",
|
||||
" error_msg = str(e)\n",
|
||||
" assert test[\"expected_error\"] in error_msg, f\"Unexpected error: {error_msg}\"\n",
|
||||
" print(f\"✅ Expected Error: {error_msg}\")\n",
|
||||
"\n",
|
||||
"\n",
|
||||
"# Run test\n",
|
||||
"test_initialization_errors()"
|
||||
]
|
||||
},
|
||||
{
|
||||
"cell_type": "markdown",
|
||||
"id": "d1c9ddf3",
|
||||
"metadata": {},
|
||||
"source": [
|
||||
"## API reference"
|
||||
]
|
||||
},
|
||||
{
|
||||
"cell_type": "markdown",
|
||||
"id": "411a8bea",
|
||||
"metadata": {},
|
||||
"source": [
|
||||
"- `ChatSeekrFlow` class: [`langchain_seekrflow.ChatSeekrFlow`](https://github.com/benfaircloth/langchain-seekrflow/blob/main/langchain_seekrflow/seekrflow.py)\n",
|
||||
"- PyPI package: [`langchain-seekrflow`](https://pypi.org/project/langchain-seekrflow/)\n"
|
||||
]
|
||||
},
|
||||
{
|
||||
"cell_type": "markdown",
|
||||
"id": "3ef00a51",
|
||||
"metadata": {},
|
||||
"source": []
|
||||
}
|
||||
],
|
||||
"metadata": {
|
||||
"kernelspec": {
|
||||
"display_name": ".venv",
|
||||
"language": "python",
|
||||
"name": "python3"
|
||||
},
|
||||
"language_info": {
|
||||
"codemirror_mode": {
|
||||
"name": "ipython",
|
||||
"version": 3
|
||||
},
|
||||
"file_extension": ".py",
|
||||
"mimetype": "text/x-python",
|
||||
"name": "python",
|
||||
"nbconvert_exporter": "python",
|
||||
"pygments_lexer": "ipython3",
|
||||
"version": "3.10.12"
|
||||
}
|
||||
},
|
||||
"nbformat": 4,
|
||||
"nbformat_minor": 5
|
||||
}
|
@ -34,33 +34,46 @@
|
||||
"id": "juAmbgoWD17u"
|
||||
},
|
||||
"source": [
|
||||
"The AstraDB Document Loader returns a list of Langchain Documents from an AstraDB database.\n",
|
||||
"The Astra DB Document Loader returns a list of Langchain `Document` objects read from an Astra DB collection.\n",
|
||||
"\n",
|
||||
"The Loader takes the following parameters:\n",
|
||||
"The loader takes the following parameters:\n",
|
||||
"\n",
|
||||
"* `api_endpoint`: AstraDB API endpoint. Looks like `https://01234567-89ab-cdef-0123-456789abcdef-us-east1.apps.astra.datastax.com`\n",
|
||||
"* `token`: AstraDB token. Looks like `AstraCS:6gBhNmsk135....`\n",
|
||||
"* `api_endpoint`: Astra DB API endpoint. Looks like `https://01234567-89ab-cdef-0123-456789abcdef-us-east1.apps.astra.datastax.com`\n",
|
||||
"* `token`: Astra DB token. Looks like `AstraCS:aBcD0123...`\n",
|
||||
"* `collection_name` : AstraDB collection name\n",
|
||||
"* `namespace`: (Optional) AstraDB namespace\n",
|
||||
"* `namespace`: (Optional) AstraDB namespace (called _keyspace_ in Astra DB)\n",
|
||||
"* `filter_criteria`: (Optional) Filter used in the find query\n",
|
||||
"* `projection`: (Optional) Projection used in the find query\n",
|
||||
"* `find_options`: (Optional) Options used in the find query\n",
|
||||
"* `nb_prefetched`: (Optional) Number of documents pre-fetched by the loader\n",
|
||||
"* `limit`: (Optional) Maximum number of documents to retrieve\n",
|
||||
"* `extraction_function`: (Optional) A function to convert the AstraDB document to the LangChain `page_content` string. Defaults to `json.dumps`\n",
|
||||
"\n",
|
||||
"The following metadata is set to the LangChain Documents metadata output:\n",
|
||||
"The loader sets the following metadata for the documents it reads:\n",
|
||||
"\n",
|
||||
"```python\n",
|
||||
"{\n",
|
||||
" metadata : {\n",
|
||||
" \"namespace\": \"...\", \n",
|
||||
" \"api_endpoint\": \"...\", \n",
|
||||
" \"collection\": \"...\"\n",
|
||||
" }\n",
|
||||
"metadata={\n",
|
||||
" \"namespace\": \"...\", \n",
|
||||
" \"api_endpoint\": \"...\", \n",
|
||||
" \"collection\": \"...\"\n",
|
||||
"}\n",
|
||||
"```"
|
||||
]
|
||||
},
|
||||
{
|
||||
"cell_type": "markdown",
|
||||
"metadata": {},
|
||||
"source": [
|
||||
"## Setup"
|
||||
]
|
||||
},
|
||||
{
|
||||
"cell_type": "code",
|
||||
"execution_count": 1,
|
||||
"metadata": {},
|
||||
"outputs": [],
|
||||
"source": [
|
||||
"!pip install \"langchain-astradb>=0.6,<0.7\""
|
||||
]
|
||||
},
|
||||
{
|
||||
"attachments": {},
|
||||
"cell_type": "markdown",
|
||||
@ -71,24 +84,43 @@
|
||||
},
|
||||
{
|
||||
"cell_type": "code",
|
||||
"execution_count": null,
|
||||
"execution_count": 2,
|
||||
"metadata": {},
|
||||
"outputs": [],
|
||||
"source": [
|
||||
"from langchain_community.document_loaders import AstraDBLoader"
|
||||
"from langchain_astradb import AstraDBLoader"
|
||||
]
|
||||
},
|
||||
{
|
||||
"cell_type": "markdown",
|
||||
"metadata": {},
|
||||
"source": [
|
||||
"[**API Reference:** `AstraDBLoader`](https://python.langchain.com/api_reference/astradb/document_loaders/langchain_astradb.document_loaders.AstraDBLoader.html#langchain_astradb.document_loaders.AstraDBLoader)"
|
||||
]
|
||||
},
|
||||
{
|
||||
"cell_type": "code",
|
||||
"execution_count": 4,
|
||||
"execution_count": 3,
|
||||
"metadata": {
|
||||
"ExecuteTime": {
|
||||
"end_time": "2024-01-08T12:41:22.643335Z",
|
||||
"start_time": "2024-01-08T12:40:57.759116Z"
|
||||
},
|
||||
"collapsed": false
|
||||
"collapsed": false,
|
||||
"jupyter": {
|
||||
"outputs_hidden": false
|
||||
}
|
||||
},
|
||||
"outputs": [],
|
||||
"outputs": [
|
||||
{
|
||||
"name": "stdin",
|
||||
"output_type": "stream",
|
||||
"text": [
|
||||
"ASTRA_DB_API_ENDPOINT = https://01234567-89ab-cdef-0123-456789abcdef-us-east1.apps.astra.datastax.com\n",
|
||||
"ASTRA_DB_APPLICATION_TOKEN = ········\n"
|
||||
]
|
||||
}
|
||||
],
|
||||
"source": [
|
||||
"from getpass import getpass\n",
|
||||
"\n",
|
||||
@ -98,7 +130,7 @@
|
||||
},
|
||||
{
|
||||
"cell_type": "code",
|
||||
"execution_count": 6,
|
||||
"execution_count": 4,
|
||||
"metadata": {
|
||||
"ExecuteTime": {
|
||||
"end_time": "2024-01-08T12:42:25.395162Z",
|
||||
@ -112,19 +144,22 @@
|
||||
" token=ASTRA_DB_APPLICATION_TOKEN,\n",
|
||||
" collection_name=\"movie_reviews\",\n",
|
||||
" projection={\"title\": 1, \"reviewtext\": 1},\n",
|
||||
" find_options={\"limit\": 10},\n",
|
||||
" limit=10,\n",
|
||||
")"
|
||||
]
|
||||
},
|
||||
{
|
||||
"cell_type": "code",
|
||||
"execution_count": 7,
|
||||
"execution_count": 5,
|
||||
"metadata": {
|
||||
"ExecuteTime": {
|
||||
"end_time": "2024-01-08T12:42:30.236489Z",
|
||||
"start_time": "2024-01-08T12:42:29.612133Z"
|
||||
},
|
||||
"collapsed": false
|
||||
"collapsed": false,
|
||||
"jupyter": {
|
||||
"outputs_hidden": false
|
||||
}
|
||||
},
|
||||
"outputs": [],
|
||||
"source": [
|
||||
@ -133,7 +168,7 @@
|
||||
},
|
||||
{
|
||||
"cell_type": "code",
|
||||
"execution_count": 8,
|
||||
"execution_count": 6,
|
||||
"metadata": {
|
||||
"ExecuteTime": {
|
||||
"end_time": "2024-01-08T12:42:31.369394Z",
|
||||
@ -144,10 +179,10 @@
|
||||
{
|
||||
"data": {
|
||||
"text/plain": [
|
||||
"Document(page_content='{\"_id\": \"659bdffa16cbc4586b11a423\", \"title\": \"Dangerous Men\", \"reviewtext\": \"\\\\\"Dangerous Men,\\\\\" the picture\\'s production notes inform, took 26 years to reach the big screen. After having seen it, I wonder: What was the rush?\"}', metadata={'namespace': 'default_keyspace', 'api_endpoint': 'https://01234567-89ab-cdef-0123-456789abcdef-us-east1.apps.astra.datastax.com', 'collection': 'movie_reviews'})"
|
||||
"Document(metadata={'namespace': 'default_keyspace', 'api_endpoint': 'https://01234567-89ab-cdef-0123-456789abcdef-us-east1.apps.astra.datastax.com', 'collection': 'movie_reviews'}, page_content='{\"_id\": \"659bdffa16cbc4586b11a423\", \"title\": \"Dangerous Men\", \"reviewtext\": \"\\\\\"Dangerous Men,\\\\\" the picture\\'s production notes inform, took 26 years to reach the big screen. After having seen it, I wonder: What was the rush?\"}')"
|
||||
]
|
||||
},
|
||||
"execution_count": 8,
|
||||
"execution_count": 7,
|
||||
"metadata": {},
|
||||
"output_type": "execute_result"
|
||||
}
|
||||
@ -179,7 +214,7 @@
|
||||
"name": "python",
|
||||
"nbconvert_exporter": "python",
|
||||
"pygments_lexer": "ipython3",
|
||||
"version": "3.9.18"
|
||||
"version": "3.12.8"
|
||||
}
|
||||
},
|
||||
"nbformat": 4,
|
||||
|
@ -49,7 +49,14 @@
|
||||
"metadata": {},
|
||||
"outputs": [],
|
||||
"source": [
|
||||
"from langchain_community.document_loaders import BrowserbaseLoader"
|
||||
"import os\n",
|
||||
"\n",
|
||||
"from langchain_community.document_loaders import BrowserbaseLoader\n",
|
||||
"\n",
|
||||
"load_dotenv()\n",
|
||||
"\n",
|
||||
"BROWSERBASE_API_KEY = os.getenv(\"BROWSERBASE_API_KEY\")\n",
|
||||
"BROWSERBASE_PROJECT_ID = os.getenv(\"BROWSERBASE_PROJECT_ID\")"
|
||||
]
|
||||
},
|
||||
{
|
||||
@ -59,6 +66,8 @@
|
||||
"outputs": [],
|
||||
"source": [
|
||||
"loader = BrowserbaseLoader(\n",
|
||||
" api_key=BROWSERBASE_API_KEY,\n",
|
||||
" project_id=BROWSERBASE_PROJECT_ID,\n",
|
||||
" urls=[\n",
|
||||
" \"https://example.com\",\n",
|
||||
" ],\n",
|
||||
@ -78,52 +87,11 @@
|
||||
"\n",
|
||||
"- `urls` Required. A list of URLs to fetch.\n",
|
||||
"- `text_content` Retrieve only text content. Default is `False`.\n",
|
||||
"- `api_key` Optional. Browserbase API key. Default is `BROWSERBASE_API_KEY` env variable.\n",
|
||||
"- `project_id` Optional. Browserbase Project ID. Default is `BROWSERBASE_PROJECT_ID` env variable.\n",
|
||||
"- `api_key` Browserbase API key. Default is `BROWSERBASE_API_KEY` env variable.\n",
|
||||
"- `project_id` Browserbase Project ID. Default is `BROWSERBASE_PROJECT_ID` env variable.\n",
|
||||
"- `session_id` Optional. Provide an existing Session ID.\n",
|
||||
"- `proxy` Optional. Enable/Disable Proxies."
|
||||
]
|
||||
},
|
||||
{
|
||||
"cell_type": "markdown",
|
||||
"metadata": {},
|
||||
"source": [
|
||||
"## Loading images\n",
|
||||
"\n",
|
||||
"You can also load screenshots of webpages (as bytes) for multi-modal models.\n",
|
||||
"\n",
|
||||
"Full example using GPT-4V:"
|
||||
]
|
||||
},
|
||||
{
|
||||
"cell_type": "code",
|
||||
"execution_count": null,
|
||||
"metadata": {},
|
||||
"outputs": [],
|
||||
"source": [
|
||||
"from browserbase import Browserbase\n",
|
||||
"from browserbase.helpers.gpt4 import GPT4VImage, GPT4VImageDetail\n",
|
||||
"from langchain_core.messages import HumanMessage\n",
|
||||
"from langchain_openai import ChatOpenAI\n",
|
||||
"\n",
|
||||
"chat = ChatOpenAI(model=\"gpt-4-vision-preview\", max_tokens=256)\n",
|
||||
"browser = Browserbase()\n",
|
||||
"\n",
|
||||
"screenshot = browser.screenshot(\"https://browserbase.com\")\n",
|
||||
"\n",
|
||||
"result = chat.invoke(\n",
|
||||
" [\n",
|
||||
" HumanMessage(\n",
|
||||
" content=[\n",
|
||||
" {\"type\": \"text\", \"text\": \"What color is the logo?\"},\n",
|
||||
" GPT4VImage(screenshot, GPT4VImageDetail.auto),\n",
|
||||
" ]\n",
|
||||
" )\n",
|
||||
" ]\n",
|
||||
")\n",
|
||||
"\n",
|
||||
"print(result.content)"
|
||||
]
|
||||
}
|
||||
],
|
||||
"metadata": {
|
||||
|
@ -36,10 +36,7 @@
|
||||
"pip install oracledb"
|
||||
],
|
||||
"metadata": {
|
||||
"collapsed": false,
|
||||
"pycharm": {
|
||||
"is_executing": true
|
||||
}
|
||||
"collapsed": false
|
||||
}
|
||||
},
|
||||
{
|
||||
@ -51,10 +48,7 @@
|
||||
"from settings import s"
|
||||
],
|
||||
"metadata": {
|
||||
"collapsed": false,
|
||||
"pycharm": {
|
||||
"is_executing": true
|
||||
}
|
||||
"collapsed": false
|
||||
}
|
||||
},
|
||||
{
|
||||
@ -97,16 +91,14 @@
|
||||
"doc_2 = doc_loader_2.load()"
|
||||
],
|
||||
"metadata": {
|
||||
"collapsed": false,
|
||||
"pycharm": {
|
||||
"is_executing": true
|
||||
}
|
||||
"collapsed": false
|
||||
}
|
||||
},
|
||||
{
|
||||
"cell_type": "markdown",
|
||||
"source": [
|
||||
"With TLS authentication, wallet_location and wallet_password are not required."
|
||||
"With TLS authentication, wallet_location and wallet_password are not required.\n",
|
||||
"Bind variable option is provided by argument \"parameters\"."
|
||||
],
|
||||
"metadata": {
|
||||
"collapsed": false
|
||||
@ -117,6 +109,8 @@
|
||||
"execution_count": null,
|
||||
"outputs": [],
|
||||
"source": [
|
||||
"SQL_QUERY = \"select channel_id, channel_desc from sh.channels where channel_desc = :1 fetch first 5 rows only\"\n",
|
||||
"\n",
|
||||
"doc_loader_3 = OracleAutonomousDatabaseLoader(\n",
|
||||
" query=SQL_QUERY,\n",
|
||||
" user=s.USERNAME,\n",
|
||||
@ -124,6 +118,7 @@
|
||||
" schema=s.SCHEMA,\n",
|
||||
" config_dir=s.CONFIG_DIR,\n",
|
||||
" tns_name=s.TNS_NAME,\n",
|
||||
" parameters=[\"Direct Sales\"],\n",
|
||||
")\n",
|
||||
"doc_3 = doc_loader_3.load()\n",
|
||||
"\n",
|
||||
@ -133,6 +128,7 @@
|
||||
" password=s.PASSWORD,\n",
|
||||
" schema=s.SCHEMA,\n",
|
||||
" connection_string=s.CONNECTION_STRING,\n",
|
||||
" parameters=[\"Direct Sales\"],\n",
|
||||
")\n",
|
||||
"doc_4 = doc_loader_4.load()"
|
||||
],
|
||||
|
187
docs/docs/integrations/document_loaders/singlestore.ipynb
Normal file
187
docs/docs/integrations/document_loaders/singlestore.ipynb
Normal file
@ -0,0 +1,187 @@
|
||||
{
|
||||
"cells": [
|
||||
{
|
||||
"cell_type": "markdown",
|
||||
"metadata": {},
|
||||
"source": [
|
||||
"---\n",
|
||||
"sidebar_label: SingleStore\n",
|
||||
"---"
|
||||
]
|
||||
},
|
||||
{
|
||||
"cell_type": "markdown",
|
||||
"metadata": {},
|
||||
"source": [
|
||||
"# SingleStoreLoader\n",
|
||||
"\n",
|
||||
"The `SingleStoreLoader` allows you to load documents directly from a SingleStore database table. It is part of the `langchain-singlestore` integration package.\n",
|
||||
"\n",
|
||||
"## Overview\n",
|
||||
"\n",
|
||||
"### Integration Details\n",
|
||||
"\n",
|
||||
"| Class | Package | JS Support |\n",
|
||||
"| :--- | :--- | :---: |\n",
|
||||
"| `SingleStoreLoader` | `langchain_singlestore` | ❌ |\n",
|
||||
"\n",
|
||||
"### Features\n",
|
||||
"- Load documents lazily to handle large datasets efficiently.\n",
|
||||
"- Supports native asynchronous operations.\n",
|
||||
"- Easily configurable to work with different database schemas.\n",
|
||||
"\n",
|
||||
"## Setup\n",
|
||||
"\n",
|
||||
"To use the `SingleStoreLoader`, you need to install the `langchain-singlestore` package. Follow the installation instructions below."
|
||||
]
|
||||
},
|
||||
{
|
||||
"cell_type": "markdown",
|
||||
"metadata": {},
|
||||
"source": [
|
||||
"### Installation\n",
|
||||
"\n",
|
||||
"Install **langchain_singlestore**."
|
||||
]
|
||||
},
|
||||
{
|
||||
"cell_type": "code",
|
||||
"execution_count": null,
|
||||
"metadata": {},
|
||||
"outputs": [],
|
||||
"source": [
|
||||
"%pip install -qU langchain_singlestore"
|
||||
]
|
||||
},
|
||||
{
|
||||
"cell_type": "markdown",
|
||||
"metadata": {},
|
||||
"source": [
|
||||
"## Initialization\n",
|
||||
"\n",
|
||||
"To initialize `SingleStoreLoader`, you need to provide connection parameters for the SingleStore database and specify the table and fields to load documents from.\n",
|
||||
"\n",
|
||||
"### Required Parameters:\n",
|
||||
"- **host** (`str`): Hostname, IP address, or URL for the database.\n",
|
||||
"- **table_name** (`str`): Name of the table to query. Defaults to `embeddings`.\n",
|
||||
"- **content_field** (`str`): Field containing document content. Defaults to `content`.\n",
|
||||
"- **metadata_field** (`str`): Field containing document metadata. Defaults to `metadata`.\n",
|
||||
"\n",
|
||||
"### Optional Parameters:\n",
|
||||
"- **id_field** (`str`): Field containing document IDs. Defaults to `id`.\n",
|
||||
"\n",
|
||||
"### Connection Pool Parameters:\n",
|
||||
"- **pool_size** (`int`): Number of active connections in the pool. Defaults to `5`.\n",
|
||||
"- **max_overflow** (`int`): Maximum connections beyond `pool_size`. Defaults to `10`.\n",
|
||||
"- **timeout** (`float`): Connection timeout in seconds. Defaults to `30`.\n",
|
||||
"\n",
|
||||
"### Additional Options:\n",
|
||||
"- **pure_python** (`bool`): Enables pure Python mode.\n",
|
||||
"- **local_infile** (`bool`): Allows local file uploads.\n",
|
||||
"- **charset** (`str`): Character set for string values.\n",
|
||||
"- **ssl_key**, **ssl_cert**, **ssl_ca** (`str`): Paths to SSL files.\n",
|
||||
"- **ssl_disabled** (`bool`): Disables SSL.\n",
|
||||
"- **ssl_verify_cert** (`bool`): Verifies server's certificate.\n",
|
||||
"- **ssl_verify_identity** (`bool`): Verifies server's identity.\n",
|
||||
"- **autocommit** (`bool`): Enables autocommits.\n",
|
||||
"- **results_type** (`str`): Structure of query results (e.g., `tuples`, `dicts`)."
|
||||
]
|
||||
},
|
||||
{
|
||||
"cell_type": "code",
|
||||
"execution_count": null,
|
||||
"metadata": {},
|
||||
"outputs": [],
|
||||
"source": [
|
||||
"from langchain_singlestore.document_loaders import SingleStoreLoader\n",
|
||||
"\n",
|
||||
"loader = SingleStoreLoader(\n",
|
||||
" host=\"127.0.0.1:3306/db\",\n",
|
||||
" table_name=\"documents\",\n",
|
||||
" content_field=\"content\",\n",
|
||||
" metadata_field=\"metadata\",\n",
|
||||
" id_field=\"id\",\n",
|
||||
")"
|
||||
]
|
||||
},
|
||||
{
|
||||
"cell_type": "markdown",
|
||||
"metadata": {},
|
||||
"source": [
|
||||
"## Load"
|
||||
]
|
||||
},
|
||||
{
|
||||
"cell_type": "code",
|
||||
"execution_count": null,
|
||||
"metadata": {},
|
||||
"outputs": [],
|
||||
"source": [
|
||||
"docs = loader.load()\n",
|
||||
"docs[0]"
|
||||
]
|
||||
},
|
||||
{
|
||||
"cell_type": "code",
|
||||
"execution_count": null,
|
||||
"metadata": {},
|
||||
"outputs": [],
|
||||
"source": [
|
||||
"print(docs[0].metadata)"
|
||||
]
|
||||
},
|
||||
{
|
||||
"cell_type": "markdown",
|
||||
"metadata": {},
|
||||
"source": [
|
||||
"## Lazy Load"
|
||||
]
|
||||
},
|
||||
{
|
||||
"cell_type": "code",
|
||||
"execution_count": null,
|
||||
"metadata": {},
|
||||
"outputs": [],
|
||||
"source": [
|
||||
"page = []\n",
|
||||
"for doc in loader.lazy_load():\n",
|
||||
" page.append(doc)\n",
|
||||
" if len(page) >= 10:\n",
|
||||
" # do some paged operation, e.g.\n",
|
||||
" # index.upsert(page)\n",
|
||||
"\n",
|
||||
" page = []"
|
||||
]
|
||||
},
|
||||
{
|
||||
"cell_type": "markdown",
|
||||
"metadata": {},
|
||||
"source": [
|
||||
"## API reference\n",
|
||||
"\n",
|
||||
"For detailed documentation of all SingleStore Document Loader features and configurations head to the github page: [https://github.com/singlestore-labs/langchain-singlestore/](https://github.com/singlestore-labs/langchain-singlestore/)"
|
||||
]
|
||||
}
|
||||
],
|
||||
"metadata": {
|
||||
"kernelspec": {
|
||||
"display_name": "Python 3 (ipykernel)",
|
||||
"language": "python",
|
||||
"name": "python3"
|
||||
},
|
||||
"language_info": {
|
||||
"codemirror_mode": {
|
||||
"name": "ipython",
|
||||
"version": 3
|
||||
},
|
||||
"file_extension": ".py",
|
||||
"mimetype": "text/x-python",
|
||||
"name": "python",
|
||||
"nbconvert_exporter": "python",
|
||||
"pygments_lexer": "ipython3",
|
||||
"version": "3.11.9"
|
||||
}
|
||||
},
|
||||
"nbformat": 4,
|
||||
"nbformat_minor": 4
|
||||
}
|
@ -11,39 +11,41 @@
|
||||
"cell_type": "markdown",
|
||||
"metadata": {},
|
||||
"source": [
|
||||
"[RankLLM](https://github.com/castorini/rank_llm) offers a suite of listwise rerankers, albeit with focus on open source LLMs finetuned for the task - RankVicuna and RankZephyr being two of them."
|
||||
"**[RankLLM](https://github.com/castorini/rank_llm)** is a **flexible reranking framework** supporting **listwise, pairwise, and pointwise ranking models**. It includes **RankVicuna, RankZephyr, MonoT5, DuoT5, LiT5, and FirstMistral**, with integration for **FastChat, vLLM, SGLang, and TensorRT-LLM** for efficient inference. RankLLM is optimized for **retrieval and ranking tasks**, leveraging both **open-source LLMs** and proprietary rerankers like **RankGPT and RankGemini**. It supports **batched inference, first-token reranking, and retrieval via BM25 and SPLADE**.\n",
|
||||
"\n",
|
||||
"> **Note:** If using the built-in retriever, RankLLM requires **Pyserini, JDK 21, PyTorch, and Faiss** for retrieval functionality."
|
||||
]
|
||||
},
|
||||
{
|
||||
"cell_type": "code",
|
||||
"execution_count": 1,
|
||||
"execution_count": null,
|
||||
"metadata": {},
|
||||
"outputs": [],
|
||||
"source": [
|
||||
"%pip install --upgrade --quiet rank_llm"
|
||||
"%pip install --upgrade --quiet rank_llm"
|
||||
]
|
||||
},
|
||||
{
|
||||
"cell_type": "code",
|
||||
"execution_count": 2,
|
||||
"execution_count": null,
|
||||
"metadata": {},
|
||||
"outputs": [],
|
||||
"source": [
|
||||
"%pip install --upgrade --quiet langchain_openai"
|
||||
"%pip install --upgrade --quiet langchain_openai"
|
||||
]
|
||||
},
|
||||
{
|
||||
"cell_type": "code",
|
||||
"execution_count": 3,
|
||||
"execution_count": null,
|
||||
"metadata": {},
|
||||
"outputs": [],
|
||||
"source": [
|
||||
"%pip install --upgrade --quiet faiss-cpu"
|
||||
"%pip install --upgrade --quiet faiss-cpu"
|
||||
]
|
||||
},
|
||||
{
|
||||
"cell_type": "code",
|
||||
"execution_count": 4,
|
||||
"execution_count": null,
|
||||
"metadata": {},
|
||||
"outputs": [],
|
||||
"source": [
|
||||
@ -56,7 +58,7 @@
|
||||
},
|
||||
{
|
||||
"cell_type": "code",
|
||||
"execution_count": 5,
|
||||
"execution_count": null,
|
||||
"metadata": {},
|
||||
"outputs": [],
|
||||
"source": [
|
||||
@ -64,7 +66,7 @@
|
||||
"def pretty_print_docs(docs):\n",
|
||||
" print(\n",
|
||||
" f\"\\n{'-' * 100}\\n\".join(\n",
|
||||
" [f\"Document {i+1}:\\n\\n\" + d.page_content for i, d in enumerate(docs)]\n",
|
||||
" [f\"Document {i + 1}:\\n\\n\" + d.page_content for i, d in enumerate(docs)]\n",
|
||||
" )\n",
|
||||
" )"
|
||||
]
|
||||
@ -79,9 +81,17 @@
|
||||
},
|
||||
{
|
||||
"cell_type": "code",
|
||||
"execution_count": 6,
|
||||
"execution_count": null,
|
||||
"metadata": {},
|
||||
"outputs": [],
|
||||
"outputs": [
|
||||
{
|
||||
"name": "stdout",
|
||||
"output_type": "stream",
|
||||
"text": [
|
||||
"2025-02-22 15:28:58,344 - INFO - HTTP Request: POST https://api.openai.com/v1/embeddings \"HTTP/1.1 200 OK\"\n"
|
||||
]
|
||||
}
|
||||
],
|
||||
"source": [
|
||||
"from langchain_community.document_loaders import TextLoader\n",
|
||||
"from langchain_community.vectorstores import FAISS\n",
|
||||
@ -114,14 +124,14 @@
|
||||
},
|
||||
{
|
||||
"cell_type": "code",
|
||||
"execution_count": 23,
|
||||
"execution_count": null,
|
||||
"metadata": {},
|
||||
"outputs": [
|
||||
{
|
||||
"name": "stderr",
|
||||
"name": "stdout",
|
||||
"output_type": "stream",
|
||||
"text": [
|
||||
"2025-02-17 04:37:08,458 - INFO - HTTP Request: POST https://api.openai.com/v1/embeddings \"HTTP/1.1 200 OK\"\n"
|
||||
"2025-02-22 15:29:00,892 - INFO - HTTP Request: POST https://api.openai.com/v1/embeddings \"HTTP/1.1 200 OK\"\n"
|
||||
]
|
||||
},
|
||||
{
|
||||
@ -331,27 +341,41 @@
|
||||
"cell_type": "markdown",
|
||||
"metadata": {},
|
||||
"source": [
|
||||
"Retrieval + Reranking with RankZephyr"
|
||||
"RankZephyr performs listwise reranking for improved retrieval quality but requires at least 24GB of VRAM to run efficiently."
|
||||
]
|
||||
},
|
||||
{
|
||||
"cell_type": "code",
|
||||
"execution_count": 12,
|
||||
"execution_count": null,
|
||||
"metadata": {},
|
||||
"outputs": [],
|
||||
"outputs": [
|
||||
{
|
||||
"name": "stdout",
|
||||
"output_type": "stream",
|
||||
"text": [
|
||||
"Downloading shards: 100%|██████████| 3/3 [00:00<00:00, 2674.37it/s]\n",
|
||||
"Loading checkpoint shards: 100%|██████████| 3/3 [01:49<00:00, 36.39s/it]\n"
|
||||
]
|
||||
}
|
||||
],
|
||||
"source": [
|
||||
"import torch\n",
|
||||
"from langchain.retrievers.contextual_compression import ContextualCompressionRetriever\n",
|
||||
"from langchain_community.document_compressors.rankllm_rerank import RankLLMRerank\n",
|
||||
"\n",
|
||||
"compressor = RankLLMRerank(top_n=3, model=\"zephyr\")\n",
|
||||
"torch.cuda.empty_cache()\n",
|
||||
"\n",
|
||||
"compressor = RankLLMRerank(top_n=3, model=\"rank_zephyr\")\n",
|
||||
"compression_retriever = ContextualCompressionRetriever(\n",
|
||||
" base_compressor=compressor, base_retriever=retriever\n",
|
||||
")"
|
||||
")\n",
|
||||
"\n",
|
||||
"del compressor"
|
||||
]
|
||||
},
|
||||
{
|
||||
"cell_type": "code",
|
||||
"execution_count": 9,
|
||||
"execution_count": null,
|
||||
"metadata": {},
|
||||
"outputs": [
|
||||
{
|
||||
@ -386,7 +410,7 @@
|
||||
]
|
||||
},
|
||||
{
|
||||
"name": "stderr",
|
||||
"name": "stdout",
|
||||
"output_type": "stream",
|
||||
"text": [
|
||||
"\n"
|
||||
@ -407,7 +431,7 @@
|
||||
},
|
||||
{
|
||||
"cell_type": "code",
|
||||
"execution_count": 10,
|
||||
"execution_count": null,
|
||||
"metadata": {},
|
||||
"outputs": [
|
||||
{
|
||||
@ -432,7 +456,7 @@
|
||||
" llm=ChatOpenAI(temperature=0), retriever=compression_retriever\n",
|
||||
")\n",
|
||||
"\n",
|
||||
"chain({\"query\": query})"
|
||||
"chain.invoke({\"query\": query})"
|
||||
]
|
||||
},
|
||||
{
|
||||
@ -451,9 +475,16 @@
|
||||
},
|
||||
{
|
||||
"cell_type": "code",
|
||||
"execution_count": 11,
|
||||
"execution_count": null,
|
||||
"metadata": {},
|
||||
"outputs": [
|
||||
{
|
||||
"name": "stdout",
|
||||
"output_type": "stream",
|
||||
"text": [
|
||||
"2025-02-22 15:01:29,469 - INFO - HTTP Request: POST https://api.openai.com/v1/embeddings \"HTTP/1.1 200 OK\"\n"
|
||||
]
|
||||
},
|
||||
{
|
||||
"name": "stdout",
|
||||
"output_type": "stream",
|
||||
@ -683,7 +714,7 @@
|
||||
},
|
||||
{
|
||||
"cell_type": "code",
|
||||
"execution_count": 20,
|
||||
"execution_count": null,
|
||||
"metadata": {},
|
||||
"outputs": [],
|
||||
"source": [
|
||||
@ -698,9 +729,18 @@
|
||||
},
|
||||
{
|
||||
"cell_type": "code",
|
||||
"execution_count": 13,
|
||||
"execution_count": null,
|
||||
"metadata": {},
|
||||
"outputs": [
|
||||
{
|
||||
"name": "stdout",
|
||||
"output_type": "stream",
|
||||
"text": [
|
||||
"2025-02-22 15:01:38,554 - INFO - HTTP Request: POST https://api.openai.com/v1/embeddings \"HTTP/1.1 200 OK\"\n",
|
||||
" 0%| | 0/1 [00:00<?, ?it/s]2025-02-22 15:01:43,704 - INFO - HTTP Request: POST https://api.openai.com/v1/chat/completions \"HTTP/1.1 200 OK\"\n",
|
||||
"100%|██████████| 1/1 [00:05<00:00, 5.15s/it]"
|
||||
]
|
||||
},
|
||||
{
|
||||
"name": "stdout",
|
||||
"output_type": "stream",
|
||||
@ -727,7 +767,7 @@
|
||||
]
|
||||
},
|
||||
{
|
||||
"name": "stderr",
|
||||
"name": "stdout",
|
||||
"output_type": "stream",
|
||||
"text": [
|
||||
"\n"
|
||||
@ -748,15 +788,14 @@
|
||||
},
|
||||
{
|
||||
"cell_type": "code",
|
||||
"execution_count": 22,
|
||||
"execution_count": null,
|
||||
"metadata": {},
|
||||
"outputs": [
|
||||
{
|
||||
"name": "stderr",
|
||||
"name": "stdout",
|
||||
"output_type": "stream",
|
||||
"text": [
|
||||
"/tmp/ipykernel_2153001/1437145854.py:10: LangChainDeprecationWarning: The method `Chain.__call__` was deprecated in langchain 0.1.0 and will be removed in 1.0. Use :meth:`~invoke` instead.\n",
|
||||
" chain({\"query\": query})\n",
|
||||
" chain.invoke({\"query\": query})\n",
|
||||
"2025-02-17 04:30:00,016 - INFO - HTTP Request: POST https://api.openai.com/v1/embeddings \"HTTP/1.1 200 OK\"\n",
|
||||
" 0%| | 0/1 [00:00<?, ?it/s]2025-02-17 04:30:01,649 - INFO - HTTP Request: POST https://api.openai.com/v1/chat/completions \"HTTP/1.1 200 OK\"\n",
|
||||
"100%|██████████| 1/1 [00:01<00:00, 1.63s/it]\n",
|
||||
@ -785,13 +824,13 @@
|
||||
" llm=ChatOpenAI(temperature=0), retriever=compression_retriever\n",
|
||||
")\n",
|
||||
"\n",
|
||||
"chain({\"query\": query})"
|
||||
"chain.invoke({\"query\": query})"
|
||||
]
|
||||
}
|
||||
],
|
||||
"metadata": {
|
||||
"kernelspec": {
|
||||
"display_name": "rankllm",
|
||||
"display_name": "Python 3",
|
||||
"language": "python",
|
||||
"name": "python3"
|
||||
},
|
||||
@ -805,7 +844,7 @@
|
||||
"name": "python",
|
||||
"nbconvert_exporter": "python",
|
||||
"pygments_lexer": "ipython3",
|
||||
"version": "3.10.14"
|
||||
"version": "3.13.2"
|
||||
}
|
||||
},
|
||||
"nbformat": 4,
|
||||
|
@ -2525,7 +2525,17 @@
|
||||
"source": [
|
||||
"## `SingleStoreDB` semantic cache\n",
|
||||
"\n",
|
||||
"You can use [SingleStoreDB](https://python.langchain.com/docs/integrations/vectorstores/singlestoredb/) as a semantic cache to cache prompts and responses."
|
||||
"You can use [SingleStore](https://python.langchain.com/docs/integrations/vectorstores/singlestore/) as a semantic cache to cache prompts and responses."
|
||||
]
|
||||
},
|
||||
{
|
||||
"cell_type": "code",
|
||||
"execution_count": null,
|
||||
"id": "596e15e8",
|
||||
"metadata": {},
|
||||
"outputs": [],
|
||||
"source": [
|
||||
"%pip install -qU langchain-singlestore"
|
||||
]
|
||||
},
|
||||
{
|
||||
@ -2535,11 +2545,11 @@
|
||||
"metadata": {},
|
||||
"outputs": [],
|
||||
"source": [
|
||||
"from langchain_community.cache import SingleStoreDBSemanticCache\n",
|
||||
"from langchain_openai import OpenAIEmbeddings\n",
|
||||
"from langchain_singlestore.cache import SingleStoreSemanticCache\n",
|
||||
"\n",
|
||||
"set_llm_cache(\n",
|
||||
" SingleStoreDBSemanticCache(\n",
|
||||
" SingleStoreSemanticCache(\n",
|
||||
" embedding=OpenAIEmbeddings(),\n",
|
||||
" host=\"root:pass@localhost:3306/db\",\n",
|
||||
" )\n",
|
||||
@ -2669,7 +2679,7 @@
|
||||
"metadata": {},
|
||||
"outputs": [],
|
||||
"source": [
|
||||
"%pip install -qU langchain_couchbase couchbase"
|
||||
"%pip install -qU langchain_couchbase"
|
||||
]
|
||||
},
|
||||
{
|
||||
@ -3102,8 +3112,8 @@
|
||||
"|------------|---------|\n",
|
||||
"| langchain_astradb.cache | [AstraDBCache](https://python.langchain.com/api_reference/astradb/cache/langchain_astradb.cache.AstraDBCache.html) |\n",
|
||||
"| langchain_astradb.cache | [AstraDBSemanticCache](https://python.langchain.com/api_reference/astradb/cache/langchain_astradb.cache.AstraDBSemanticCache.html) |\n",
|
||||
"| langchain_community.cache | [AstraDBCache](https://python.langchain.com/api_reference/community/cache/langchain_community.cache.AstraDBCache.html) |\n",
|
||||
"| langchain_community.cache | [AstraDBSemanticCache](https://python.langchain.com/api_reference/community/cache/langchain_community.cache.AstraDBSemanticCache.html) |\n",
|
||||
"| langchain_community.cache | [AstraDBCache](https://python.langchain.com/api_reference/community/cache/langchain_community.cache.AstraDBCache.html) (deprecated since `langchain-community==0.0.28`) |\n",
|
||||
"| langchain_community.cache | [AstraDBSemanticCache](https://python.langchain.com/api_reference/community/cache/langchain_community.cache.AstraDBSemanticCache.html) (deprecated since `langchain-community==0.0.28`) |\n",
|
||||
"| langchain_community.cache | [AzureCosmosDBSemanticCache](https://python.langchain.com/api_reference/community/cache/langchain_community.cache.AzureCosmosDBSemanticCache.html) |\n",
|
||||
"| langchain_community.cache | [CassandraCache](https://python.langchain.com/api_reference/community/cache/langchain_community.cache.CassandraCache.html) |\n",
|
||||
"| langchain_community.cache | [CassandraSemanticCache](https://python.langchain.com/api_reference/community/cache/langchain_community.cache.CassandraSemanticCache.html) |\n",
|
||||
|
@ -90,7 +90,7 @@
|
||||
},
|
||||
{
|
||||
"cell_type": "code",
|
||||
"execution_count": 3,
|
||||
"execution_count": null,
|
||||
"id": "d285fd7f",
|
||||
"metadata": {},
|
||||
"outputs": [],
|
||||
@ -99,7 +99,7 @@
|
||||
"\n",
|
||||
"# Initialize a Fireworks model\n",
|
||||
"llm = Fireworks(\n",
|
||||
" model=\"accounts/fireworks/models/mixtral-8x7b-instruct\",\n",
|
||||
" model=\"accounts/fireworks/models/llama-v3p1-8b-instruct\",\n",
|
||||
" base_url=\"https://api.fireworks.ai/inference/v1/completions\",\n",
|
||||
")"
|
||||
]
|
||||
@ -176,7 +176,7 @@
|
||||
},
|
||||
{
|
||||
"cell_type": "code",
|
||||
"execution_count": 6,
|
||||
"execution_count": null,
|
||||
"id": "b801c20d",
|
||||
"metadata": {},
|
||||
"outputs": [
|
||||
@ -192,7 +192,7 @@
|
||||
"source": [
|
||||
"# Setting additional parameters: temperature, max_tokens, top_p\n",
|
||||
"llm = Fireworks(\n",
|
||||
" model=\"accounts/fireworks/models/mixtral-8x7b-instruct\",\n",
|
||||
" model=\"accounts/fireworks/models/llama-v3p1-8b-instruct\",\n",
|
||||
" temperature=0.7,\n",
|
||||
" max_tokens=15,\n",
|
||||
" top_p=1.0,\n",
|
||||
@ -218,7 +218,7 @@
|
||||
},
|
||||
{
|
||||
"cell_type": "code",
|
||||
"execution_count": 7,
|
||||
"execution_count": null,
|
||||
"id": "fd2c6bc1",
|
||||
"metadata": {},
|
||||
"outputs": [
|
||||
@ -235,7 +235,7 @@
|
||||
"from langchain_fireworks import Fireworks\n",
|
||||
"\n",
|
||||
"llm = Fireworks(\n",
|
||||
" model=\"accounts/fireworks/models/mixtral-8x7b-instruct\",\n",
|
||||
" model=\"accounts/fireworks/models/llama-v3p1-8b-instruct\",\n",
|
||||
" temperature=0.7,\n",
|
||||
" max_tokens=15,\n",
|
||||
" top_p=1.0,\n",
|
||||
|
266
docs/docs/integrations/llms/runpod.ipynb
Normal file
266
docs/docs/integrations/llms/runpod.ipynb
Normal file
@ -0,0 +1,266 @@
|
||||
{
|
||||
"cells": [
|
||||
{
|
||||
"cell_type": "markdown",
|
||||
"metadata": {},
|
||||
"source": [
|
||||
"# RunPod LLM"
|
||||
]
|
||||
},
|
||||
{
|
||||
"cell_type": "markdown",
|
||||
"metadata": {},
|
||||
"source": [
|
||||
"Get started with RunPod LLMs.\n",
|
||||
"\n",
|
||||
"## Overview\n",
|
||||
"\n",
|
||||
"This guide covers how to use the LangChain `RunPod` LLM class to interact with text generation models hosted on [RunPod Serverless](https://www.runpod.io/serverless-gpu)."
|
||||
]
|
||||
},
|
||||
{
|
||||
"cell_type": "markdown",
|
||||
"metadata": {},
|
||||
"source": [
|
||||
"## Setup\n",
|
||||
"\n",
|
||||
"1. **Install the package:**\n",
|
||||
" ```bash\n",
|
||||
" pip install -qU langchain-runpod\n",
|
||||
" ```\n",
|
||||
"2. **Deploy an LLM Endpoint:** Follow the setup steps in the [RunPod Provider Guide](/docs/integrations/providers/runpod#setup) to deploy a compatible text generation endpoint on RunPod Serverless and get its Endpoint ID.\n",
|
||||
"3. **Set Environment Variables:** Make sure `RUNPOD_API_KEY` and `RUNPOD_ENDPOINT_ID` are set."
|
||||
]
|
||||
},
|
||||
{
|
||||
"cell_type": "code",
|
||||
"execution_count": null,
|
||||
"metadata": {
|
||||
"vscode": {
|
||||
"languageId": "plaintext"
|
||||
}
|
||||
},
|
||||
"outputs": [],
|
||||
"source": [
|
||||
"import getpass\n",
|
||||
"import os\n",
|
||||
"\n",
|
||||
"# Make sure environment variables are set (or pass them directly to RunPod)\n",
|
||||
"if \"RUNPOD_API_KEY\" not in os.environ:\n",
|
||||
" os.environ[\"RUNPOD_API_KEY\"] = getpass.getpass(\"Enter your RunPod API Key: \")\n",
|
||||
"if \"RUNPOD_ENDPOINT_ID\" not in os.environ:\n",
|
||||
" os.environ[\"RUNPOD_ENDPOINT_ID\"] = input(\"Enter your RunPod Endpoint ID: \")"
|
||||
]
|
||||
},
|
||||
{
|
||||
"cell_type": "markdown",
|
||||
"metadata": {},
|
||||
"source": [
|
||||
"## Instantiation\n",
|
||||
"\n",
|
||||
"Initialize the `RunPod` class. You can pass model-specific parameters via `model_kwargs` and configure polling behavior."
|
||||
]
|
||||
},
|
||||
{
|
||||
"cell_type": "code",
|
||||
"execution_count": null,
|
||||
"metadata": {
|
||||
"vscode": {
|
||||
"languageId": "plaintext"
|
||||
}
|
||||
},
|
||||
"outputs": [],
|
||||
"source": [
|
||||
"from langchain_runpod import RunPod\n",
|
||||
"\n",
|
||||
"llm = RunPod(\n",
|
||||
" # runpod_endpoint_id can be passed here if not set in env\n",
|
||||
" model_kwargs={\n",
|
||||
" \"max_new_tokens\": 256,\n",
|
||||
" \"temperature\": 0.6,\n",
|
||||
" \"top_k\": 50,\n",
|
||||
" # Add other parameters supported by your endpoint handler\n",
|
||||
" },\n",
|
||||
" # Optional: Adjust polling\n",
|
||||
" # poll_interval=0.3,\n",
|
||||
" # max_polling_attempts=100\n",
|
||||
")"
|
||||
]
|
||||
},
|
||||
{
|
||||
"cell_type": "markdown",
|
||||
"metadata": {},
|
||||
"source": [
|
||||
"## Invocation\n",
|
||||
"\n",
|
||||
"Use the standard LangChain `.invoke()` and `.ainvoke()` methods to call the model. Streaming is also supported via `.stream()` and `.astream()` (simulated by polling the RunPod `/stream` endpoint)."
|
||||
]
|
||||
},
|
||||
{
|
||||
"cell_type": "code",
|
||||
"execution_count": null,
|
||||
"metadata": {
|
||||
"vscode": {
|
||||
"languageId": "plaintext"
|
||||
}
|
||||
},
|
||||
"outputs": [],
|
||||
"source": [
|
||||
"prompt = \"Write a tagline for an ice cream shop on the moon.\"\n",
|
||||
"\n",
|
||||
"# Invoke (Sync)\n",
|
||||
"try:\n",
|
||||
" response = llm.invoke(prompt)\n",
|
||||
" print(\"--- Sync Invoke Response ---\")\n",
|
||||
" print(response)\n",
|
||||
"except Exception as e:\n",
|
||||
" print(\n",
|
||||
" f\"Error invoking LLM: {e}. Ensure endpoint ID/API key are correct and endpoint is active/compatible.\"\n",
|
||||
" )"
|
||||
]
|
||||
},
|
||||
{
|
||||
"cell_type": "code",
|
||||
"execution_count": null,
|
||||
"metadata": {
|
||||
"vscode": {
|
||||
"languageId": "plaintext"
|
||||
}
|
||||
},
|
||||
"outputs": [],
|
||||
"source": [
|
||||
"# Stream (Sync, simulated via polling /stream)\n",
|
||||
"print(\"\\n--- Sync Stream Response ---\")\n",
|
||||
"try:\n",
|
||||
" for chunk in llm.stream(prompt):\n",
|
||||
" print(chunk, end=\"\", flush=True)\n",
|
||||
" print() # Newline\n",
|
||||
"except Exception as e:\n",
|
||||
" print(\n",
|
||||
" f\"\\nError streaming LLM: {e}. Ensure endpoint handler supports streaming output format.\"\n",
|
||||
" )"
|
||||
]
|
||||
},
|
||||
{
|
||||
"cell_type": "markdown",
|
||||
"metadata": {},
|
||||
"source": [
|
||||
"### Async Usage"
|
||||
]
|
||||
},
|
||||
{
|
||||
"cell_type": "code",
|
||||
"execution_count": null,
|
||||
"metadata": {
|
||||
"vscode": {
|
||||
"languageId": "plaintext"
|
||||
}
|
||||
},
|
||||
"outputs": [],
|
||||
"source": [
|
||||
"# AInvoke (Async)\n",
|
||||
"try:\n",
|
||||
" async_response = await llm.ainvoke(prompt)\n",
|
||||
" print(\"--- Async Invoke Response ---\")\n",
|
||||
" print(async_response)\n",
|
||||
"except Exception as e:\n",
|
||||
" print(f\"Error invoking LLM asynchronously: {e}.\")"
|
||||
]
|
||||
},
|
||||
{
|
||||
"cell_type": "code",
|
||||
"execution_count": null,
|
||||
"metadata": {
|
||||
"vscode": {
|
||||
"languageId": "plaintext"
|
||||
}
|
||||
},
|
||||
"outputs": [],
|
||||
"source": [
|
||||
"# AStream (Async)\n",
|
||||
"print(\"\\n--- Async Stream Response ---\")\n",
|
||||
"try:\n",
|
||||
" async for chunk in llm.astream(prompt):\n",
|
||||
" print(chunk, end=\"\", flush=True)\n",
|
||||
" print() # Newline\n",
|
||||
"except Exception as e:\n",
|
||||
" print(\n",
|
||||
" f\"\\nError streaming LLM asynchronously: {e}. Ensure endpoint handler supports streaming output format.\"\n",
|
||||
" )"
|
||||
]
|
||||
},
|
||||
{
|
||||
"cell_type": "markdown",
|
||||
"metadata": {},
|
||||
"source": [
|
||||
"## Chaining\n",
|
||||
"\n",
|
||||
"The LLM integrates seamlessly with LangChain Expression Language (LCEL) chains."
|
||||
]
|
||||
},
|
||||
{
|
||||
"cell_type": "code",
|
||||
"execution_count": null,
|
||||
"metadata": {
|
||||
"vscode": {
|
||||
"languageId": "plaintext"
|
||||
}
|
||||
},
|
||||
"outputs": [],
|
||||
"source": [
|
||||
"from langchain_core.output_parsers import StrOutputParser\n",
|
||||
"from langchain_core.prompts import PromptTemplate\n",
|
||||
"\n",
|
||||
"# Assumes 'llm' variable is instantiated from the 'Instantiation' cell\n",
|
||||
"prompt_template = PromptTemplate.from_template(\"Tell me a joke about {topic}\")\n",
|
||||
"parser = StrOutputParser()\n",
|
||||
"\n",
|
||||
"chain = prompt_template | llm | parser\n",
|
||||
"\n",
|
||||
"try:\n",
|
||||
" chain_response = chain.invoke({\"topic\": \"bears\"})\n",
|
||||
" print(\"--- Chain Response ---\")\n",
|
||||
" print(chain_response)\n",
|
||||
"except Exception as e:\n",
|
||||
" print(f\"Error running chain: {e}\")\n",
|
||||
"\n",
|
||||
"# Async chain\n",
|
||||
"try:\n",
|
||||
" async_chain_response = await chain.ainvoke({\"topic\": \"robots\"})\n",
|
||||
" print(\"--- Async Chain Response ---\")\n",
|
||||
" print(async_chain_response)\n",
|
||||
"except Exception as e:\n",
|
||||
" print(f\"Error running async chain: {e}\")"
|
||||
]
|
||||
},
|
||||
{
|
||||
"cell_type": "markdown",
|
||||
"metadata": {},
|
||||
"source": [
|
||||
"## Endpoint Considerations\n",
|
||||
"\n",
|
||||
"- **Input:** The endpoint handler should expect the prompt string within `{\"input\": {\"prompt\": \"...\", ...}}`.\n",
|
||||
"- **Output:** The handler should return the generated text within the `\"output\"` key of the final status response (e.g., `{\"output\": \"Generated text...\"}` or `{\"output\": {\"text\": \"...\"}}`).\n",
|
||||
"- **Streaming:** For simulated streaming via the `/stream` endpoint, the handler must populate the `\"stream\"` key in the status response with a list of chunk dictionaries, like `[{\"output\": \"token1\"}, {\"output\": \"token2\"}]`."
|
||||
]
|
||||
},
|
||||
{
|
||||
"cell_type": "markdown",
|
||||
"metadata": {},
|
||||
"source": [
|
||||
"## API reference\n",
|
||||
"\n",
|
||||
"For detailed documentation of the `RunPod` LLM class, parameters, and methods, refer to the source code or the generated API reference (if available).\n",
|
||||
"\n",
|
||||
"Link to source code: [https://github.com/runpod/langchain-runpod/blob/main/langchain_runpod/llms.py](https://github.com/runpod/langchain-runpod/blob/main/langchain_runpod/llms.py)"
|
||||
]
|
||||
}
|
||||
],
|
||||
"metadata": {
|
||||
"language_info": {
|
||||
"name": "python"
|
||||
}
|
||||
},
|
||||
"nbformat": 4,
|
||||
"nbformat_minor": 2
|
||||
}
|
@ -17,22 +17,22 @@
|
||||
"id": "f507f58b-bf22-4a48-8daf-68d869bcd1ba",
|
||||
"metadata": {},
|
||||
"source": [
|
||||
"## Setting up\n",
|
||||
"## Setup\n",
|
||||
"\n",
|
||||
"To run this notebook you need a running Astra DB. Get the connection secrets on your Astra dashboard:\n",
|
||||
"\n",
|
||||
"- the API Endpoint looks like `https://01234567-89ab-cdef-0123-456789abcdef-us-east1.apps.astra.datastax.com`;\n",
|
||||
"- the Token looks like `AstraCS:6gBhNmsk135...`."
|
||||
"- the Database Token looks like `AstraCS:aBcD0123...`."
|
||||
]
|
||||
},
|
||||
{
|
||||
"cell_type": "code",
|
||||
"execution_count": null,
|
||||
"execution_count": 1,
|
||||
"id": "d7092199",
|
||||
"metadata": {},
|
||||
"outputs": [],
|
||||
"source": [
|
||||
"%pip install --upgrade --quiet \"astrapy>=0.7.1 langchain-community\" "
|
||||
"!pip install \"langchain-astradb>=0.6,<0.7\""
|
||||
]
|
||||
},
|
||||
{
|
||||
@ -45,12 +45,12 @@
|
||||
},
|
||||
{
|
||||
"cell_type": "code",
|
||||
"execution_count": 1,
|
||||
"execution_count": 2,
|
||||
"id": "163d97f0",
|
||||
"metadata": {},
|
||||
"outputs": [
|
||||
{
|
||||
"name": "stdout",
|
||||
"name": "stdin",
|
||||
"output_type": "stream",
|
||||
"text": [
|
||||
"ASTRA_DB_API_ENDPOINT = https://01234567-89ab-cdef-0123-456789abcdef-us-east1.apps.astra.datastax.com\n",
|
||||
@ -65,14 +65,6 @@
|
||||
"ASTRA_DB_APPLICATION_TOKEN = getpass.getpass(\"ASTRA_DB_APPLICATION_TOKEN = \")"
|
||||
]
|
||||
},
|
||||
{
|
||||
"cell_type": "markdown",
|
||||
"id": "55860b2d",
|
||||
"metadata": {},
|
||||
"source": [
|
||||
"Depending on whether local or cloud-based Astra DB, create the corresponding database connection \"Session\" object."
|
||||
]
|
||||
},
|
||||
{
|
||||
"cell_type": "markdown",
|
||||
"id": "36c163e8",
|
||||
@ -83,12 +75,12 @@
|
||||
},
|
||||
{
|
||||
"cell_type": "code",
|
||||
"execution_count": 2,
|
||||
"execution_count": 3,
|
||||
"id": "d15e3302",
|
||||
"metadata": {},
|
||||
"outputs": [],
|
||||
"source": [
|
||||
"from langchain_community.chat_message_histories import AstraDBChatMessageHistory\n",
|
||||
"from langchain_astradb import AstraDBChatMessageHistory\n",
|
||||
"\n",
|
||||
"message_history = AstraDBChatMessageHistory(\n",
|
||||
" session_id=\"test-session\",\n",
|
||||
@ -98,22 +90,31 @@
|
||||
"\n",
|
||||
"message_history.add_user_message(\"hi!\")\n",
|
||||
"\n",
|
||||
"message_history.add_ai_message(\"whats up?\")"
|
||||
"message_history.add_ai_message(\"hello, how are you?\")"
|
||||
]
|
||||
},
|
||||
{
|
||||
"cell_type": "markdown",
|
||||
"id": "53acb4a8-d536-4a58-9fee-7d70033d9c81",
|
||||
"metadata": {},
|
||||
"source": [
|
||||
"[**API Reference:** `AstraDBChatMessageHistory`](https://python.langchain.com/api_reference/astradb/chat_message_histories/langchain_astradb.chat_message_histories.AstraDBChatMessageHistory.html#langchain_astradb.chat_message_histories.AstraDBChatMessageHistory)"
|
||||
]
|
||||
},
|
||||
{
|
||||
"cell_type": "code",
|
||||
"execution_count": 3,
|
||||
"execution_count": 4,
|
||||
"id": "64fc465e",
|
||||
"metadata": {},
|
||||
"outputs": [
|
||||
{
|
||||
"data": {
|
||||
"text/plain": [
|
||||
"[HumanMessage(content='hi!'), AIMessage(content='whats up?')]"
|
||||
"[HumanMessage(content='hi!', additional_kwargs={}, response_metadata={}),\n",
|
||||
" AIMessage(content='hello, how are you?', additional_kwargs={}, response_metadata={})]"
|
||||
]
|
||||
},
|
||||
"execution_count": 3,
|
||||
"execution_count": 4,
|
||||
"metadata": {},
|
||||
"output_type": "execute_result"
|
||||
}
|
||||
@ -139,7 +140,7 @@
|
||||
"name": "python",
|
||||
"nbconvert_exporter": "python",
|
||||
"pygments_lexer": "ipython3",
|
||||
"version": "3.10.12"
|
||||
"version": "3.12.8"
|
||||
}
|
||||
},
|
||||
"nbformat": 4,
|
||||
|
@ -46,6 +46,14 @@ See [installation instructions and a usage example](/docs/integrations/chat/tong
|
||||
from langchain_community.chat_models.tongyi import ChatTongyi
|
||||
```
|
||||
|
||||
### Qwen QwQ Chat
|
||||
|
||||
See [installation instructions and a usage example](/docs/integrations/chat/qwq)
|
||||
|
||||
```python
|
||||
from langchain_qwq import ChatQwQ
|
||||
```
|
||||
|
||||
## Document Loaders
|
||||
|
||||
### Alibaba Cloud MaxCompute
|
||||
|
@ -35,9 +35,18 @@ from langchain_aws import ChatBedrock
|
||||
```
|
||||
|
||||
### Bedrock Converse
|
||||
AWS has recently released the Bedrock Converse API which provides a unified conversational interface for Bedrock models. This API does not yet support custom models. You can see a list of all [models that are supported here](https://docs.aws.amazon.com/bedrock/latest/userguide/conversation-inference.html). To improve reliability the ChatBedrock integration will switch to using the Bedrock Converse API as soon as it has feature parity with the existing Bedrock API. Until then a separate [ChatBedrockConverse](https://python.langchain.com/api_reference/aws/chat_models/langchain_aws.chat_models.bedrock_converse.ChatBedrockConverse.html) integration has been released.
|
||||
AWS Bedrock maintains a [Converse API](https://docs.aws.amazon.com/bedrock/latest/APIReference/API_runtime_Converse.html)
|
||||
that provides a unified conversational interface for Bedrock models. This API does not
|
||||
yet support custom models. You can see a list of all
|
||||
[models that are supported here](https://docs.aws.amazon.com/bedrock/latest/userguide/conversation-inference.html).
|
||||
|
||||
We recommend using `ChatBedrockConverse` for users who do not need to use custom models. See the [docs](/docs/integrations/chat/bedrock/#bedrock-converse-api) and [API reference](https://python.langchain.com/api_reference/aws/chat_models/langchain_aws.chat_models.bedrock_converse.ChatBedrockConverse.html) for more detail.
|
||||
:::info
|
||||
|
||||
We recommend the Converse API for users who do not need to use custom models. It can be accessed using [ChatBedrockConverse](https://python.langchain.com/api_reference/aws/chat_models/langchain_aws.chat_models.bedrock_converse.ChatBedrockConverse.html).
|
||||
|
||||
:::
|
||||
|
||||
See a [usage example](/docs/integrations/chat/bedrock).
|
||||
|
||||
```python
|
||||
from langchain_aws import ChatBedrockConverse
|
||||
|
@ -8,18 +8,34 @@
|
||||
> learning models, on the `Cloudflare` network, from your code via REST API.
|
||||
|
||||
|
||||
## ChatModels
|
||||
|
||||
See [installation instructions and usage example](/docs/integrations/chat/cloudflare_workersai).
|
||||
|
||||
```python
|
||||
from langchain_cloudflare import ChatCloudflareWorkersAI
|
||||
```
|
||||
|
||||
## VectorStore
|
||||
|
||||
See [installation instructions and usage example](/docs/integrations/vectorstores/cloudflare_vectorize).
|
||||
|
||||
```python
|
||||
from langchain_cloudflare import CloudflareVectorize
|
||||
```
|
||||
|
||||
## Embeddings
|
||||
|
||||
See [installation instructions and usage example](/docs/integrations/text_embedding/cloudflare_workersai).
|
||||
|
||||
```python
|
||||
from langchain_cloudflare import CloudflareWorkersAIEmbeddings
|
||||
```
|
||||
|
||||
## LLMs
|
||||
|
||||
See [installation instructions and usage example](/docs/integrations/llms/cloudflare_workersai).
|
||||
|
||||
```python
|
||||
from langchain_community.llms.cloudflare_workersai import CloudflareWorkersAI
|
||||
```
|
||||
|
||||
## Embedding models
|
||||
|
||||
See [installation instructions and usage example](/docs/integrations/text_embedding/cloudflare_workersai).
|
||||
|
||||
```python
|
||||
from langchain_community.embeddings.cloudflare_workersai import CloudflareWorkersAIEmbeddings
|
||||
```
|
||||
```
|
@ -17,7 +17,7 @@ pip install langchain-couchbase
|
||||
See a [usage example](/docs/integrations/vectorstores/couchbase).
|
||||
|
||||
```python
|
||||
from langchain_couchbase import CouchbaseVectorStore
|
||||
from langchain_couchbase import CouchbaseSearchVectorStore
|
||||
```
|
||||
|
||||
## Document loader
|
||||
|
32
docs/docs/integrations/providers/galaxia.mdx
Normal file
32
docs/docs/integrations/providers/galaxia.mdx
Normal file
@ -0,0 +1,32 @@
|
||||
# Smabbler
|
||||
> Smabbler’s graph-powered platform boosts AI development by transforming data into a structured knowledge foundation.
|
||||
|
||||
# Galaxia
|
||||
|
||||
> Galaxia Knowledge Base is an integrated knowledge base and retrieval mechanism for RAG. In contrast to standard solution, it is based on Knowledge Graphs built using symbolic NLP and Knowledge Representation solutions. Provided texts are analysed and transformed into Graphs containing text, language and semantic information. This rich structure allows for retrieval that is based on semantic information, not on vector similarity/distance.
|
||||
|
||||
Implementing RAG using Galaxia involves first uploading your files to [Galaxia](https://beta.cloud.smabbler.com/home), analyzing them there and then building a model (knowledge graph). When the model is built, you can use `GalaxiaRetriever` to connect to the API and start retrieving.
|
||||
|
||||
More information: [docs](https://smabbler.gitbook.io/smabbler)
|
||||
|
||||
## Installation
|
||||
```
|
||||
pip install langchain-galaxia-retriever
|
||||
```
|
||||
|
||||
## Usage
|
||||
|
||||
```
|
||||
from langchain_galaxia_retriever.retriever import GalaxiaRetriever
|
||||
|
||||
gr = GalaxiaRetriever(
|
||||
api_url="beta.api.smabbler.com",
|
||||
api_key="<key>",
|
||||
knowledge_base_id="<knowledge_base_id>",
|
||||
n_retries=10,
|
||||
wait_time=5,
|
||||
)
|
||||
|
||||
result = gr.invoke('<test question>')
|
||||
print(result)
|
||||
|
File diff suppressed because it is too large
Load Diff
@ -25,6 +25,114 @@ And you should configure credentials by setting the following environment variab
|
||||
|
||||
Make sure to get your API Key from https://app.hyperbrowser.ai/
|
||||
|
||||
## Available Tools
|
||||
|
||||
Hyperbrowser provides two main categories of tools that are particularly useful for:
|
||||
- Web scraping and data extraction from complex websites
|
||||
- Automating repetitive web tasks
|
||||
- Interacting with web applications that require authentication
|
||||
- Performing research across multiple websites
|
||||
- Testing web applications
|
||||
|
||||
### Browser Agent Tools
|
||||
|
||||
Hyperbrowser provides a number of Browser Agents tools. Currently we supported
|
||||
- Claude Computer Use
|
||||
- OpenAI CUA
|
||||
- Browser Use
|
||||
|
||||
You can see more details [here](/docs/integrations/tools/hyperbrowser_browser_agent_tools)
|
||||
|
||||
#### Browser Use Tool
|
||||
A general-purpose browser automation tool that can handle various web tasks through natural language instructions.
|
||||
|
||||
```python
|
||||
from langchain_hyperbrowser import HyperbrowserBrowserUseTool
|
||||
|
||||
tool = HyperbrowserBrowserUseTool()
|
||||
result = tool.run({
|
||||
"task": "Go to npmjs.com, find the React package, and tell me when it was last updated"
|
||||
})
|
||||
print(result)
|
||||
```
|
||||
|
||||
#### OpenAI CUA Tool
|
||||
Leverages OpenAI's Computer Use Agent capabilities for advanced web interactions and information gathering.
|
||||
|
||||
```python
|
||||
from langchain_hyperbrowser import HyperbrowserOpenAICUATool
|
||||
|
||||
tool = HyperbrowserOpenAICUATool()
|
||||
result = tool.run({
|
||||
"task": "Go to Hacker News and summarize the top 5 posts right now"
|
||||
})
|
||||
print(result)
|
||||
```
|
||||
|
||||
#### Claude Computer Use Tool
|
||||
Utilizes Anthropic's Claude for sophisticated web browsing and information processing tasks.
|
||||
|
||||
```python
|
||||
from langchain_hyperbrowser import HyperbrowserClaudeComputerUseTool
|
||||
|
||||
tool = HyperbrowserClaudeComputerUseTool()
|
||||
result = tool.run({
|
||||
"task": "Go to GitHub's trending repositories page, and list the top 3 posts there right now"
|
||||
})
|
||||
print(result)
|
||||
```
|
||||
|
||||
### Web Scraping Tools
|
||||
|
||||
Here is a brief description of the Web Scraping Tools available with Hyperbrowser. You can see more details [here](/docs/integrations/tools/hyperbrowser_web_scraping_tools)
|
||||
|
||||
#### Scrape Tool
|
||||
The Scrape Tool allows you to extract content from a single webpage in markdown, HTML, or link format.
|
||||
|
||||
```python
|
||||
from langchain_hyperbrowser import HyperbrowserScrapeTool
|
||||
|
||||
tool = HyperbrowserScrapeTool()
|
||||
result = tool.run({
|
||||
"url": "https://example.com",
|
||||
"scrape_options": {"formats": ["markdown"]}
|
||||
})
|
||||
print(result)
|
||||
```
|
||||
|
||||
#### Crawl Tool
|
||||
The Crawl Tool enables you to traverse entire websites, starting from a given URL, with configurable page limits.
|
||||
|
||||
```python
|
||||
from langchain_hyperbrowser import HyperbrowserCrawlTool
|
||||
|
||||
tool = HyperbrowserCrawlTool()
|
||||
result = tool.run({
|
||||
"url": "https://example.com",
|
||||
"max_pages": 2,
|
||||
"scrape_options": {"formats": ["markdown"]}
|
||||
})
|
||||
print(result)
|
||||
```
|
||||
|
||||
#### Extract Tool
|
||||
The Extract Tool uses AI to pull structured data from web pages based on predefined schemas, making it perfect for data extraction tasks.
|
||||
|
||||
```python
|
||||
from langchain_hyperbrowser import HyperbrowserExtractTool
|
||||
from pydantic import BaseModel
|
||||
|
||||
class SimpleExtractionModel(BaseModel):
|
||||
title: str
|
||||
|
||||
tool = HyperbrowserExtractTool()
|
||||
result = tool.run({
|
||||
"url": "https://example.com",
|
||||
"schema": SimpleExtractionModel
|
||||
})
|
||||
print(result)
|
||||
```
|
||||
|
||||
## Document Loader
|
||||
|
||||
The `HyperbrowserLoader` class in `langchain-hyperbrowser` can easily be used to load content from any single page or multiple pages as well as crawl an entire site.
|
||||
@ -39,7 +147,7 @@ docs = loader.load()
|
||||
print(docs[0])
|
||||
```
|
||||
|
||||
## Advanced Usage
|
||||
### Advanced Usage
|
||||
|
||||
You can specify the operation to be performed by the loader. The default operation is `scrape`. For `scrape`, you can provide a single URL or a list of URLs to be scraped. For `crawl`, you can only provide a single URL. The `crawl` operation will crawl the provided page and subpages and return a document for each page.
|
||||
|
||||
|
165
docs/docs/integrations/providers/langfuse.mdx
Normal file
165
docs/docs/integrations/providers/langfuse.mdx
Normal file
@ -0,0 +1,165 @@
|
||||
# Langfuse 🪢
|
||||
|
||||
> **What is Langfuse?** [Langfuse](https://langfuse.com) is an open source LLM engineering platform that helps teams trace API calls, monitor performance, and debug issues in their AI applications.
|
||||
|
||||
## Tracing LangChain
|
||||
|
||||
[Langfuse Tracing](https://langfuse.com/docs/tracing) integrates with Langchain using Langchain Callbacks ([Python](https://python.langchain.com/docs/how_to/#callbacks), [JS](https://js.langchain.com/docs/how_to/#callbacks)). Thereby, the Langfuse SDK automatically creates a nested trace for every run of your Langchain applications. This allows you to log, analyze and debug your LangChain application.
|
||||
|
||||
You can configure the integration via (1) constructor arguments or (2) environment variables. Get your Langfuse credentials by signing up at [cloud.langfuse.com](https://cloud.langfuse.com) or [self-hosting Langfuse](https://langfuse.com/self-hosting).
|
||||
|
||||
### Constructor arguments
|
||||
|
||||
```python
|
||||
pip install langfuse
|
||||
```
|
||||
|
||||
```python
|
||||
# Initialize Langfuse handler
|
||||
from langfuse.callback import CallbackHandler
|
||||
langfuse_handler = CallbackHandler(
|
||||
secret_key="sk-lf-...",
|
||||
public_key="pk-lf-...",
|
||||
host="https://cloud.langfuse.com", # 🇪🇺 EU region
|
||||
# host="https://us.cloud.langfuse.com", # 🇺🇸 US region
|
||||
)
|
||||
|
||||
# Your Langchain code
|
||||
|
||||
# Add Langfuse handler as callback (classic and LCEL)
|
||||
chain.invoke({"input": "<user_input>"}, config={"callbacks": [langfuse_handler]})
|
||||
```
|
||||
|
||||
### Environment variables
|
||||
|
||||
```bash filename=".env"
|
||||
LANGFUSE_SECRET_KEY="sk-lf-..."
|
||||
LANGFUSE_PUBLIC_KEY="pk-lf-..."
|
||||
# 🇪🇺 EU region
|
||||
LANGFUSE_HOST="https://cloud.langfuse.com"
|
||||
# 🇺🇸 US region
|
||||
# LANGFUSE_HOST="https://us.cloud.langfuse.com"
|
||||
```
|
||||
|
||||
```python
|
||||
# Initialize Langfuse handler
|
||||
from langfuse.callback import CallbackHandler
|
||||
langfuse_handler = CallbackHandler()
|
||||
|
||||
# Your Langchain code
|
||||
|
||||
# Add Langfuse handler as callback (classic and LCEL)
|
||||
chain.invoke({"input": "<user_input>"}, config={"callbacks": [langfuse_handler]})
|
||||
```
|
||||
|
||||
To see how to use this integration together with other Langfuse features, check out [this end-to-end example](https://langfuse.com/docs/integrations/langchain/example-python).
|
||||
|
||||
## Tracing LangGraph
|
||||
|
||||
This part demonstrates how [Langfuse](https://langfuse.com/docs) helps to debug, analyze, and iterate on your LangGraph application using the [LangChain integration](https://langfuse.com/docs/integrations/langchain/tracing).
|
||||
|
||||
### Initialize Langfuse
|
||||
|
||||
**Note:** You need to run at least Python 3.11 ([GitHub Issue](https://github.com/langfuse/langfuse/issues/1926)).
|
||||
|
||||
Initialize the Langfuse client with your [API keys](https://langfuse.com/faq/all/where-are-langfuse-api-keys) from the project settings in the Langfuse UI and add them to your environment.
|
||||
|
||||
|
||||
```python
|
||||
%pip install langfuse
|
||||
%pip install langchain langgraph langchain_openai langchain_community
|
||||
```
|
||||
|
||||
|
||||
```python
|
||||
import os
|
||||
|
||||
# get keys for your project from https://cloud.langfuse.com
|
||||
os.environ["LANGFUSE_PUBLIC_KEY"] = "pk-lf-***"
|
||||
os.environ["LANGFUSE_SECRET_KEY"] = "sk-lf-***"
|
||||
os.environ["LANGFUSE_HOST"] = "https://cloud.langfuse.com" # for EU data region
|
||||
# os.environ["LANGFUSE_HOST"] = "https://us.cloud.langfuse.com" # for US data region
|
||||
|
||||
# your openai key
|
||||
os.environ["OPENAI_API_KEY"] = "***"
|
||||
```
|
||||
|
||||
### Simple chat app with LangGraph
|
||||
|
||||
**What we will do in this section:**
|
||||
|
||||
* Build a support chatbot in LangGraph that can answer common questions
|
||||
* Tracing the chatbot's input and output using Langfuse
|
||||
|
||||
We will start with a basic chatbot and build a more advanced multi agent setup in the next section, introducing key LangGraph concepts along the way.
|
||||
|
||||
#### Create Agent
|
||||
|
||||
Start by creating a `StateGraph`. A `StateGraph` object defines our chatbot's structure as a state machine. We will add nodes to represent the LLM and functions the chatbot can call, and edges to specify how the bot transitions between these functions.
|
||||
|
||||
|
||||
```python
|
||||
from typing import Annotated
|
||||
|
||||
from langchain_openai import ChatOpenAI
|
||||
from langchain_core.messages import HumanMessage
|
||||
from typing_extensions import TypedDict
|
||||
|
||||
from langgraph.graph import StateGraph
|
||||
from langgraph.graph.message import add_messages
|
||||
|
||||
class State(TypedDict):
|
||||
# Messages have the type "list". The `add_messages` function in the annotation defines how this state key should be updated
|
||||
# (in this case, it appends messages to the list, rather than overwriting them)
|
||||
messages: Annotated[list, add_messages]
|
||||
|
||||
graph_builder = StateGraph(State)
|
||||
|
||||
llm = ChatOpenAI(model = "gpt-4o", temperature = 0.2)
|
||||
|
||||
# The chatbot node function takes the current State as input and returns an updated messages list. This is the basic pattern for all LangGraph node functions.
|
||||
def chatbot(state: State):
|
||||
return {"messages": [llm.invoke(state["messages"])]}
|
||||
|
||||
# Add a "chatbot" node. Nodes represent units of work. They are typically regular python functions.
|
||||
graph_builder.add_node("chatbot", chatbot)
|
||||
|
||||
# Add an entry point. This tells our graph where to start its work each time we run it.
|
||||
graph_builder.set_entry_point("chatbot")
|
||||
|
||||
# Set a finish point. This instructs the graph "any time this node is run, you can exit."
|
||||
graph_builder.set_finish_point("chatbot")
|
||||
|
||||
# To be able to run our graph, call "compile()" on the graph builder. This creates a "CompiledGraph" we can use invoke on our state.
|
||||
graph = graph_builder.compile()
|
||||
```
|
||||
|
||||
#### Add Langfuse as callback to the invocation
|
||||
|
||||
Now, we will add then [Langfuse callback handler for LangChain](https://langfuse.com/docs/integrations/langchain/tracing) to trace the steps of our application: `config={"callbacks": [langfuse_handler]}`
|
||||
|
||||
|
||||
```python
|
||||
from langfuse.callback import CallbackHandler
|
||||
|
||||
# Initialize Langfuse CallbackHandler for Langchain (tracing)
|
||||
langfuse_handler = CallbackHandler()
|
||||
|
||||
for s in graph.stream({"messages": [HumanMessage(content = "What is Langfuse?")]},
|
||||
config={"callbacks": [langfuse_handler]}):
|
||||
print(s)
|
||||
```
|
||||
|
||||
```
|
||||
{'chatbot': {'messages': [AIMessage(content='Langfuse is a tool designed to help developers monitor and observe the performance of their Large Language Model (LLM) applications. It provides detailed insights into how these applications are functioning, allowing for better debugging, optimization, and overall management. Langfuse offers features such as tracking key metrics, visualizing data, and identifying potential issues in real-time, making it easier for developers to maintain and improve their LLM-based solutions.', response_metadata={'token_usage': {'completion_tokens': 86, 'prompt_tokens': 13, 'total_tokens': 99}, 'model_name': 'gpt-4o-2024-05-13', 'system_fingerprint': 'fp_400f27fa1f', 'finish_reason': 'stop', 'logprobs': None}, id='run-9a0c97cb-ccfe-463e-902c-5a5900b796b4-0', usage_metadata={'input_tokens': 13, 'output_tokens': 86, 'total_tokens': 99})]}}
|
||||
```
|
||||
|
||||
|
||||
#### View traces in Langfuse
|
||||
|
||||
Example trace in Langfuse: https://cloud.langfuse.com/project/cloramnkj0002jz088vzn1ja4/traces/d109e148-d188-4d6e-823f-aac0864afbab
|
||||
|
||||
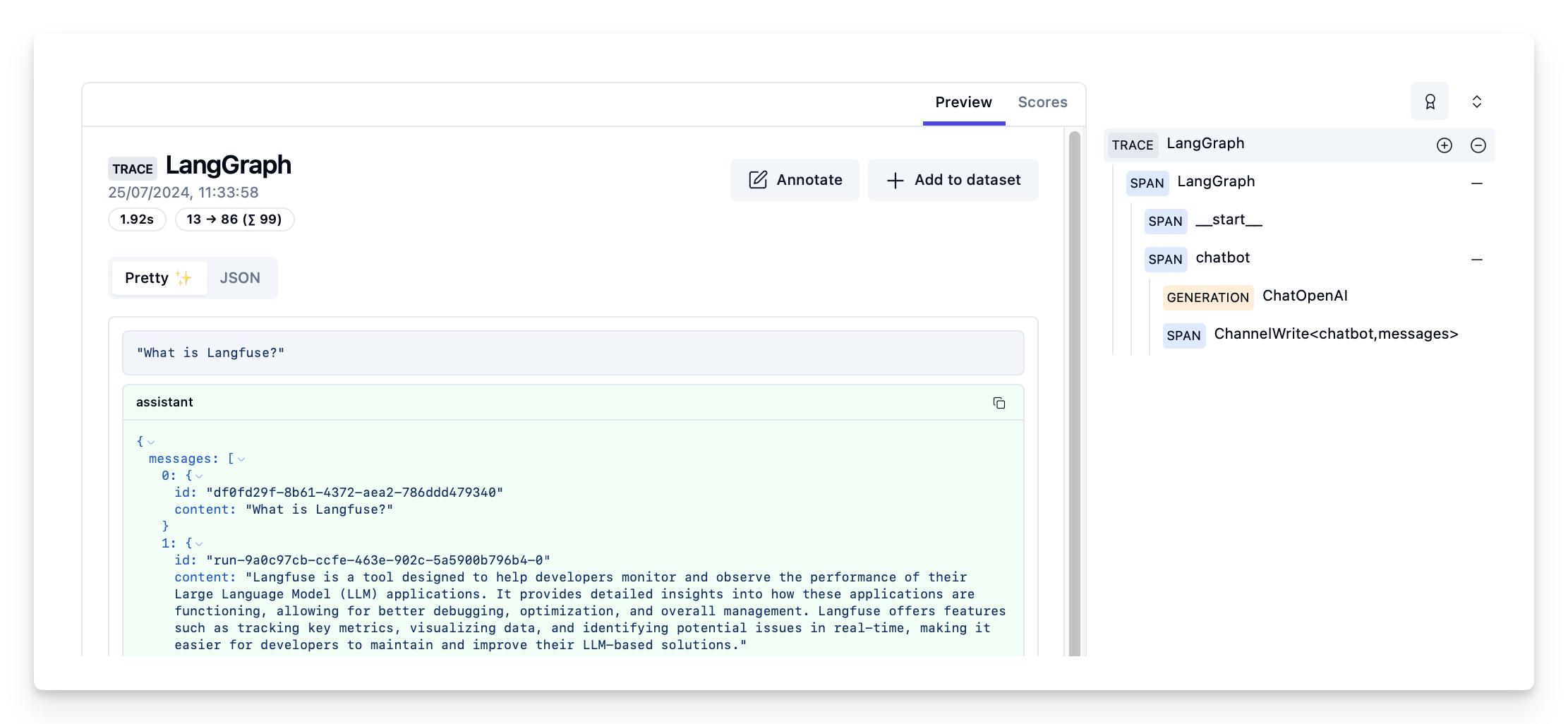
|
||||
|
||||
- Check out the [full notebook](https://langfuse.com/docs/integrations/langchain/example-python-langgraph) to see more examples.
|
||||
- To learn how to evaluate the performance of your LangGraph application, check out the [LangGraph evaluation guide](https://langfuse.com/docs/integrations/langchain/example-langgraph-agents).
|
21
docs/docs/integrations/providers/litellm.mdx
Normal file
21
docs/docs/integrations/providers/litellm.mdx
Normal file
@ -0,0 +1,21 @@
|
||||
# LiteLLM
|
||||
|
||||
>[LiteLLM](https://github.com/BerriAI/litellm) is a library that simplifies calling Anthropic, Azure, Huggingface, Replicate, etc.
|
||||
|
||||
## Installation and setup
|
||||
|
||||
```bash
|
||||
pip install langchain-litellm
|
||||
```
|
||||
|
||||
## Chat Models
|
||||
```python
|
||||
from langchain_litellm import ChatLiteLLM
|
||||
```
|
||||
```python
|
||||
from langchain_litellm import ChatLiteLLMRouter
|
||||
```
|
||||
See more detail in the guide [here](/docs/integrations/chat/litellm).
|
||||
|
||||
## API reference
|
||||
For detailed documentation of all `ChatLiteLLM` and `ChatLiteLLMRouter` features and configurations head to the API reference: https://github.com/Akshay-Dongare/langchain-litellm
|
@ -1,37 +0,0 @@
|
||||
# LiteLLM
|
||||
|
||||
>[LiteLLM](https://docs.litellm.ai/docs/) is a library that simplifies calling Anthropic,
|
||||
> Azure, Huggingface, Replicate, etc. LLMs in a unified way.
|
||||
>
|
||||
>You can use `LiteLLM` through either:
|
||||
>
|
||||
>* [LiteLLM Proxy Server](https://docs.litellm.ai/docs/#openai-proxy) - Server to call 100+ LLMs, load balance, cost tracking across projects
|
||||
>* [LiteLLM python SDK](https://docs.litellm.ai/docs/#basic-usage) - Python Client to call 100+ LLMs, load balance, cost tracking
|
||||
|
||||
## Installation and setup
|
||||
|
||||
Install the `litellm` python package.
|
||||
|
||||
```bash
|
||||
pip install litellm
|
||||
```
|
||||
|
||||
## Chat models
|
||||
|
||||
### ChatLiteLLM
|
||||
|
||||
See a [usage example](/docs/integrations/chat/litellm).
|
||||
|
||||
```python
|
||||
from langchain_community.chat_models import ChatLiteLLM
|
||||
```
|
||||
|
||||
### ChatLiteLLMRouter
|
||||
|
||||
You also can use the `ChatLiteLLMRouter` to route requests to different LLMs or LLM providers.
|
||||
|
||||
See a [usage example](/docs/integrations/chat/litellm_router).
|
||||
|
||||
```python
|
||||
from langchain_community.chat_models import ChatLiteLLMRouter
|
||||
```
|
41
docs/docs/integrations/providers/mariadb.mdx
Normal file
41
docs/docs/integrations/providers/mariadb.mdx
Normal file
@ -0,0 +1,41 @@
|
||||
# MariaDB
|
||||
|
||||
This page covers how to use the [MariaDB](https://github.com/mariadb/) ecosystem within LangChain.
|
||||
It is broken into two parts: installation and setup, and then references to specific PGVector wrappers.
|
||||
|
||||
## Installation
|
||||
- Install c/c connector
|
||||
|
||||
on Debian, Ubuntu
|
||||
```bash
|
||||
sudo apt install libmariadb3 libmariadb-dev
|
||||
```
|
||||
|
||||
on CentOS, RHEL, Rocky Linux
|
||||
```bash
|
||||
sudo yum install MariaDB-shared MariaDB-devel
|
||||
```
|
||||
|
||||
- Install the Python connector package with `pip install mariadb`
|
||||
|
||||
|
||||
## Setup
|
||||
1. The first step is to have a MariaDB 11.7.1 or later installed.
|
||||
|
||||
The docker image is the easiest way to get started.
|
||||
|
||||
## Wrappers
|
||||
|
||||
### VectorStore
|
||||
|
||||
There exists a wrapper around MariaDB vector databases, allowing you to use it as a vectorstore,
|
||||
whether for semantic search or example selection.
|
||||
|
||||
To import this vectorstore:
|
||||
```python
|
||||
from langchain_mariadb import MariaDBStore
|
||||
```
|
||||
|
||||
### Usage
|
||||
|
||||
For a more detailed walkthrough of the MariaDB wrapper, see [this notebook](/docs/integrations/vectorstores/mariadb)
|
@ -10,19 +10,23 @@ Please refer to [NCP User Guide](https://guide.ncloud-docs.com/docs/clovastudio-
|
||||
|
||||
## Installation and Setup
|
||||
|
||||
- Get a CLOVA Studio API Key by [issuing it](https://api.ncloud-docs.com/docs/ai-naver-clovastudio-summary#API%ED%82%A4) and set it as an environment variable (`NCP_CLOVASTUDIO_API_KEY`).
|
||||
- If you are using a legacy API Key (that doesn't start with `nv-*` prefix), you might need to get an additional API Key by [creating your app](https://guide.ncloud-docs.com/docs/en/clovastudio-playground01#create-test-app) and set it as `NCP_APIGW_API_KEY`.
|
||||
- Get a CLOVA Studio API Key by [issuing it](https://api.ncloud-docs.com/docs/ai-naver-clovastudio-summary#API%ED%82%A4) and set it as an environment variable (`CLOVASTUDIO_API_KEY`).
|
||||
|
||||
|
||||
Naver integrations live in two packages:
|
||||
|
||||
- `langchain-naver-community`: a dedicated integration package for Naver. It is a community-maintained package and is not officially maintained by Naver or LangChain.
|
||||
- `langchain-community`: a collection of [third-party integrations](https://python.langchain.com/docs/concepts/architecture/#langchain-community),
|
||||
including Naver. **New features should be implemented in the dedicated `langchain-naver-community` package**.
|
||||
- `langchain-naver`: a dedicated integration package for Naver.
|
||||
- `langchain-naver-community`: a community-maintained package and is not officially maintained by Naver or LangChain.
|
||||
|
||||
```bash
|
||||
pip install -U langchain-community langchain-naver-community
|
||||
pip install -U langchain-naver
|
||||
# pip install -U langchain-naver-community // Install to use Naver Search tool.
|
||||
```
|
||||
|
||||
> **(Note)** Naver integration via `langchain-community`, a collection of [third-party integrations](https://python.langchain.com/docs/concepts/architecture/#langchain-community), is outdated.
|
||||
> - **Use `langchain-naver` instead as new features should only be implemented via this package**.
|
||||
> - If you are using `langchain-community` (outdated) and got a legacy API Key (that doesn't start with `nv-*` prefix), you should set it as `NCP_CLOVASTUDIO_API_KEY`, and might need to get an additional API Gateway API Key by [creating your app](https://guide.ncloud-docs.com/docs/en/clovastudio-playground01#create-test-app) and set it as `NCP_APIGW_API_KEY`.
|
||||
|
||||
## Chat models
|
||||
|
||||
### ChatClovaX
|
||||
@ -30,7 +34,7 @@ pip install -U langchain-community langchain-naver-community
|
||||
See a [usage example](/docs/integrations/chat/naver).
|
||||
|
||||
```python
|
||||
from langchain_community.chat_models import ChatClovaX
|
||||
from langchain_naver import ChatClovaX
|
||||
```
|
||||
|
||||
## Embedding models
|
||||
@ -40,7 +44,7 @@ from langchain_community.chat_models import ChatClovaX
|
||||
See a [usage example](/docs/integrations/text_embedding/naver).
|
||||
|
||||
```python
|
||||
from langchain_community.embeddings import ClovaXEmbeddings
|
||||
from langchain_naver import ClovaXEmbeddings
|
||||
```
|
||||
|
||||
## Tools
|
||||
|
18
docs/docs/integrations/providers/oxylabs.mdx
Normal file
18
docs/docs/integrations/providers/oxylabs.mdx
Normal file
@ -0,0 +1,18 @@
|
||||
# Oxylabs
|
||||
|
||||
[Oxylabs](https://oxylabs.io/) is a market-leading web intelligence collection platform, driven by the highest business,
|
||||
ethics, and compliance standards, enabling companies worldwide to unlock data-driven insights.
|
||||
|
||||
[langchain-oxylabs](https://pypi.org/project/langchain-oxylabs/) implements
|
||||
tools enabling LLMs to interact with Oxylabs Web Scraper API.
|
||||
|
||||
|
||||
## Installation and Setup
|
||||
|
||||
```bash
|
||||
pip install langchain-oxylabs
|
||||
```
|
||||
|
||||
## Tools
|
||||
|
||||
See details on available tools [here](/docs/integrations/tools/oxylabs/).
|
@ -8,18 +8,18 @@
|
||||
|
||||
## Installation and Setup
|
||||
|
||||
Install a Python package:
|
||||
Install the Perplexity x LangChain integration package:
|
||||
|
||||
```bash
|
||||
pip install openai
|
||||
pip install langchain-perplexity
|
||||
````
|
||||
|
||||
Get your API key from [here](https://docs.perplexity.ai/docs/getting-started).
|
||||
|
||||
## Chat models
|
||||
|
||||
See a [usage example](/docs/integrations/chat/perplexity).
|
||||
See a variety of usage examples [here](/docs/integrations/chat/perplexity).
|
||||
|
||||
```python
|
||||
from langchain_community.chat_models import ChatPerplexity
|
||||
from langchain_perplexity import ChatPerplexity
|
||||
```
|
||||
|
@ -59,7 +59,7 @@ with the default values of "service-name = mymaster" and "db-number = 0" if not
|
||||
The service-name is the redis server monitoring group name as configured within the Sentinel.
|
||||
|
||||
The current url format limits the connection string to one sentinel host only (no list can be given) and
|
||||
booth Redis server and sentinel must have the same password set (if used).
|
||||
both Redis server and sentinel must have the same password set (if used).
|
||||
|
||||
#### Redis Cluster connection url
|
||||
|
||||
|
173
docs/docs/integrations/providers/runpod.ipynb
Normal file
173
docs/docs/integrations/providers/runpod.ipynb
Normal file
@ -0,0 +1,173 @@
|
||||
{
|
||||
"cells": [
|
||||
{
|
||||
"cell_type": "markdown",
|
||||
"metadata": {},
|
||||
"source": [
|
||||
"# Runpod\n",
|
||||
"\n",
|
||||
"[RunPod](https://www.runpod.io/) provides GPU cloud infrastructure, including Serverless endpoints optimized for deploying and scaling AI models.\n",
|
||||
"\n",
|
||||
"This guide covers how to use the `langchain-runpod` integration package to connect LangChain applications to models hosted on [RunPod Serverless](https://www.runpod.io/serverless-gpu).\n",
|
||||
"\n",
|
||||
"The integration offers interfaces for both standard Language Models (LLMs) and Chat Models."
|
||||
]
|
||||
},
|
||||
{
|
||||
"cell_type": "markdown",
|
||||
"metadata": {},
|
||||
"source": [
|
||||
"## Intstallation\n",
|
||||
"\n",
|
||||
"Install the dedicated partner package:"
|
||||
]
|
||||
},
|
||||
{
|
||||
"cell_type": "code",
|
||||
"execution_count": null,
|
||||
"metadata": {
|
||||
"vscode": {
|
||||
"languageId": "plaintext"
|
||||
}
|
||||
},
|
||||
"outputs": [],
|
||||
"source": [
|
||||
"%pip install -qU langchain-runpod"
|
||||
]
|
||||
},
|
||||
{
|
||||
"cell_type": "markdown",
|
||||
"metadata": {},
|
||||
"source": [
|
||||
"## Setup\n",
|
||||
"### 1. Deploy an Endpoint on RunPod\n",
|
||||
"- Navigate to your [RunPod Serverless Console](https://www.runpod.io/console/serverless/user/endpoints).\n",
|
||||
"- Create a \\\"New Endpoint\\\", selecting an appropriate GPU and template (e.g., vLLM, TGI, text-generation-webui) compatible with your model and the expected input/output format (see component guides or the package [README](https://github.com/runpod/langchain-runpod)).\n",
|
||||
"- Configure settings and deploy.\n",
|
||||
"- **Crucially, copy the Endpoint ID** after deployment."
|
||||
]
|
||||
},
|
||||
{
|
||||
"cell_type": "markdown",
|
||||
"metadata": {},
|
||||
"source": [
|
||||
"### 2. Set API Credentials\n",
|
||||
"The integration needs your RunPod API Key and the Endpoint ID. Set them as environment variables for secure access:"
|
||||
]
|
||||
},
|
||||
{
|
||||
"cell_type": "code",
|
||||
"execution_count": null,
|
||||
"metadata": {
|
||||
"vscode": {
|
||||
"languageId": "plaintext"
|
||||
}
|
||||
},
|
||||
"outputs": [],
|
||||
"source": [
|
||||
"import getpass\n",
|
||||
"import os\n",
|
||||
"\n",
|
||||
"os.environ[\"RUNPOD_API_KEY\"] = getpass.getpass(\"Enter your RunPod API Key: \")\n",
|
||||
"os.environ[\"RUNPOD_ENDPOINT_ID\"] = input(\"Enter your RunPod Endpoint ID: \")"
|
||||
]
|
||||
},
|
||||
{
|
||||
"cell_type": "markdown",
|
||||
"metadata": {},
|
||||
"source": [
|
||||
"*(Optional)* If using different endpoints for LLM and Chat models, you might need to set `RUNPOD_CHAT_ENDPOINT_ID` or pass the ID directly during initialization."
|
||||
]
|
||||
},
|
||||
{
|
||||
"cell_type": "markdown",
|
||||
"metadata": {},
|
||||
"source": [
|
||||
"## Components\n",
|
||||
"This package provides two main components:"
|
||||
]
|
||||
},
|
||||
{
|
||||
"cell_type": "markdown",
|
||||
"metadata": {},
|
||||
"source": [
|
||||
"### 1. LLM\n",
|
||||
"\n",
|
||||
"For interacting with standard text completion models.\n",
|
||||
"\n",
|
||||
"See the [RunPod LLM Integration Guide](/docs/integrations/llms/runpod) for detailed usage"
|
||||
]
|
||||
},
|
||||
{
|
||||
"cell_type": "code",
|
||||
"execution_count": null,
|
||||
"metadata": {
|
||||
"vscode": {
|
||||
"languageId": "plaintext"
|
||||
}
|
||||
},
|
||||
"outputs": [],
|
||||
"source": [
|
||||
"from langchain_runpod import RunPod\n",
|
||||
"\n",
|
||||
"# Example initialization (uses environment variables)\n",
|
||||
"llm = RunPod(model_kwargs={\"max_new_tokens\": 100}) # Add generation params here\n",
|
||||
"\n",
|
||||
"# Example Invocation\n",
|
||||
"try:\n",
|
||||
" response = llm.invoke(\"Write a short poem about the cloud.\")\n",
|
||||
" print(response)\n",
|
||||
"except Exception as e:\n",
|
||||
" print(\n",
|
||||
" f\"Error invoking LLM: {e}. Ensure endpoint ID and API key are correct and endpoint is active.\"\n",
|
||||
" )"
|
||||
]
|
||||
},
|
||||
{
|
||||
"cell_type": "markdown",
|
||||
"metadata": {},
|
||||
"source": [
|
||||
"### 2. Chat Model\n",
|
||||
"\n",
|
||||
"For interacting with conversational models.\n",
|
||||
"\n",
|
||||
"See the [RunPod Chat Model Integration Guide](/docs/integrations/chat/runpod) for detailed usage and feature support."
|
||||
]
|
||||
},
|
||||
{
|
||||
"cell_type": "code",
|
||||
"execution_count": null,
|
||||
"metadata": {
|
||||
"vscode": {
|
||||
"languageId": "plaintext"
|
||||
}
|
||||
},
|
||||
"outputs": [],
|
||||
"source": [
|
||||
"from langchain_core.messages import HumanMessage\n",
|
||||
"from langchain_runpod import ChatRunPod\n",
|
||||
"\n",
|
||||
"# Example initialization (uses environment variables)\n",
|
||||
"chat = ChatRunPod(model_kwargs={\"temperature\": 0.8}) # Add generation params here\n",
|
||||
"\n",
|
||||
"# Example Invocation\n",
|
||||
"try:\n",
|
||||
" response = chat.invoke(\n",
|
||||
" [HumanMessage(content=\"Explain RunPod Serverless in one sentence.\")]\n",
|
||||
" )\n",
|
||||
" print(response.content)\n",
|
||||
"except Exception as e:\n",
|
||||
" print(\n",
|
||||
" f\"Error invoking Chat Model: {e}. Ensure endpoint ID and API key are correct and endpoint is active.\"\n",
|
||||
" )"
|
||||
]
|
||||
}
|
||||
],
|
||||
"metadata": {
|
||||
"language_info": {
|
||||
"name": "python"
|
||||
}
|
||||
},
|
||||
"nbformat": 4,
|
||||
"nbformat_minor": 2
|
||||
}
|
Some files were not shown because too many files have changed in this diff Show More
Loading…
Reference in New Issue
Block a user